OpineSum: Entailment-based self-training for abstractive opinion summarization
conf_acl(2022)
摘要
A typical product or place often has hundreds of reviews, and summarization of these texts is an important and challenging problem. Recent progress on abstractive summarization in domains such as news has been driven by supervised systems trained on hundreds of thousands of news articles paired with human-written summaries. However for opinion texts, such large scale datasets are rarely available. Unsupervised methods, self-training, and few-shot learning approaches bridge that gap. In this work, we present a novel self-training approach, OpineSum, for abstractive opinion summarization. The summaries in this approach are built using a novel application of textual entailment and capture the consensus of opinions across the various reviews for an item. This method can be used to obtain silver-standard summaries on a large scale and train both unsupervised and few-shot abstractive summarization systems. OpineSum achieves state-of-the-art performance in both settings.
更多查看译文
关键词
abstractive opinion
AI 理解论文
溯源树
样例
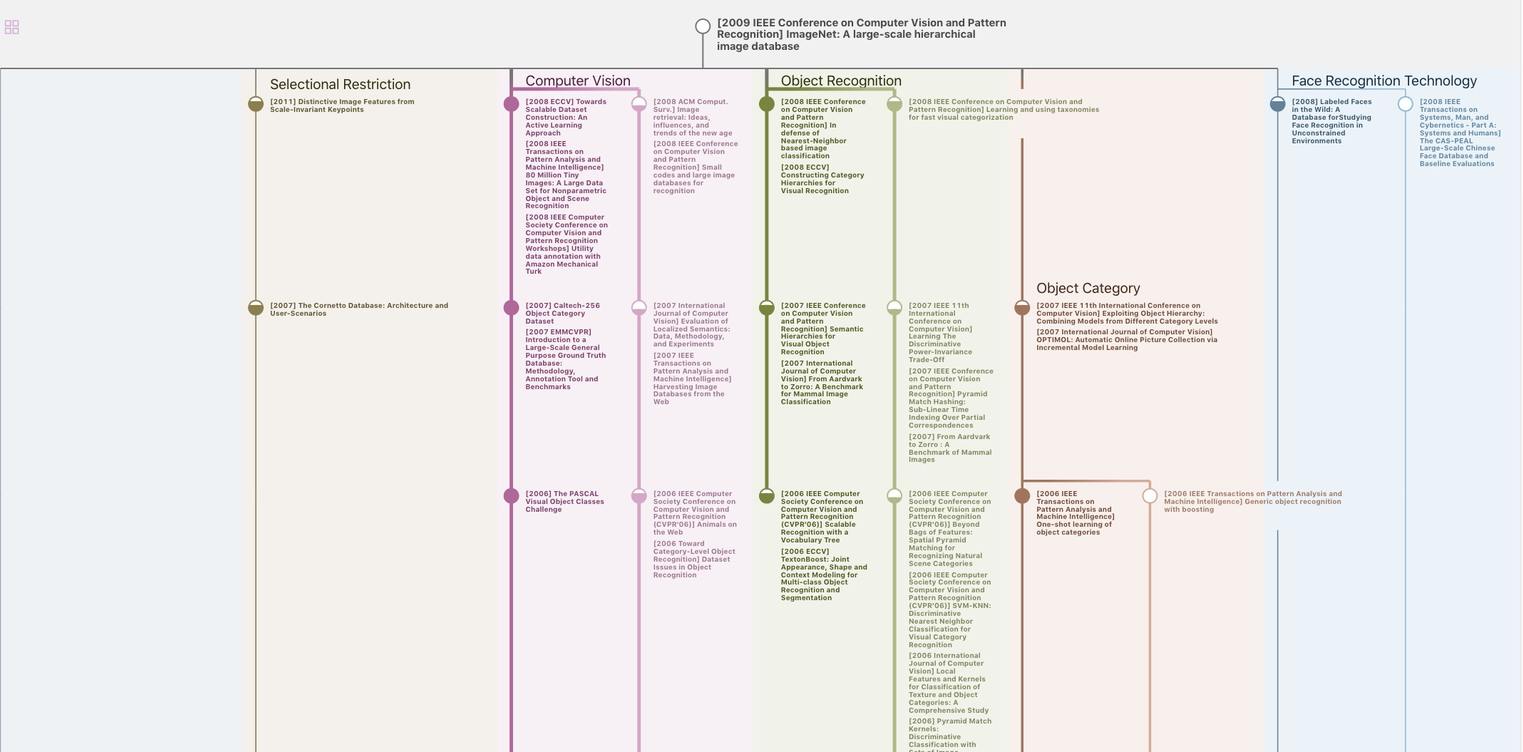
生成溯源树,研究论文发展脉络
Chat Paper
正在生成论文摘要