Radiomics-Based Machine Learning for Predicting the Injury Time of Rib Fractures in Gemstone Spectral Imaging Scans.
Bioengineering (Basel, Switzerland)(2022)
摘要
This retrospective study aimed to predict the injury time of rib fractures in distinguishing fresh (30 days) or old (90 days) rib fractures. We enrolled 111 patients with chest trauma who had been scanned for rib fractures at our hospital between January 2018 and December 2018 using gemstone spectral imaging (GSI). The volume of interest of each broken end of the rib fractures was segmented using calcium-based material decomposition images derived from the GSI scans. The training and testing sets were randomly assigned in a 7:3 ratio. All cases were divided into groups distinguishing the injury time at 30 and 90 days. We constructed radiomics-based models to predict the injury time of rib fractures. The model performance was assessed by the area under the curve (AUC) obtained by the receiver operating characteristic analysis. We included 54 patients with 259 rib fracture segmentations (34 men; mean age, 52 years ± 12.02; and range, 19-72 years). Nine features were excluded by the least absolute shrinkage and selection operator logistic regression to build the radiomics signature. For distinguishing the injury time at 30 days, the Support Vector Machine (SVM) model and human-model collaboration resulted in an accuracy and AUC of 0.85 and 0.871 and 0.91 and 0.912, respectively, and 0.81 and 0.804 and 0.83 and 0.85, respectively, at 90 days in the testing set. The radiomics-based model displayed good accuracy in differentiating between the injury time of rib fractures at 30 and 90 days, and the human-model collaboration generated more accurate outcomes, which may help to add value to clinical practice and distinguish artificial injury in forensic medicine.
更多查看译文
关键词
chest trauma,human-model collaboration,injury time,radiomics-based model,rib fractures
AI 理解论文
溯源树
样例
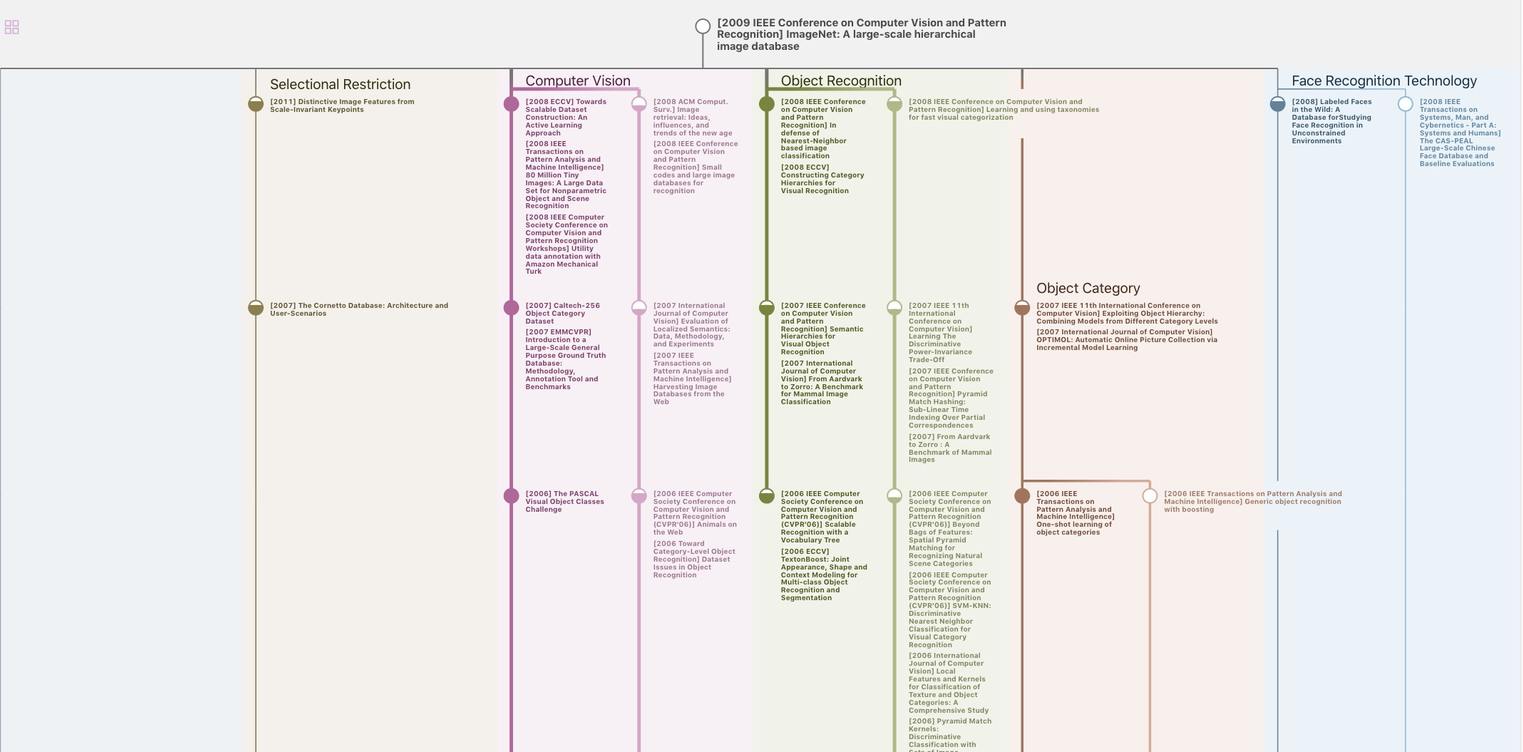
生成溯源树,研究论文发展脉络
Chat Paper
正在生成论文摘要