Benchmarking Large Language Models for Automated Verilog RTL Code Generation
2023 DESIGN, AUTOMATION & TEST IN EUROPE CONFERENCE & EXHIBITION, DATE(2023)
摘要
Automating hardware design could obviate a significant amount of human error from the engineering process and lead to fewer errors. Verilog is a popular hardware description language to model and design digital systems, thus generating Verilog code is a critical first step. Emerging large language models (LLMs) are able to write high-quality code in other programming languages. In this paper, we characterize the ability of LLMs to generate useful Verilog. For this, we fine-tune pre-trained LLMs on Verilog datasets collected from GitHub and Verilog textbooks. We construct an evaluation framework comprising test-benches for functional analysis and a flow to test the syntax of Verilog code generated in response to problems of varying difficulty. Our findings show that across our problem scenarios, the fine-tuning results in LLMs more capable of producing syntactically correct code (25.9% overall). Further, when analyzing functional correctness, a fine-tuned open-source CodeGen LLM can outperform the state-of-the-art commercial Codex LLM (6.5% overall). We release our training/evaluation scripts and LLM checkpoints as open source contributions.
更多查看译文
关键词
Transformers,Verilog,GPT,LLM
AI 理解论文
溯源树
样例
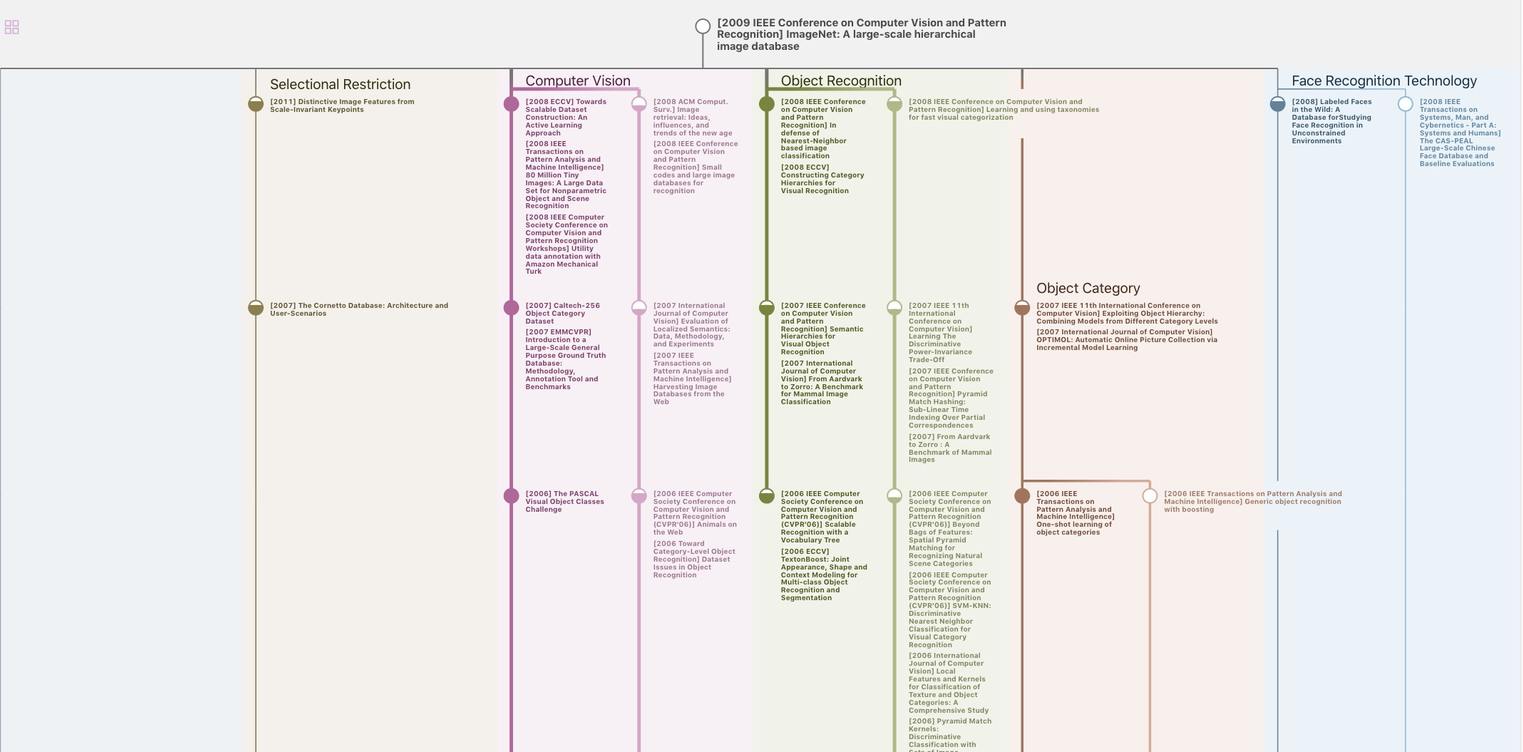
生成溯源树,研究论文发展脉络
Chat Paper
正在生成论文摘要