Diamond Abrasive Electroplated Surface Anomaly Detection using Convolutional Neural Networks for Industrial Quality Inspection
arxiv(2022)
摘要
Electroplated diamond abrasive tools require nickel coating on a metal surface for abrasive bonding and part functionality. The electroplated nickel-coated abrasive tool is expected to have a high-quality part performance by having a nickel coating thickness of between 50% to 60% of the abrasive median diameter, uniformity of the nickel layer, abrasive distribution over the electroplated surface, and bright gloss. Electroplating parameters are set accordingly for this purpose. Industrial quality inspection for defects of these abrasive electroplated parts with optical inspection instruments is extremely challenging due to the diamond's light refraction, dispersion nature, and reflective bright nickel surface. The difficulty posed by this challenge requires parts to be quality inspected manually with an eye loupe that is subjective and costly. In this study, we use a Convolutional Neural Network (CNN) model in the production line to detect abrasive electroplated part anomalies allowing us to fix or eliminate those parts or elements that are in bad condition from the production chain and ultimately reduce manual quality inspection cost. We used 744 samples to train our model. Our model successfully identified over 99% of the parts with an anomaly. Keywords: Artificial Intelligence, Anomaly Detection, Industrial Quality Inspection, Electroplating, Diamond Abrasive Tool
更多查看译文
AI 理解论文
溯源树
样例
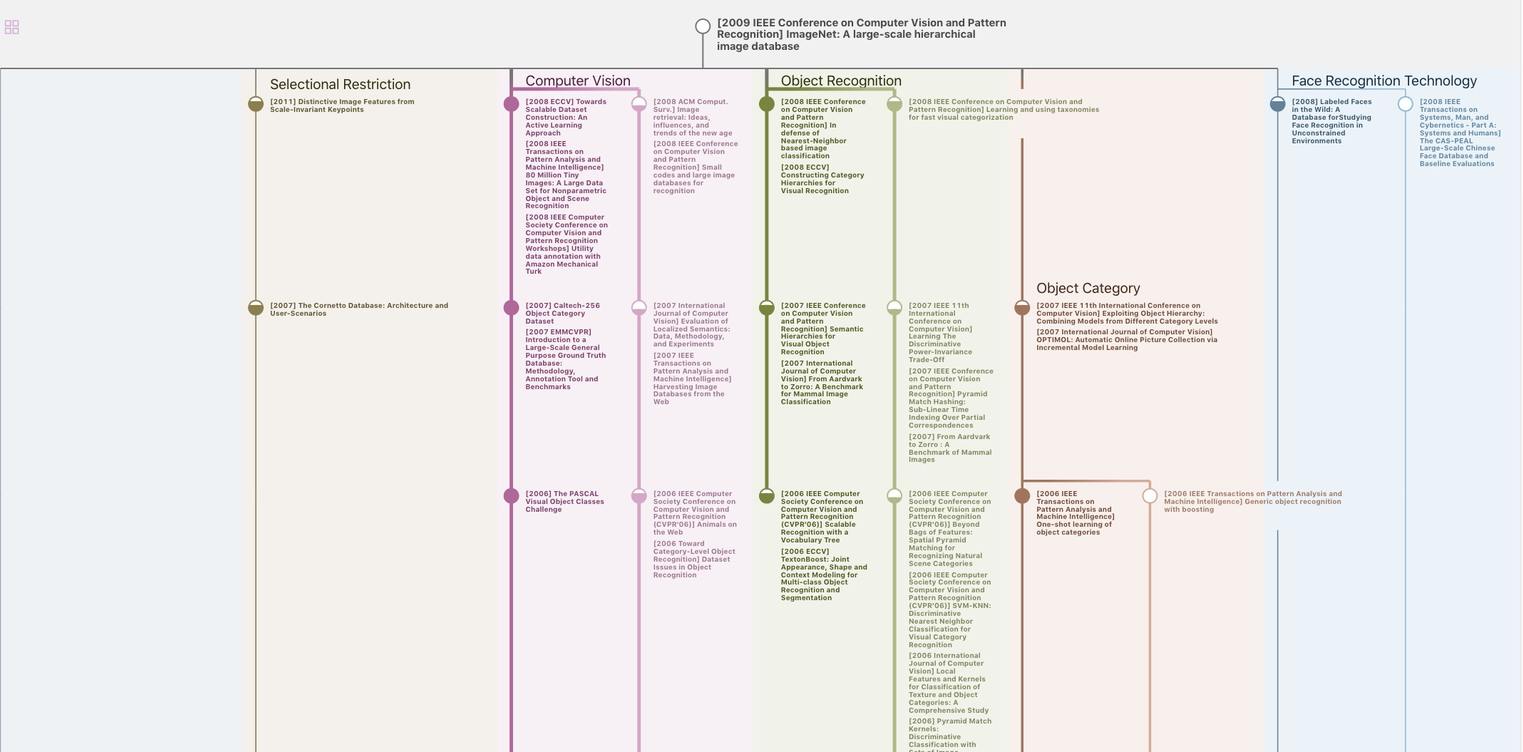
生成溯源树,研究论文发展脉络
Chat Paper
正在生成论文摘要