Tea-cake CBIR: a tea-cake content-based image retrieval model with rich and intensive feature extraction
The Visual Computer(2022)
摘要
Tea-cake content-based image retrieval (CBIR) is an essential issue in tea-cake traceability. Popular CBIR methods work on medical and social networking, whereas tea-cake images have intensive and parallel representations among classes. While facing tea-cake CBIR, low inter-class and high intra-class distances increase retrieval difficulties using traditional CBIR methods. Thus, this paper proposes a tea-cake CBIR approach based on deep neural networks to retrieve tea-cakes. In the model, we establish a feature extraction model with designed dense blocks, where a cross-entropy loss function is explored to train the model and catch detailed features. Furthermore, to decrease intra-class and expand inter-class interval, a masked autoregressive discriminative normalization flow (MADNF) is presented to map the gained features in high dimensions to corresponding representations in Gaussian spaces. Particularly, a maximum likelihood function is developed to train MADNF for avoiding non-convergence. Extensive experiments on the tea-cake dataset show our method has significant performance compared with current competitors. Furthermore, experiments on the bird species dataset further demonstrate the effectiveness of our proposed approach.
更多查看译文
关键词
Tea-cake image retrieval,Convolutional neural networks,Feature distance measurement,Normalization flow
AI 理解论文
溯源树
样例
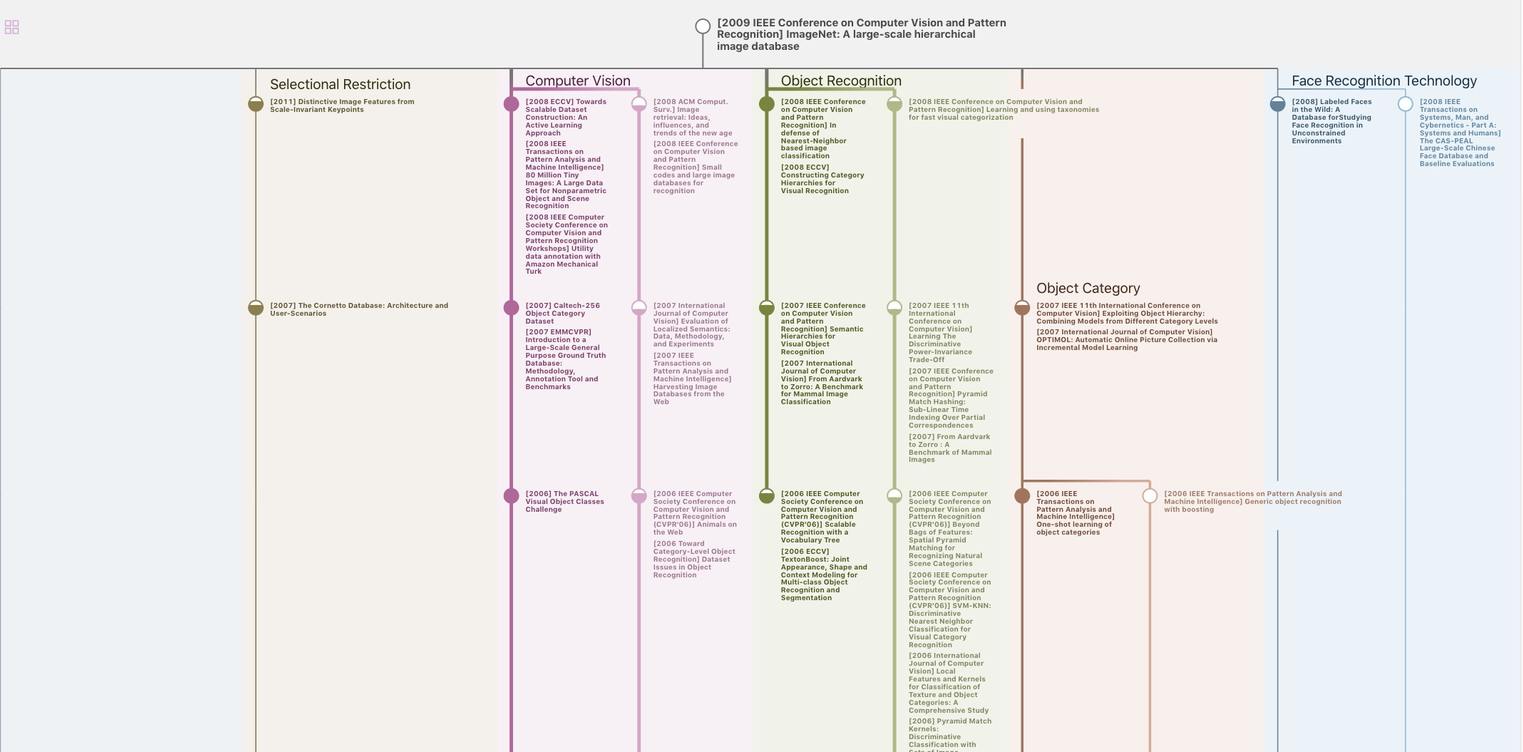
生成溯源树,研究论文发展脉络
Chat Paper
正在生成论文摘要