Improving estimation efficiency for two-phase, outcome-dependent sampling studies
arxiv(2023)
摘要
Two-phase outcome dependent sampling (ODS) is widely used in many fields, especially when certain covariates are expensive and/or difficult to measure. For two-phase ODS, the conditional maximum likelihood (CML) method is very attractive because it can handle zero Phase 2 selection probabilities and avoids modeling the covariate distribution. However, most existing CML-based methods use only the Phase 2 sample and thus may be less efficient than other methods. We propose a general empirical likelihood method that uses CML augmented with additional information in the whole Phase 1 sample to improve estimation efficiency. The proposed method maintains the ability to handle zero selection probabilities and avoids modeling the covariate distribution, but can lead to substantial efficiency gains over CML in the inexpensive covariates, or in the influential covariate when a surrogate is available, because of an effective use of the Phase 1 data. Simulations and a real data illustration using NHANES data are presented.
更多查看译文
关键词
Conditional likelihood, empirical likelihood, missing at random, two-phase study, expensive covariate, surrogate covariate
AI 理解论文
溯源树
样例
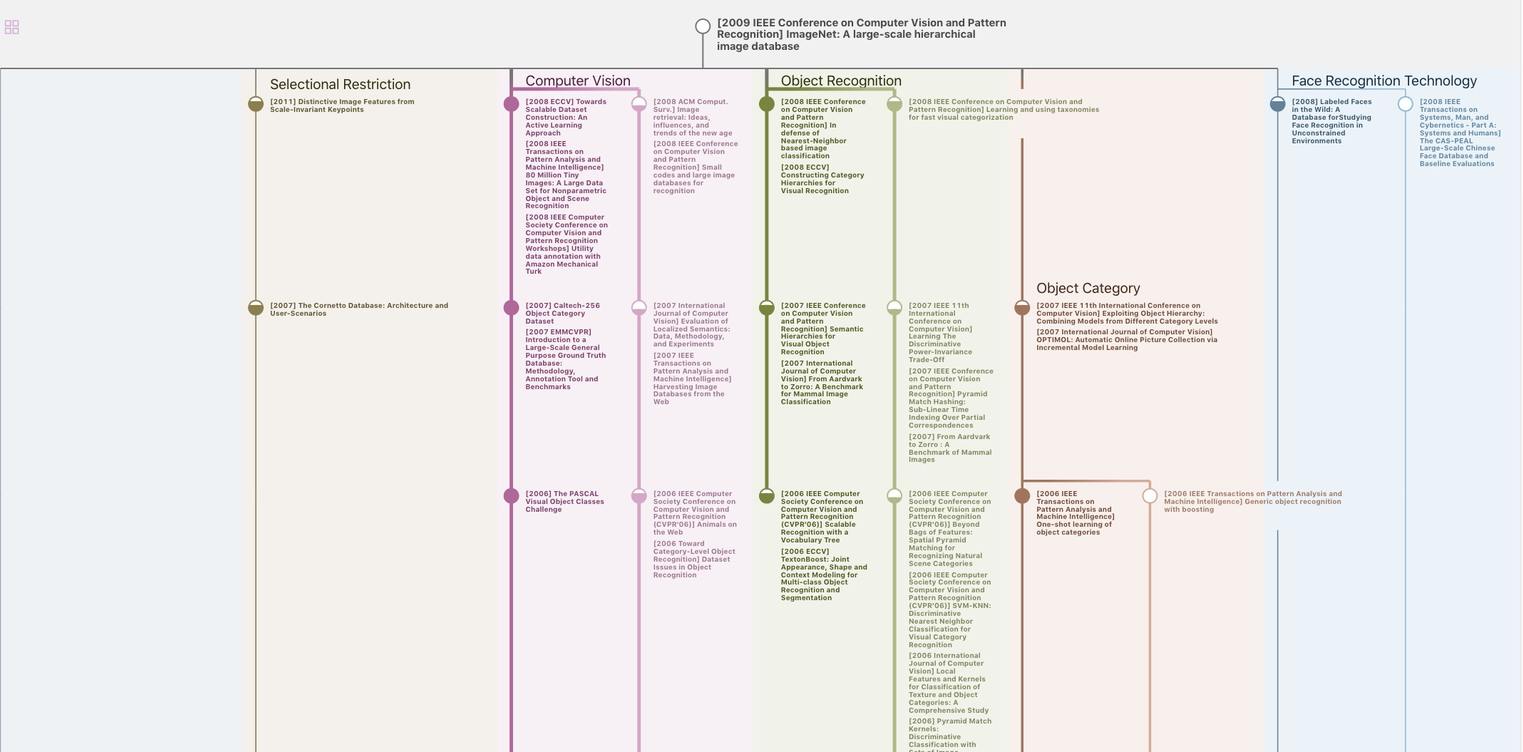
生成溯源树,研究论文发展脉络
Chat Paper
正在生成论文摘要