Dual Prototypical Network for Robust Few-shot Image Classification
2022 Asia-Pacific Signal and Information Processing Association Annual Summit and Conference (APSIPA ASC)(2022)
摘要
Deep neural networks have outperformed humans on some image recognition and classification tasks. However, with the emergence of various novel classes, it remains a chal-lenge to continuously expand the learning capability of such networks from a limited number of labeled samples. Metric-based approaches have been playing a key role in few-shot image classification, but most of them measure the distance between samples in the metric space using only a single metric function. In this paper, we propose a Dual Prototypical Network (DPN) to improve the test-time robustness of the classical prototypical network. The proposed method not only focuses on the distance of the original features, but also adds perturbation noise to the image and calculates the distance of noisy features. By enforcing the model to predict well under both metrics, more representative and robust class prototypes are learned and thus lead to better generalization performance. We validate our method on three fine-grained datasets in both clean and noisy settings.
更多查看译文
关键词
classification,dual,image,few-shot
AI 理解论文
溯源树
样例
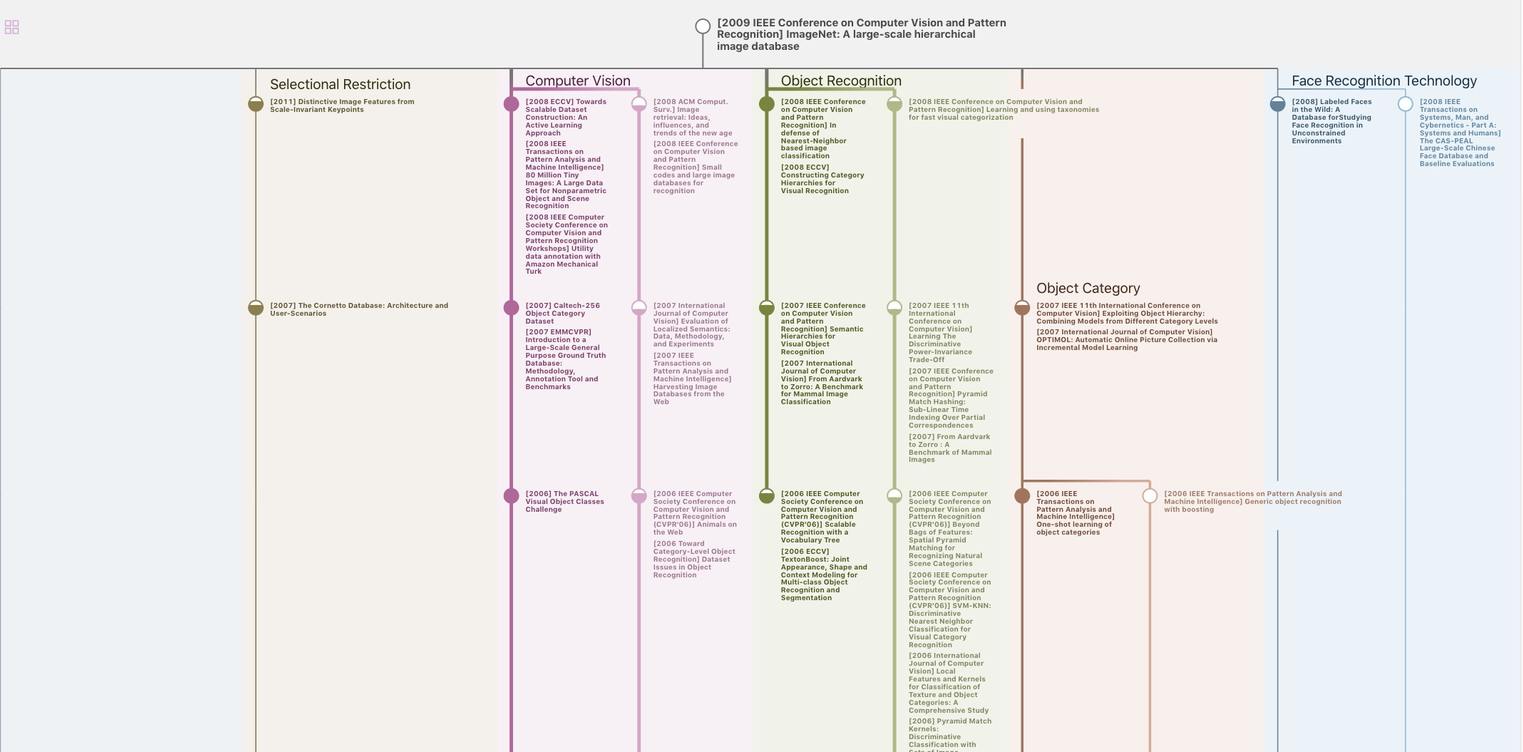
生成溯源树,研究论文发展脉络
Chat Paper
正在生成论文摘要