Object detection-based inspection of power line insulators: Incipient fault detection in the low data-regime
arxiv(2022)
摘要
Deep learning-based object detection is a powerful approach for detecting faulty insulators in power lines. This involves training an object detection model from scratch, or fine tuning a model that is pre-trained on benchmark computer vision datasets. This approach works well with a large number of insulator images, but can result in unreliable models in the low data regime. The current literature mainly focuses on detecting the presence or absence of insulator caps, which is a relatively easy detection task, and does not consider detection of finer faults such as flashed and broken disks. In this article, we formulate three object detection tasks for insulator and asset inspection from aerial images, focusing on incipient faults in disks. We curate a large reference dataset of insulator images that can be used to learn robust features for detecting healthy and faulty insulators. We study the advantage of using this dataset in the low target data regime by pre-training on the reference dataset followed by fine-tuning on the target dataset. The results suggest that object detection models can be used to detect faults in insulators at a much incipient stage, and that transfer learning adds value depending on the type of object detection model. We identify key factors that dictate performance in the low data-regime and outline potential approaches to improve the state-of-the-art.
更多查看译文
AI 理解论文
溯源树
样例
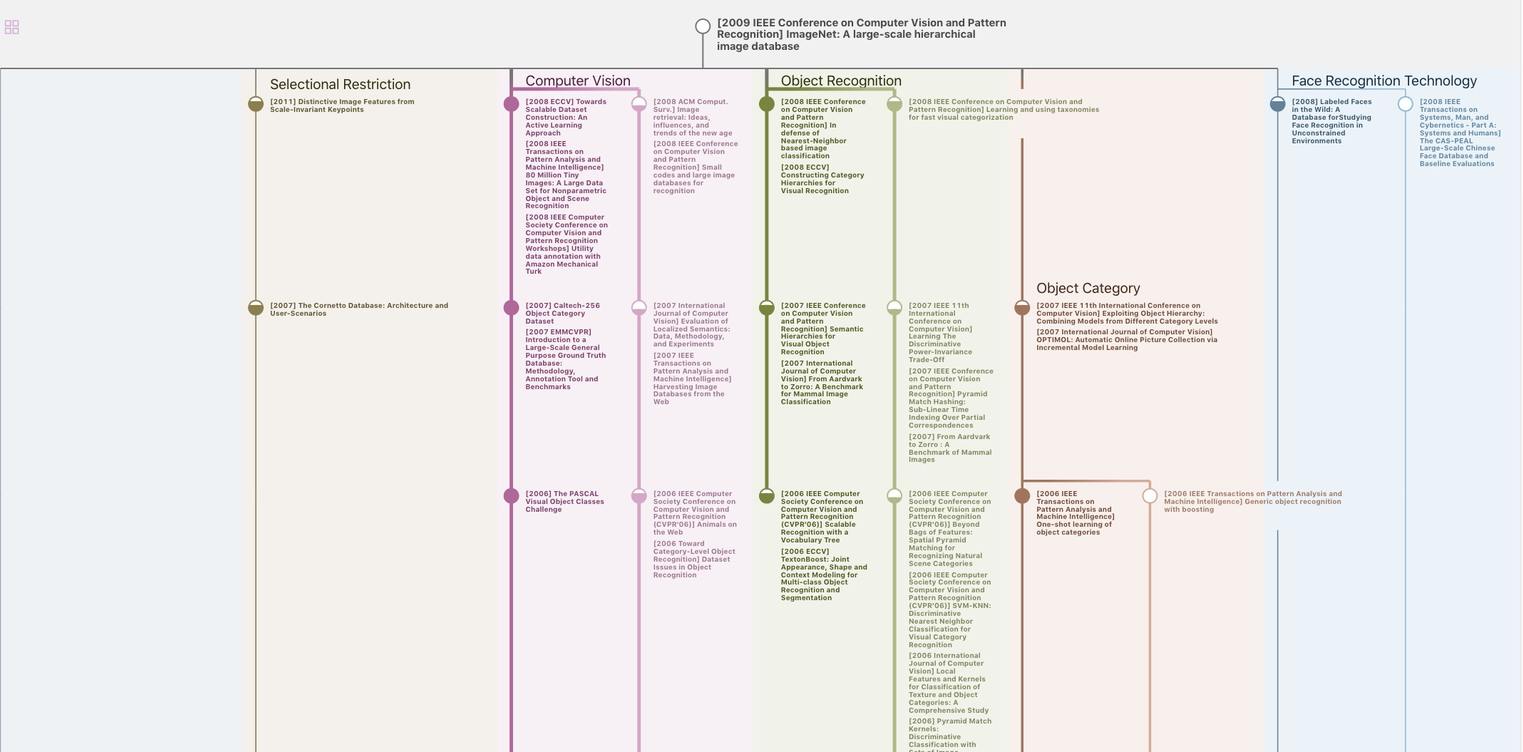
生成溯源树,研究论文发展脉络
Chat Paper
正在生成论文摘要