Predicting and analyzing flood susceptibility using boosting-based ensemble machine learning algorithms with SHapley Additive exPlanations
Natural Hazards(2022)
摘要
In recent years, the number of floods around the world has increased. As a result, Flood Susceptibility Maps (FSMs) became vital for flood prevention, risk mitigation, and decision-making. The purpose of this study is to develop FSMs for Adana province on the Mediterranean coast of Türkiye using tree-based machine learning (ML) classifiers. This study seeks to analyze the predictive performance of Natural Gradient Boosting Machines (NGBoost) for the first time in FSM studies, as well as the first comparative study of Light Gradient Boosting Machines (LightGBM) and CatBoost versus other techniques, including Random Forest (RF), Gradient Boosting (GB), eXtreme Gradient Boosting (XGBoost), and Adaptive Boosting (AdaBoost). These ML approaches were evaluated using fourteen flood conditioning parameters divided into five categories: topographical, meteorological, vegetation, lithological, and anthropogenic. The AdaBoost and LightGBM models scored the highest in terms of test accuracy (0.8978), followed by GB and NGBoost (0.8832), XGBoost (0.8759), RF (0.8613), and CatBoost (0.8102). A McNemar's test was used to determine the statistical significance of classifier predictions. According to the FSMs generated, Adana province has a substantial quantity of land that is moderately to extremely prone to flooding. For feature selection, the majority of previous studies used solely the Information Gain (IG) method and multicollinearity analysis. However, only a few studies used global explanatory models to calculate the relevance of their conditioning factors. A locally explained model is required to understand the associations and dependencies between each conditioning factor. Therefore, this study locally explains the generated ML-based FSMs with the help of an eXplainable Artificial Intelligence (XAI) approach, namely SHapley Additive exPlanations (SHAP). According to the findings, elevation, slope, and distance to rivers are the top three contributing factors in most models. SHAP results show that lower elevations, lower slopes, areas closer to river banks, agricultural areas, and sparsely vegetated areas are shown to be more prone to flooding.
更多查看译文
关键词
Flood susceptibility mapping,NGBoost,LightGBM,CatBoost,SHapley Additive exPlanations
AI 理解论文
溯源树
样例
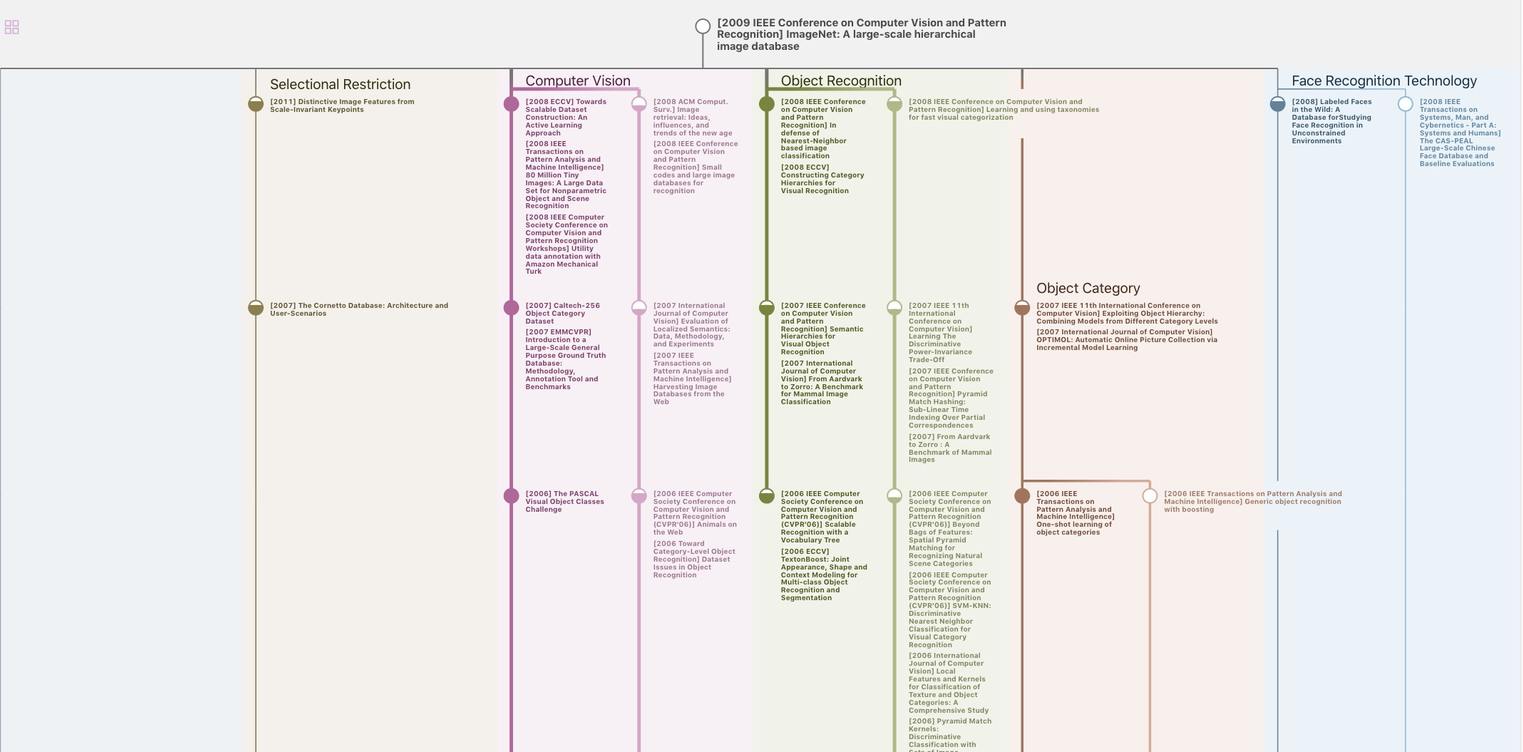
生成溯源树,研究论文发展脉络
Chat Paper
正在生成论文摘要