Gaussian Copula Embeddings
NeurIPS 2022(2022)
摘要
Learning latent vector representations via embedding models has been shown promising in machine learning. However, most of the embedding models are still limited to a single type of observation data. We propose a Gaussian copula embedding model to learn latent vector representations of items in a heterogeneous data setting. The proposed model can effectively incorporate different types of observed data and, at the same time, yield robust embeddings. We demonstrate the proposed model can effectively learn in many different scenarios, outperforming competing models in modeling quality and task performance.
更多查看译文
关键词
representation learning,gaussian copula,heterogeneous data,vectorial embedding,probabilistic models
AI 理解论文
溯源树
样例
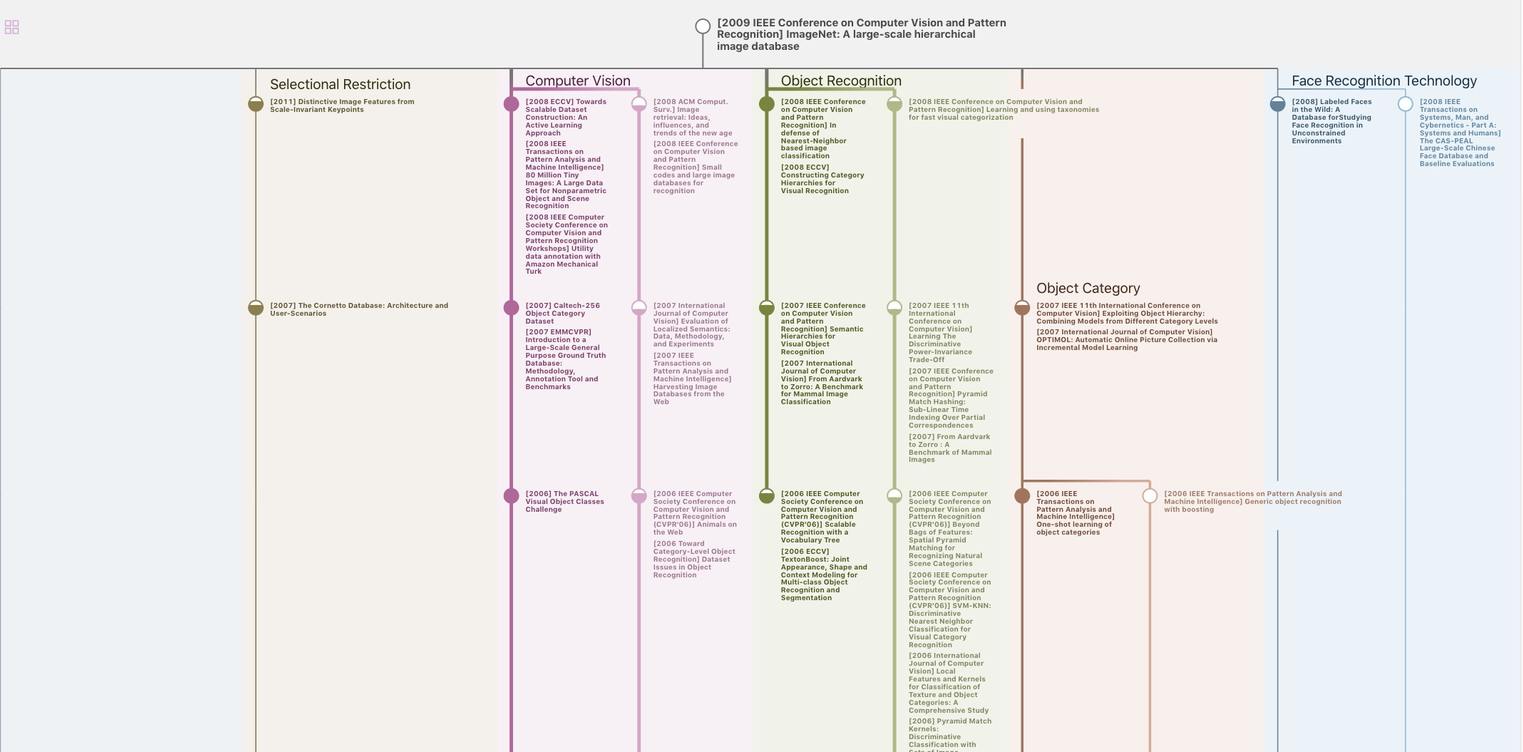
生成溯源树,研究论文发展脉络
Chat Paper
正在生成论文摘要