Fully Data-Driven Robust Output Formation Tracking Control for Heterogeneous Multiagent System With Multiple Leaders and Actuator Faults
IEEE TRANSACTIONS ON CYBERNETICS(2024)
摘要
This article investigates a fully data-driven method to solve the robust output formation tracking control problem for the multiagent system (MAS) under actuator faults. The outputs of the followers are controlled to track those of multiple leaders with respect to a convex point while achieving an expected time-varying formation. To obviate the requirement of various system prior knowledge in typical MAS control, a hierarchical frame is developed with three learning and control stages using the online measured data. First, a distributed adaptive observer is designed to coordinate the state convex of multiple leaders while estimating unknown dynamics. The adaptive mechanism relaxes the demand for global topology. Second, by collecting and reusing the online system data, an off-policy reinforcement learning (RL) method is proposed in a continuous form to acquire nominal feedback gains from partial observations of the followers. Essential system models are learned along with the RL process, while solutions to the output regulation equations are implicitly obtained. Third, a comprehensive robust controller is further presented based on the previous learning results. To address the actuator faults with efficiency loss and bias, the adaptive neural networks and robust compensations are utilized in a model-free manner. The output formation tracking is achieved under a derived feasibility condition while stabilities of the learning and control methods are analyzed. Finally, simulation results demonstrate the validity of this fully data-driven control frame.
更多查看译文
关键词
Adaptation models,Actuators,Q-learning,Observers,Multi-agent systems,Mathematical models,Fault tolerant systems,Adaptive neural networks (AdNNs),data-driven control,fault-tolerant,multiagent system (MAS),output formation tracking,partial observations,reinforcement learning (RL)
AI 理解论文
溯源树
样例
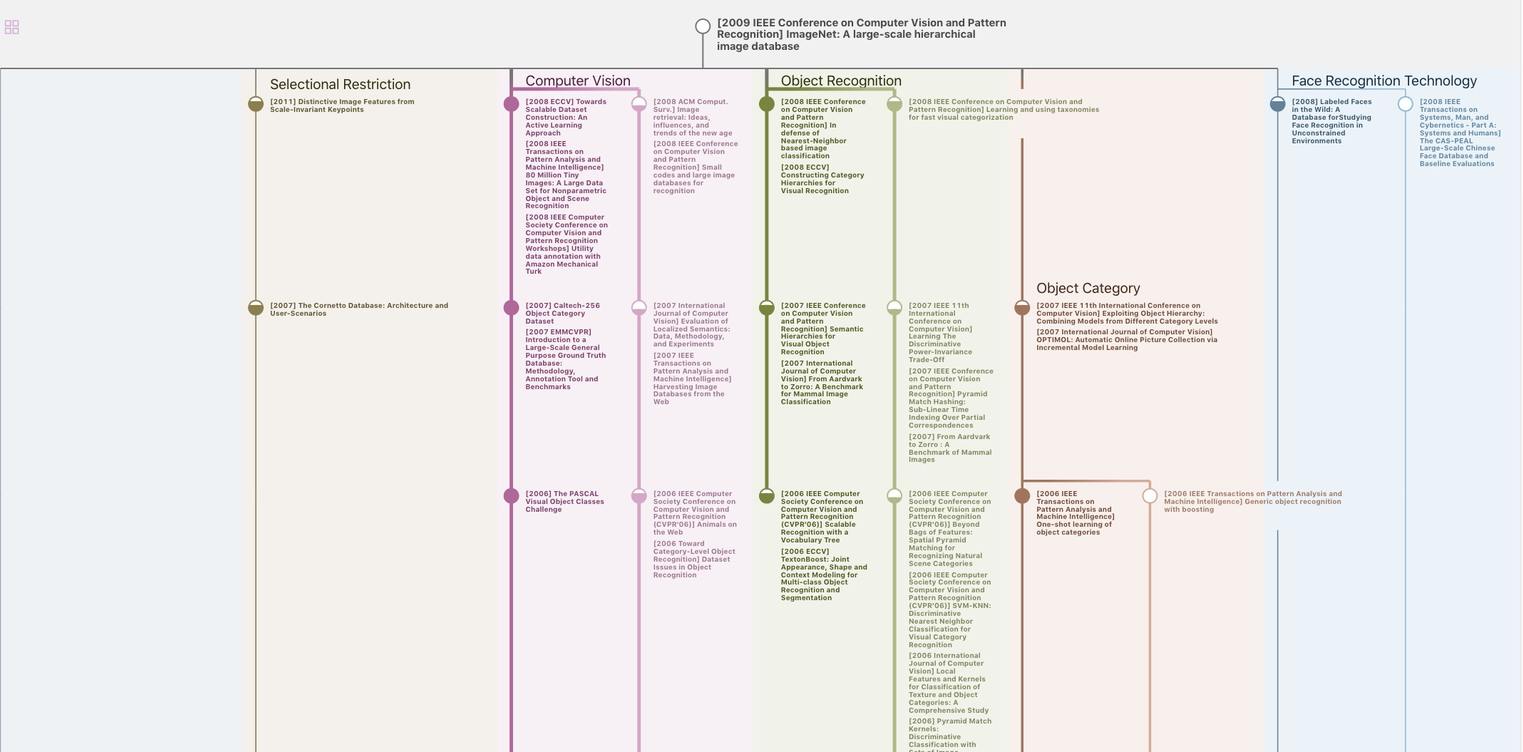
生成溯源树,研究论文发展脉络
Chat Paper
正在生成论文摘要