Radar Point Clouds Processing for Human Activity Classification using Convolutional Multilinear Subspace Learning
IEEE Transactions on Geoscience and Remote Sensing(2022)
摘要
Radar-based human activity classification is crucial for applications such as healthcare monitoring, fall detection, and assisted living due to its superior sensing capabilities and privacy protection. Traditional classification methods generally retrieve features from the time-range domain or the time-frequency (TF) domain. Such 2-D representation neglects the underlying dependence between the three radar signal variables of time, range, and Doppler frequency, and cannot fully depict the dynamic human motion features. In this article, we propose a time-range-Doppler radar point clouds (RPCs)-based learning model for human activity classification using a frequency-modulated continuous waveform (FMCW) radar. The human echoes are first transformed into a series of 3-D point cloud cubes integrating the motion signatures in three domains, namely time-range, time-Doppler, and range-Doppler domains. The generated RPC cubes are then fed into a newly developed two-layer convolutional multilinear principal component analysis network (CMPCANet) for feature extraction and motion classification. The CMPCANet comprises a simple network architecture with small training parameters, and can be directly implemented on the 3-D tensor dataset to extract highly discriminative features. Experimental results demonstrate that proposed framework can achieve superior classification accuracy and noise robustness compared to other methods using multidomain information, even with small training samples.
更多查看译文
关键词
Human activity classification,micro-Doppler signatures,multilinear principal component analysis (PCA),radar point clouds (RPCs),range map
AI 理解论文
溯源树
样例
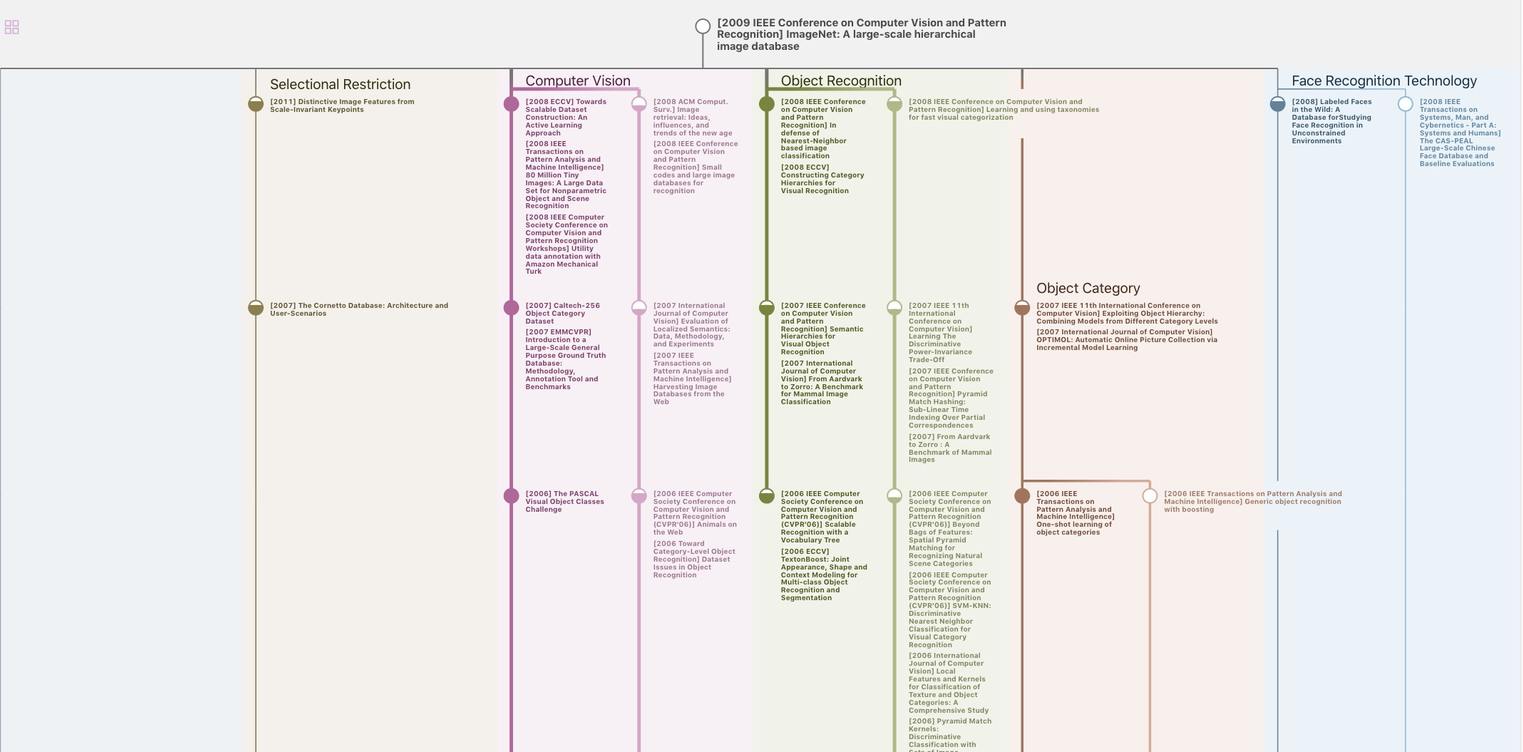
生成溯源树,研究论文发展脉络
Chat Paper
正在生成论文摘要