Automated method for segmenting enlarged perivascular spaces
Alzheimer's & Dementia(2022)
摘要
Abstract Background Enlarged perivascular spaces (ePVS) on magnetic resonance (MR) imaging are difficult to quantify due to their small size, diffuse distribution, and often, high prevalence. We present a method for automating ePVS volume quantification in the basal ganglia. Method Vanderbilt Memory and Aging Project participants (n=327, 73±7, 41% female) underwent MR imaging and serial neuropsychological assessment. Fifty T1‐weighted MR scans were manually coded for basal ganglia ePVS and used to train and test a deep learning U‐net. The model was then used to segment 277 remaining scans. Dice similarity coefficient (DSC), volume Pearson correlation, and mean symmetric surface distance (MSSD) were used to compare ground‐truth segmentations to predicted segmentations. In head‐to‐head regressions using pseudo‐conditional R 2 , basal ganglia ePVS volume and count were compared to two existing ordinal scores (Patankar et al., 2005; Hansen et al., 2015) to assess which method best predicted longitudinal cognition. Models adjusted for demographic, genetic, and risk factors. Result The basal ganglia deep learning model attained a mean DSC of 0.76 on testing images (n=10) with an ePVS volume correlation of 0.95 and MSSD of 1.16 mm. Next, to assess the utility of automated measures derived from the model, ePVS volume and count were compared to commonly used ordinal scores on cognitive trajectory over a mean follow‐up time of 5.2±1.7 years. All automated and ordinal ePVS predictors achieved comparable pseudo‐conditional R 2 values (e.g., 0.893 to 0.894 for episodic memory, 0.829 to 0.831 for executive function) on the longitudinal cognitive outcomes. ePVS volume had the highest or identical to the highest pseudo‐conditional R2 value on four of seven outcomes, including episodic memory (0.894), information processing (0.559), executive function (0.831), and visuospatial skills (0.743). Conclusion We present a deep learning model that reliably segments ePVS in the basal ganglia of T1‐weighted brain MR scans, creating regional volume measures of ePVS. Automated basal ganglia ePVS volume measures perform comparably to existing validated manual methods when predicting longitudinal cognition. Automated methods are less time‐consuming and produce a continuous measure, providing a more robust way to quantify longitudinal ePVS change. Funding: F31‐AG066358, IIRG‐08‐88733, R01‐AG034962, R01‐AG056534, K24‐AG046373, P20‐AG068082
更多查看译文
关键词
enlarged perivascular spaces
AI 理解论文
溯源树
样例
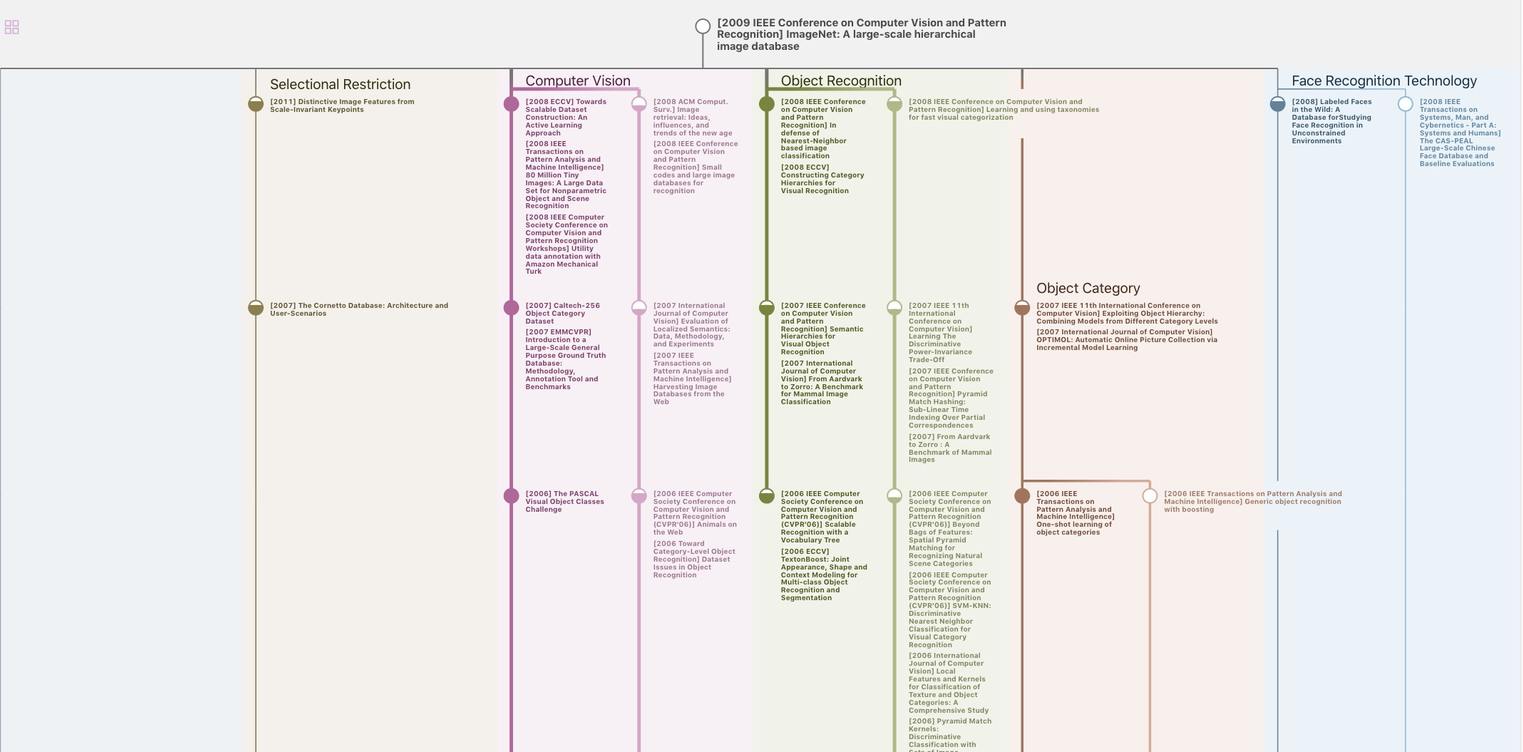
生成溯源树,研究论文发展脉络
Chat Paper
正在生成论文摘要