An inspection method of rail head surface defect via bimodal structured light sensors
International Journal of Machine Learning and Cybernetics(2022)
摘要
Rail defects have long threatened the safety of railway vehicles. Existing inspection methods still have some problems and flaws that can not meet practical application. In this paper, we propose a rail surface defect inspection method based on bimodal structured light sensors, termed Rail Surface Defect Inspection Network (RSDINet), which can detect and measure defect in bimodal rail images. To verify effect of the method, we establish a bimodal image dataset of intensity and depth images collected by the constructed bimodal structured light sensors data acquisition system. To solve the irregularity of rail surface defect shape, we propose a feature extraction backbone network by introducing deformable convolution. Moreover, RSDINet adopts a parallel feature extraction strategy to process bimodal images respectively. Specifically, we apply different backbone networks to bimodal images respectively for different image characteristics to enhance the feature representation ability of network. Then, our RSDINet fuses multi-scale feature of bimodal images respectively and carries out multi-scale rail surface defect detection and measurement. It is worth noting that the proposed RSDINet can accomplish these two tasks end-to-end simultaneously. Experiments demonstrate that based on the established dataset, the method achieves 87.17 mAP and 39.07 mSAP for detection and measurement respectively at 6.2 FPS on a single GPU, which has a better performance than previous SOTA methods and shows a promising potential for application in high-speed railway.
更多查看译文
关键词
Rail surface defect inspection,Defect severity measurement,Bimodal structured light sensors,Bimodal image fusion,Deep convolutional neural network,High-speed railway
AI 理解论文
溯源树
样例
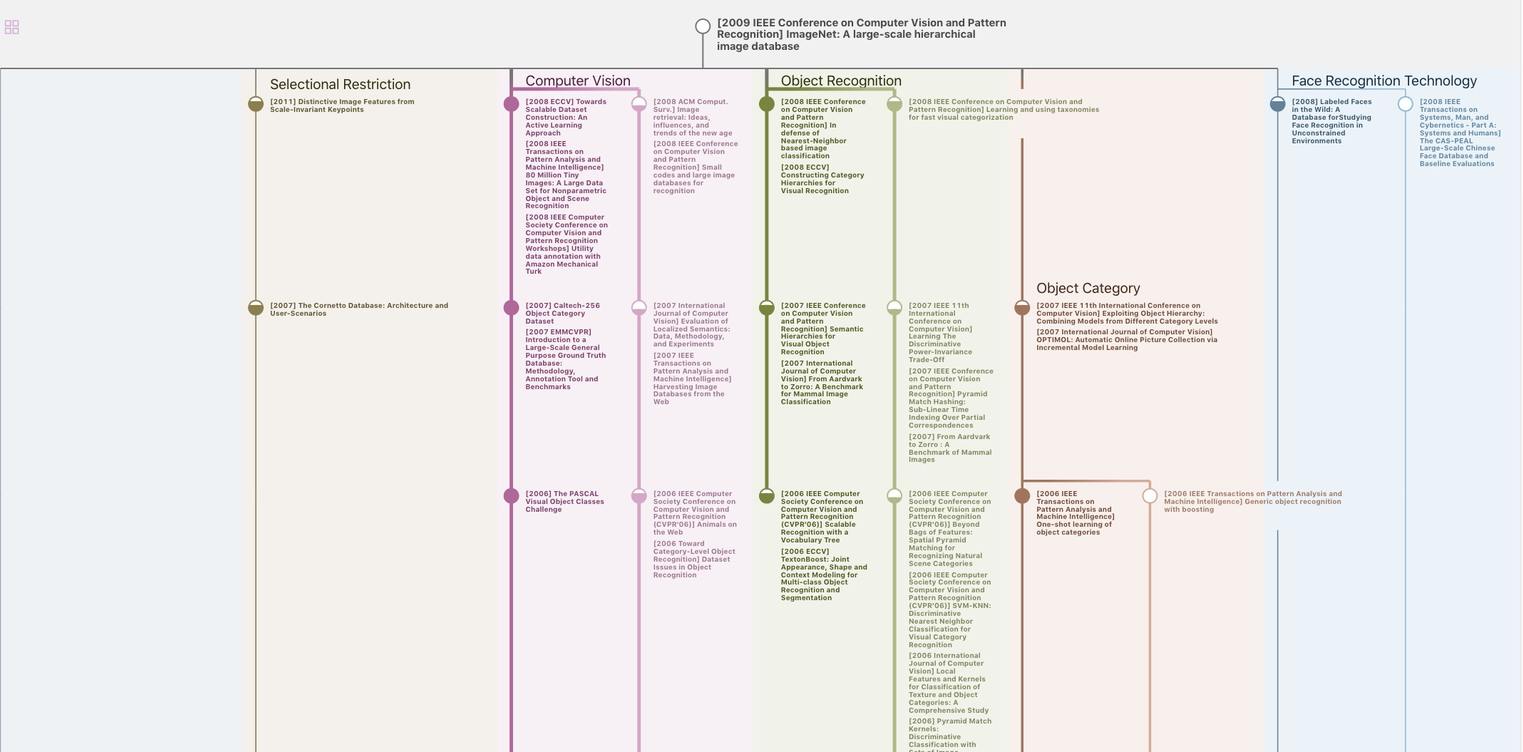
生成溯源树,研究论文发展脉络
Chat Paper
正在生成论文摘要