Investigation of Network Architecture for Multimodal Head-and-Neck Tumor Segmentation
arxiv(2022)
摘要
Inspired by the recent success of Transformers for Natural Language Processing and vision Transformer for Computer Vision, many researchers in the medical imaging community have flocked to Transformer-based networks for various main stream medical tasks such as classification, segmentation, and estimation. In this study, we analyze, two recently published Transformer-based network architectures for the task of multimodal head-and-tumor segmentation and compare their performance to the de facto standard 3D segmentation network - the nnU-Net. Our results showed that modeling long-range dependencies may be helpful in cases where large structures are present and/or large field of view is needed. However, for small structures such as head-and-neck tumor, the convolution-based U-Net architecture seemed to perform well, especially when training dataset is small and computational resource is limited.
更多查看译文
关键词
Tumor Segmentation,Training Dataset,Computational Resources,Large Structures,Large Field Of View,Long-range Dependencies,Vision Transformer,Primary Tumor,Input Image,Range Of Intensities,Semantic Segmentation,Dependent Model,Isotropic Resolution,U-Net Model
AI 理解论文
溯源树
样例
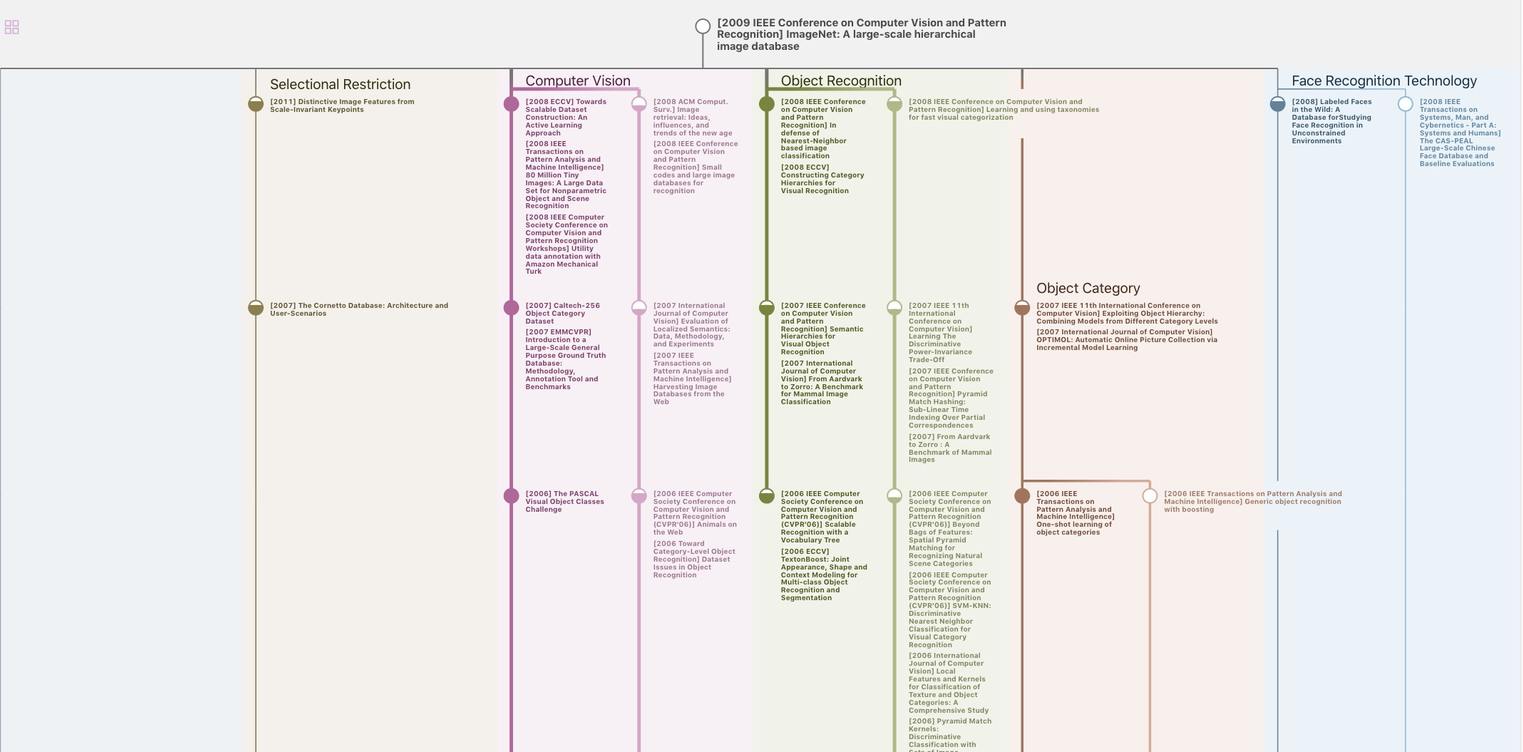
生成溯源树,研究论文发展脉络
Chat Paper
正在生成论文摘要