Neighboring state-based RL Exploration
arxiv(2023)
摘要
Reinforcement Learning is a powerful tool to model decision-making processes. However, it relies on an exploration-exploitation trade-off that remains an open challenge for many tasks. In this work, we study neighboring state-based, model-free exploration led by the intuition that, for an early-stage agent, considering actions derived from a bounded region of nearby states may lead to better actions when exploring. We propose two algorithms that choose exploratory actions based on a survey of nearby states, and find that one of our methods, ${\rho}$-explore, consistently outperforms the Double DQN baseline in an discrete environment by 49\% in terms of Eval Reward Return.
更多查看译文
关键词
exploration,state-based
AI 理解论文
溯源树
样例
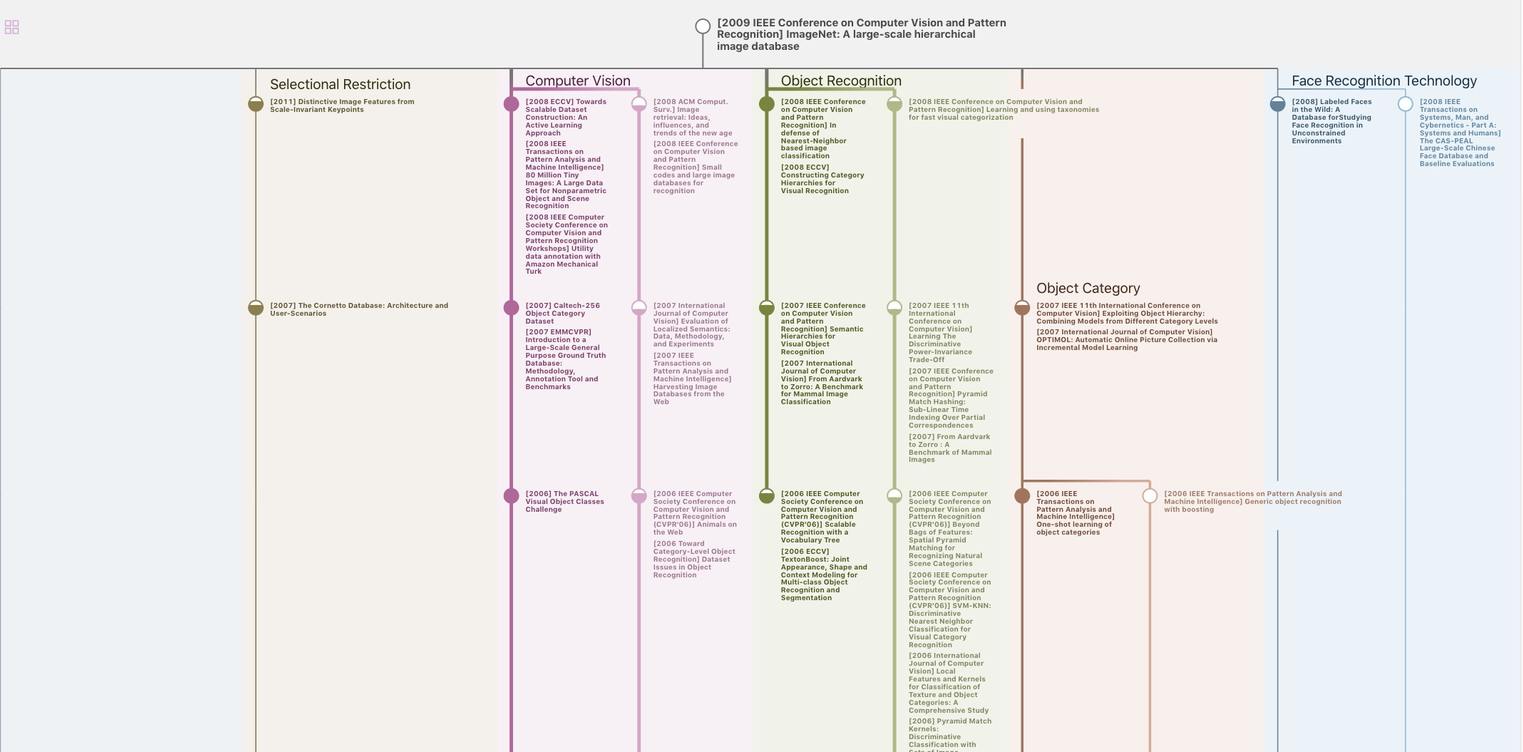
生成溯源树,研究论文发展脉络
Chat Paper
正在生成论文摘要