Concept Embedding Models
NeurIPS 2022(2022)
Abstract
Deploying AI-powered systems requires trustworthy models supporting effective human interactions, going beyond raw prediction accuracy. Concept bottleneck models promote trustworthiness by conditioning classification tasks on an intermediate level of human-like concepts. This enables human interventions which can correct mispredicted concepts to improve the model's performance. However, existing concept bottleneck models are unable to find optimal compromises between high task accuracy, robust concept-based explanations, and effective interventions on concepts---particularly in real-world conditions where complete and accurate concept supervisions are scarce. To address this, we propose Concept Embedding Models, a novel family of concept bottleneck models which goes beyond the current accuracy-vs-interpretability trade-off by learning interpretable high-dimensional concept representations. Our experiments demonstrate that Concept Embedding Models (1) attain better or competitive task accuracy w.r.t. standard neural models without concepts, (2) provide concept representations capturing meaningful semantics including and beyond their ground truth labels, (3) support test-time concept interventions whose effect in test accuracy surpasses that in standard concept bottleneck models, and (4) scale to real-world conditions where complete concept supervisions are scarce.
MoreTranslated text
Key words
Explainable Artificial Intelligence,Concept Bottleneck Models,Concept-based Explainability,Interpretability,XAI
AI Read Science
Must-Reading Tree
Example
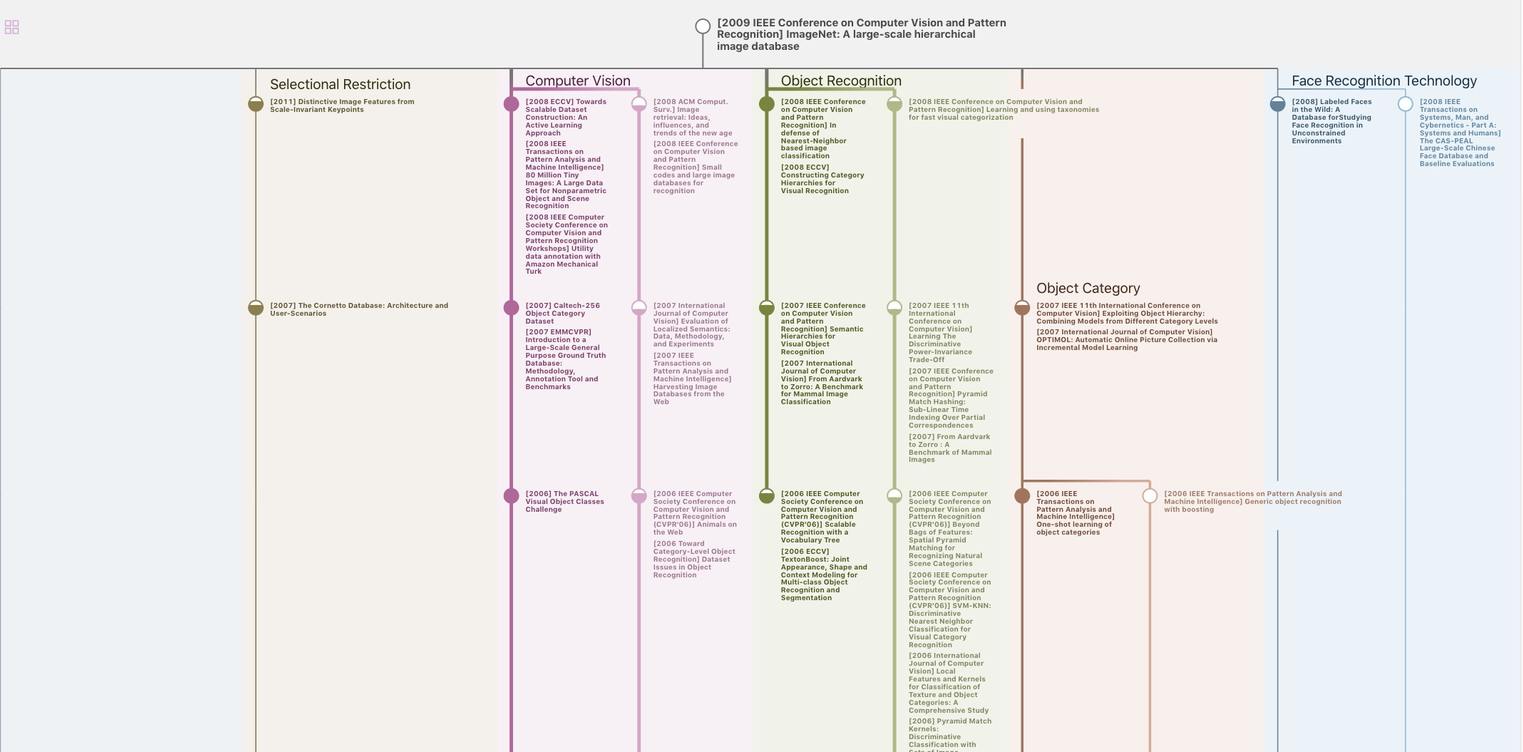
Generate MRT to find the research sequence of this paper
Chat Paper
Summary is being generated by the instructions you defined