An Unsupervised Data‐Driven Approach to Profiling Distinct Tau Progression Patterns
Alzheimer's & Dementia(2022)
摘要
Abstract Background Given the central role that accumulation of tau pathology plays in the clinical progression of Alzheimer’s disease, the ability to quantify and predict the spread of tau is of great interest. Due to observed heterogeneity in patterns of tau deposition, though, stratification of individuals into homogenous phenotypes is critical to better understanding the natural course of this pathology progression, and ultimately the clinical disease progression. We propose an unsupervised data‐driven whole‐brain pattern analysis approach leveraging the spatially rich information provided by longitudinal tau‐PET, first to identify distinct tau accumulation profiles from longitudinal observation data and then to build baseline models predictive of tau accumulation type. Method Longitudinal ADNI flortaucipir‐PET images (N=530) were normalized to cerebellar‐uptake and corrected for partial‐volume‐effects to estimate flortaucipir‐burden for Braak regions I, III, IV, V, and VI. Braak II region was excluded due to spill‐in from choroid‐plexus. A mixture of multivariate generalized linear mixed models was used as an unsupervised data‐driven longitudinal clustering approach to identify distinct flortaucipir‐progression profiles with random‐intercept to analyze flortaucipir‐burden from all Braak regions and all time‐points simultaneously (i.e., multivariate longitudinal feature‐space) while accounting for inter‐regional dependence and irregular time sampling. Multiclass random‐forest classification (MRFC) was employed to predict the identified flortaucipir‐progression profiles using baseline variables. Result Three distinct flortaucipir‐progression profiles were identified: 1) “stable” with minimal flortaucipir‐accumulation across all Braak regions; 2) “moderate‐accumulator” with slow (1.01‐2.35 %SUVR/year) flortaucipir‐accumulation rates within Braak I, III, IV, and V; and 3) “fast‐accumulator” with accelerated (1.07‐4.03 %SUVR/year) flortaucipir‐accumulation rates in Braak IV, V, and VI. Prevalence of moderate to fast flortaucipir‐progression profiles increased with Aβ‐positivity, cognitive impairment, age and APOE‐ϵ4. Majority of Aβ‐negative cognitively unimpaired individuals fell within the "stable” category while Aβ‐positive individuals with dementia presented with moderate to fast flortaucipir‐progression profiles. MRFC trained on baseline demographic/clinical characteristics and baseline Braak flortaucipir burdens (60%/40% train/test) differentiated the three flortaucipir‐progression groups with a balanced accuracy of 93% (sensitivity=92%; specificity=95%). Conclusion We derived data‐driven distinct longitudinal tau‐PET patterns that characterize the flortaucipir‐progression differences in ADNI. The ability to detect flortaucipir‐progression with baseline imaging and non‐imaging markers could have potential to screen high‐risk individuals for accelerated pathology progression.
更多查看译文
关键词
patterns,profiling,progression
AI 理解论文
溯源树
样例
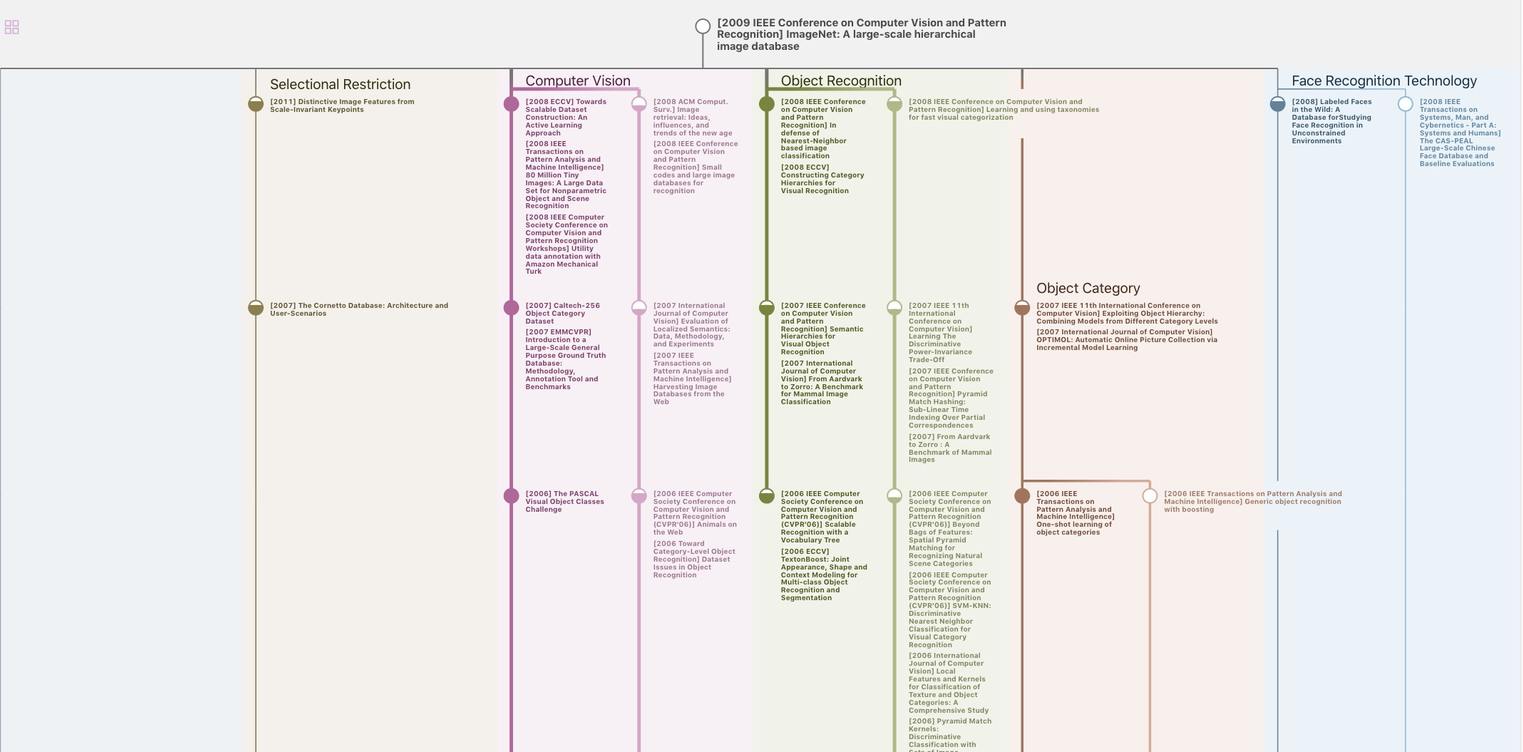
生成溯源树,研究论文发展脉络
Chat Paper
正在生成论文摘要