Few-Shot Classification With Feature Reconstruction Bias
2022 Asia-Pacific Signal and Information Processing Association Annual Summit and Conference (APSIPA ASC)(2022)
Abstract
Few-shot classification aims to classify unseen samples by learning from very few labeled samples. Very recently, reconstruction-based methods have been proposed and shown superior performance on few-shot fine-grained image classification, which, on top of the challenge of few labeled samples, faces the difficulty of identifying subtle differences between sub-categories. In essence, these methods reconstruct unseen samples from few seen samples and use the distance between the original unseen samples and their reconstruction as the criterion for classification. However, as pointed out in this paper, a bias is introduced in the overall distribution between the reconstructed features and original features, which consequently affects the distance calculation and subsequent classification. To address this issue, we propose a new concept of Feature Reconstruction Bias (FRB), which can be computed easily in the training stage without introducing any new parameters. Moreover, we propose to use this bias to correct query features in the test stage, which is shown to increase inter-class distances and decrease intra-class distances. Experiments on four fine-grained benchmarks demonstrate the effectiveness of our approach, with state-of-the-art performance achieved in most scenarios.
MoreTranslated text
Key words
classification,reconstruction,few-shot
AI Read Science
Must-Reading Tree
Example
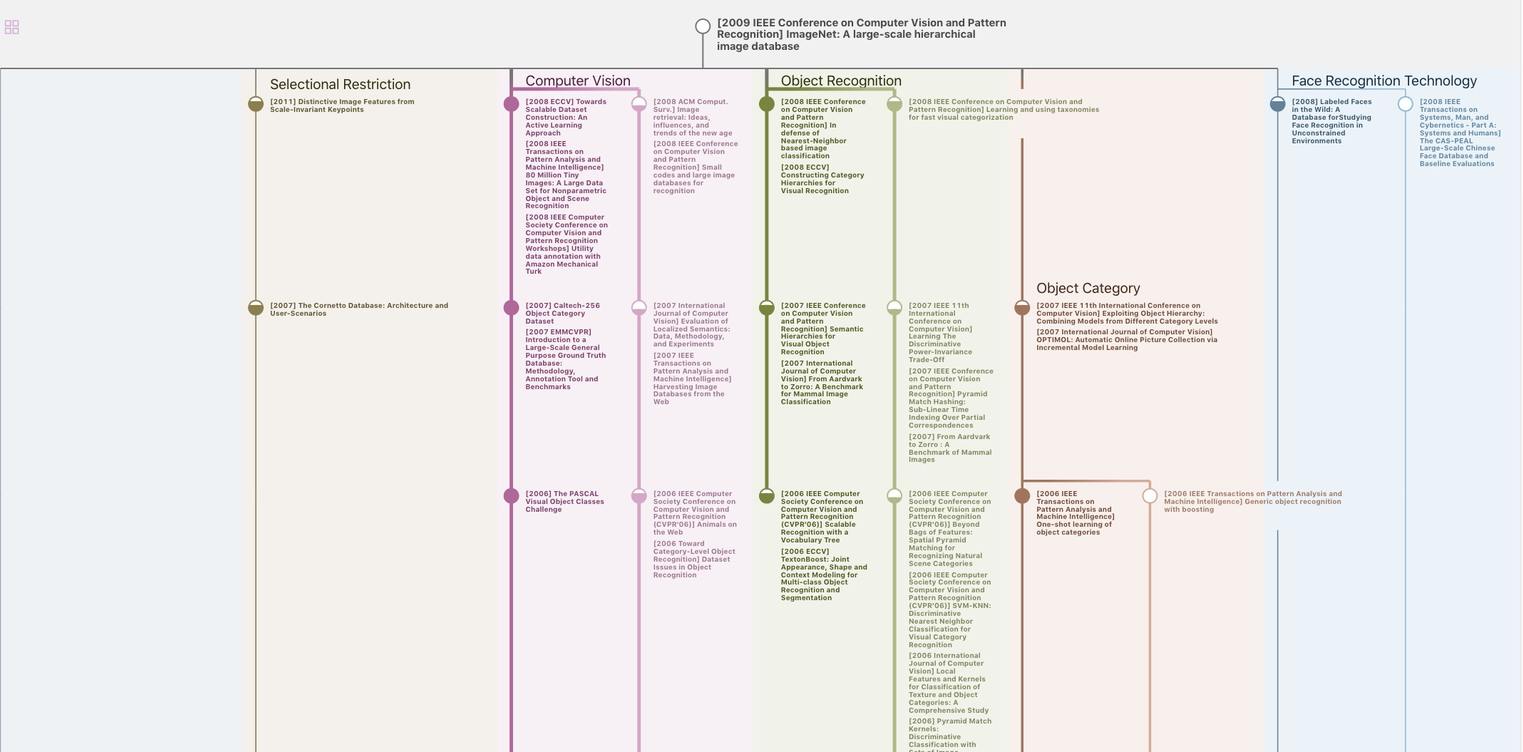
Generate MRT to find the research sequence of this paper
Chat Paper
Summary is being generated by the instructions you defined