The Role of Baselines in Policy Gradient Optimization
NeurIPS 2022(2022)
摘要
We study the effect of baselines in on-policy stochastic policy gradient optimization, and close the gap between the theory and practice of policy optimization methods. Our first contribution is to show that the \emph{state value} baseline allows on-policy stochastic \emph{natural} policy gradient (NPG) to converge to a globally optimal policy at an $O(1/t)$ rate, which was not previously known. The analysis relies on two novel findings: the expected progress of the NPG update satisfies a stochastic version of the non-uniform \L{}ojasiewicz (N\L{}) inequality, and with probability 1 the state value baseline prevents the optimal action's probability from vanishing, thus ensuring sufficient exploration. Importantly, these results provide a new understanding of the role of baselines in stochastic policy gradient: by showing that the variance of natural policy gradient estimates remains unbounded with or without a baseline, we find that variance reduction \emph{cannot} explain their utility in this setting. Instead, the analysis reveals that the primary effect of the value baseline is to \textbf{reduce the aggressiveness of the updates} rather than their variance. That is, we demonstrate that a finite variance is \emph{not necessary} for almost sure convergence of stochastic NPG, while controlling update aggressiveness is both necessary and sufficient. Additional experimental results verify these theoretical findings.
更多查看译文
关键词
reinforcement learning,policy optimization,policy gradient,global convergence
AI 理解论文
溯源树
样例
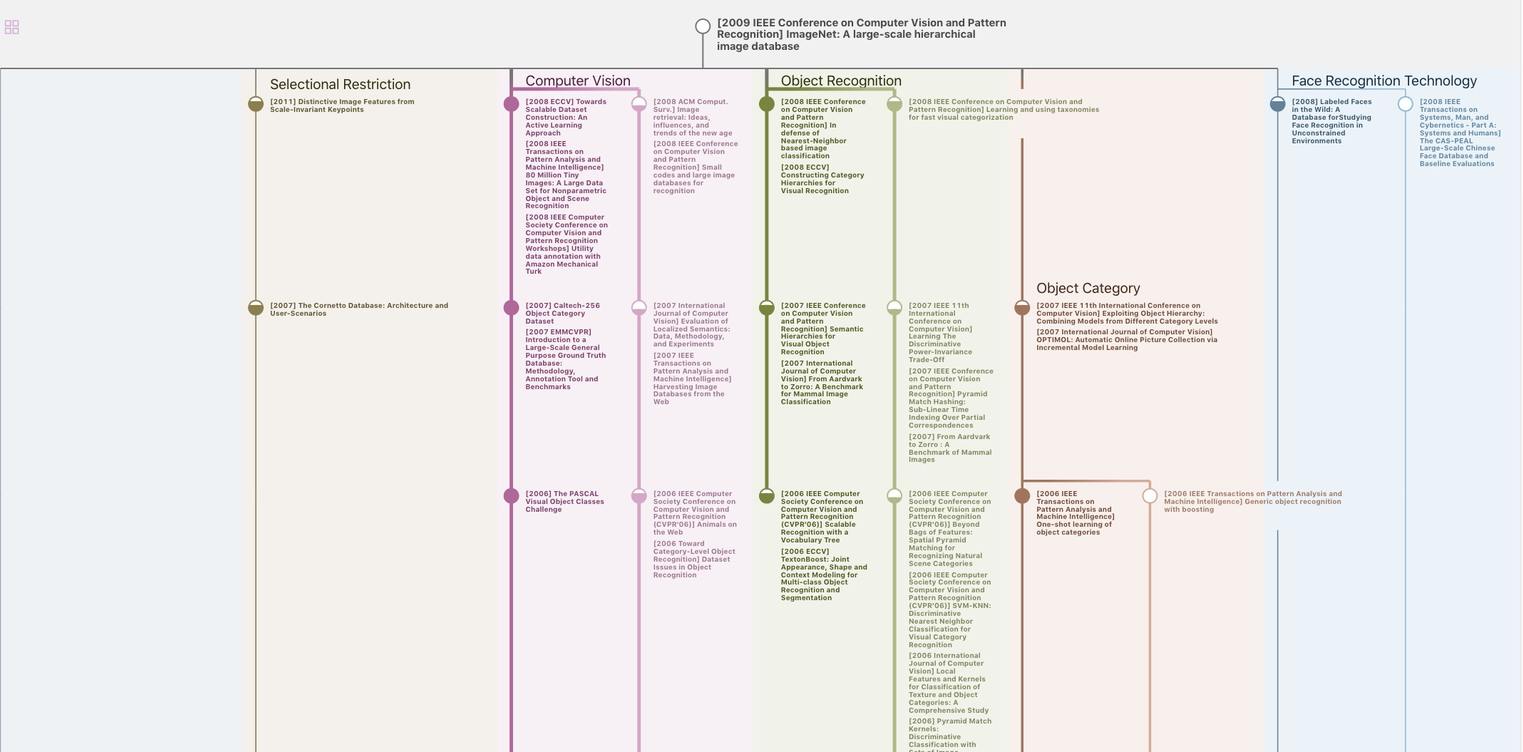
生成溯源树,研究论文发展脉络
Chat Paper
正在生成论文摘要