Effectiveness of Vision Transformer for Fast and Accurate Single-Stage Pedestrian Detection
NeurIPS 2022(2022)
摘要
Vision transformers have demonstrated remarkable performance on a variety of computer vision tasks. In this paper, we illustrate the effectiveness of the deformable vision transformer for single-stage pedestrian detection and propose a spatial and multi-scale feature enhancement module, which aims to achieve the optimal balance between speed and accuracy. Performance improvement with the use of vision transformers on various commonly used single-stage structures is demonstrated. The design of the proposed architecture is investigated in depth. Comprehensive comparisons with state-of-the-art single- and two-stage detectors on various pedestrian datasets are performed. The proposed detector achieves leading performance on Caltech and Citypersons datasets among single- and two-stage methods using fewer parameters than the baseline. The log-average miss rates for Reasonable and Heavy are decreased to 2.6% and 28.0% on the Caltech test set, and 10.9% and 38.6% on the Citypersons validation set respectively. The proposed method outperforms SOTA two-stage detectors in the Heavy subset on the Citypersons validation set with considerably faster inference speed.
更多查看译文
AI 理解论文
溯源树
样例
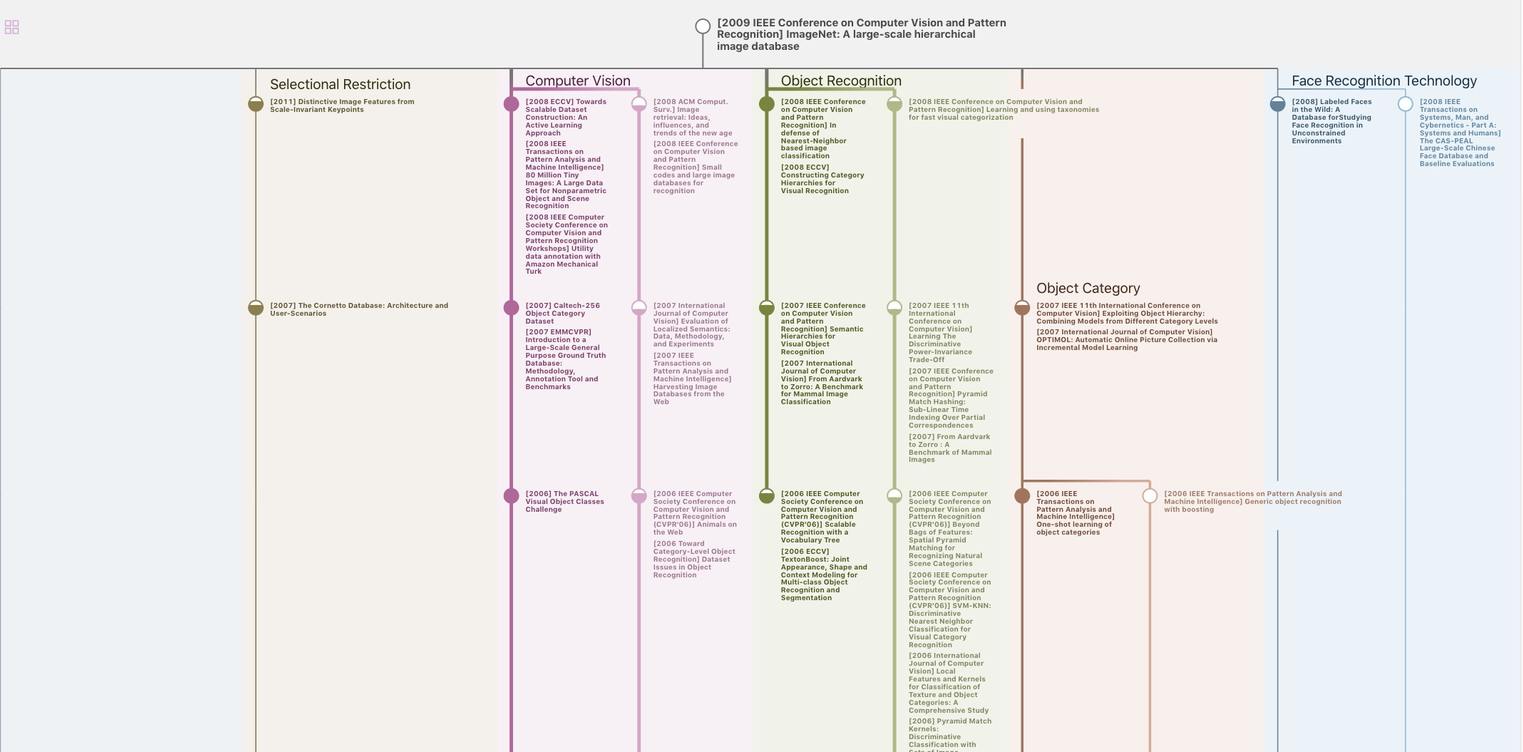
生成溯源树,研究论文发展脉络
Chat Paper
正在生成论文摘要