Data-driven model predictive control for ships with Gaussian process
Ocean Engineering(2023)
摘要
Nonlinear and underactuated ship maneuvering model is the main difficulty in ship motion control, and model predictive control (MPC) offers a great choice to handle this problem. However, most of the research on MPC adopts traditional parametric models based on physical prior knowledge, which limits its control performance and further application for various USVs. Recent success of machine learning has aroused a growing interest in data-driven and learning-based controller. Here, we present a data-driven ship heading controller, which com-bines the Gaussian process (GP) and MPC. The proposed method can identify the ship maneuvering model without any prior knowledge, and realize the heading control and waypoints following based on the learned nonparametric model. The similarity-based sparse GP algorithm is presented to reduce the computational complexity. Evaluated by both heading control and waypoints following in a real container ship data-driven simulation under environmental disturbances, the developed scheme is a powerful data-driven controller with good generalization ability and robustness to unknown disturbance.
更多查看译文
关键词
Model predictive control,System identification,Gaussian process,Ship maneuvering model
AI 理解论文
溯源树
样例
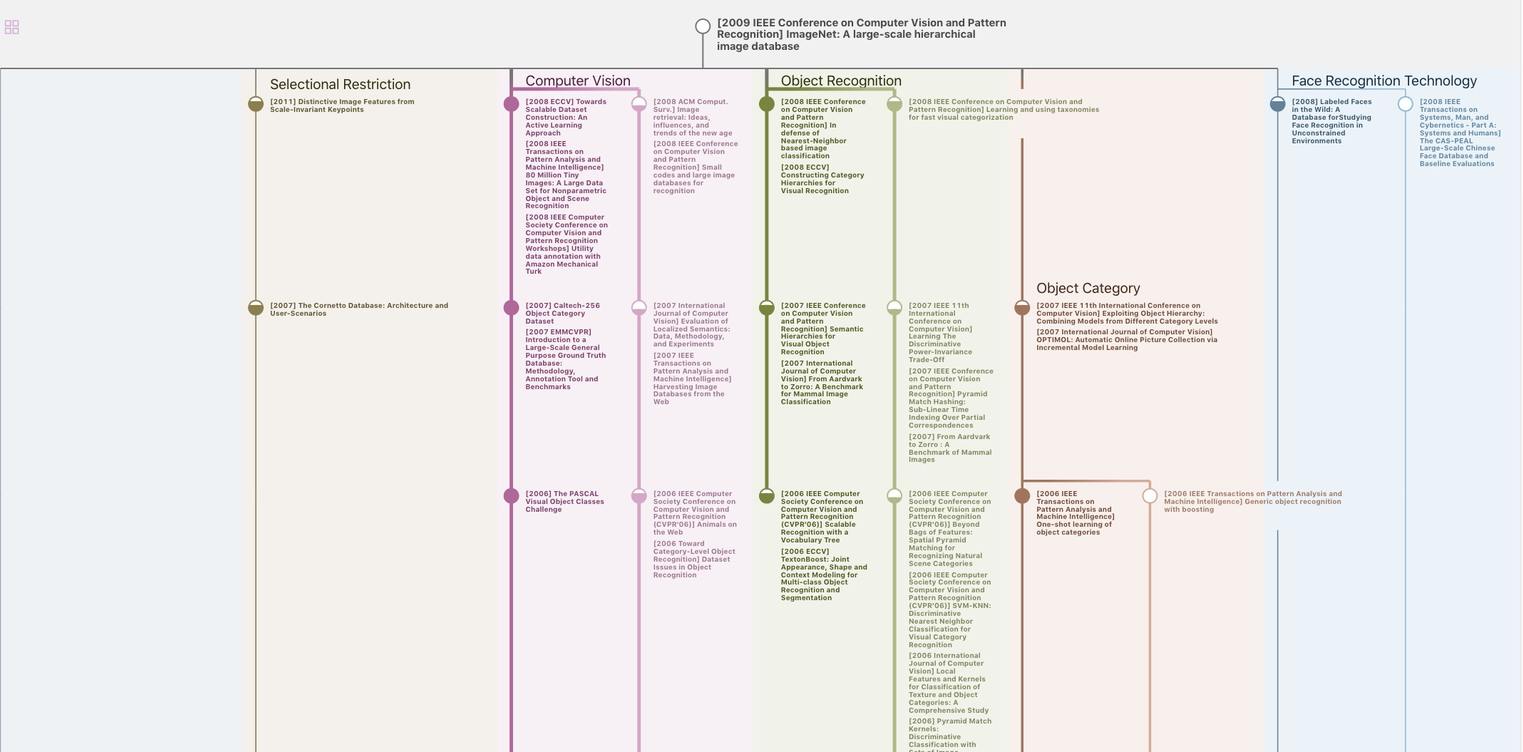
生成溯源树,研究论文发展脉络
Chat Paper
正在生成论文摘要