Prediction of steel nanohardness by using graph neural networks on surface polycrystallinity maps
Scripta Materialia(2022)
摘要
As a bulk mechanical property, nanoscale hardness in polycrystalline metals is strongly dependent on microstructural features that are believed to be heavily influenced from complex features of polycrystallinity -- namely, individual grain orientations and neighboring grain properties. We train a graph neural network (GNN) model, with each grain center location being a graph node, to assess the predictability of micromechanical responses of nano-indented low-carbon 310S stainless steel (alloyed with Ni and Cr) surfaces, solely based on surface polycrystallinity, captured by electron backscatter diffraction maps. The grain size distribution ranges between $1-100~\mu$m, with mean grain size at $18~\mu$m. The GNN model is trained on a set of nanomechanical load-displacement curves, obtained from nanoindentation tests and is subsequently used to make predictions of nano-hardness at various depths, with sole input being the grain locations and orientations. Model training is based on a sub-standard set of $\sim10^2$ hardness measurements, leading to an overall satisfactory performance. We explore model performance and its dependence on various structural/topological grain-level descriptors, such as the grain size and number of nearest neighbors. Analogous GNN model frameworks may be utilized for quick, inexpensive hardness estimates, for guidance to detailed nanoindentation experiments, akin to cartography tool developments in the world exploration era.
更多查看译文
关键词
Modeling, Hall-Petch effect, Hardness, Graph Neural Network, Misorientation
AI 理解论文
溯源树
样例
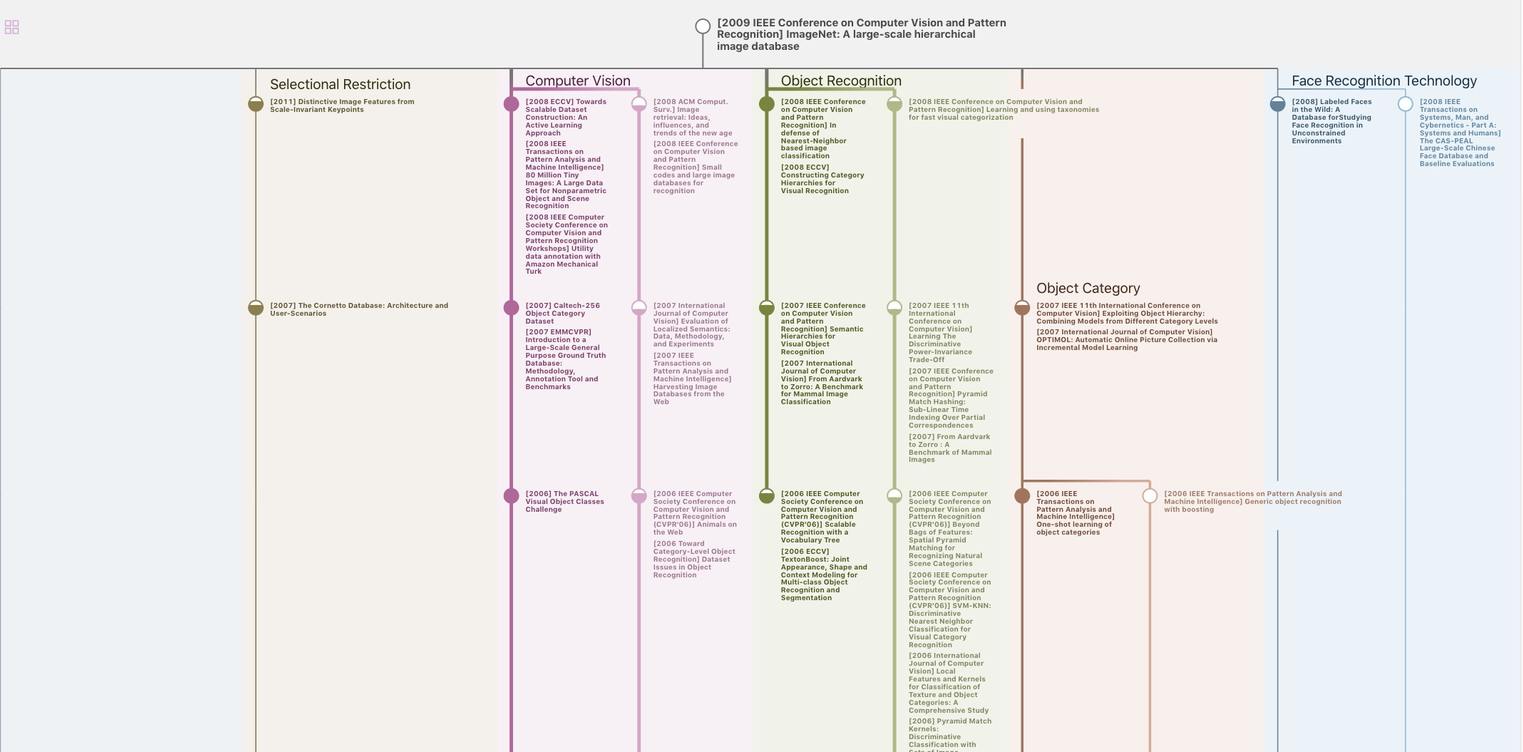
生成溯源树,研究论文发展脉络
Chat Paper
正在生成论文摘要