Intelligent air defense task assignment based on hierarchical reinforcement learning.
Frontiers in neurorobotics(2022)
摘要
Modern air defense battlefield situations are complex and varied, requiring high-speed computing capabilities and real-time situational processing for task assignment. Current methods struggle to balance the quality and speed of assignment strategies. This paper proposes a hierarchical reinforcement learning architecture for ground-to-air confrontation (HRL-GC) and an algorithm combining model predictive control with proximal policy optimization (MPC-PPO), which effectively combines the advantages of centralized and distributed approaches. To improve training efficiency while ensuring the quality of the final decision. In a large-scale area air defense scenario, this paper validates the effectiveness and superiority of the HRL-GC architecture and MPC-PPO algorithm, proving that the method can meet the needs of large-scale air defense task assignment in terms of quality and speed.
更多查看译文
关键词
agent,air defense task assignment,hierarchical reinforcement learning,model predictive control,proximal policy optimization
AI 理解论文
溯源树
样例
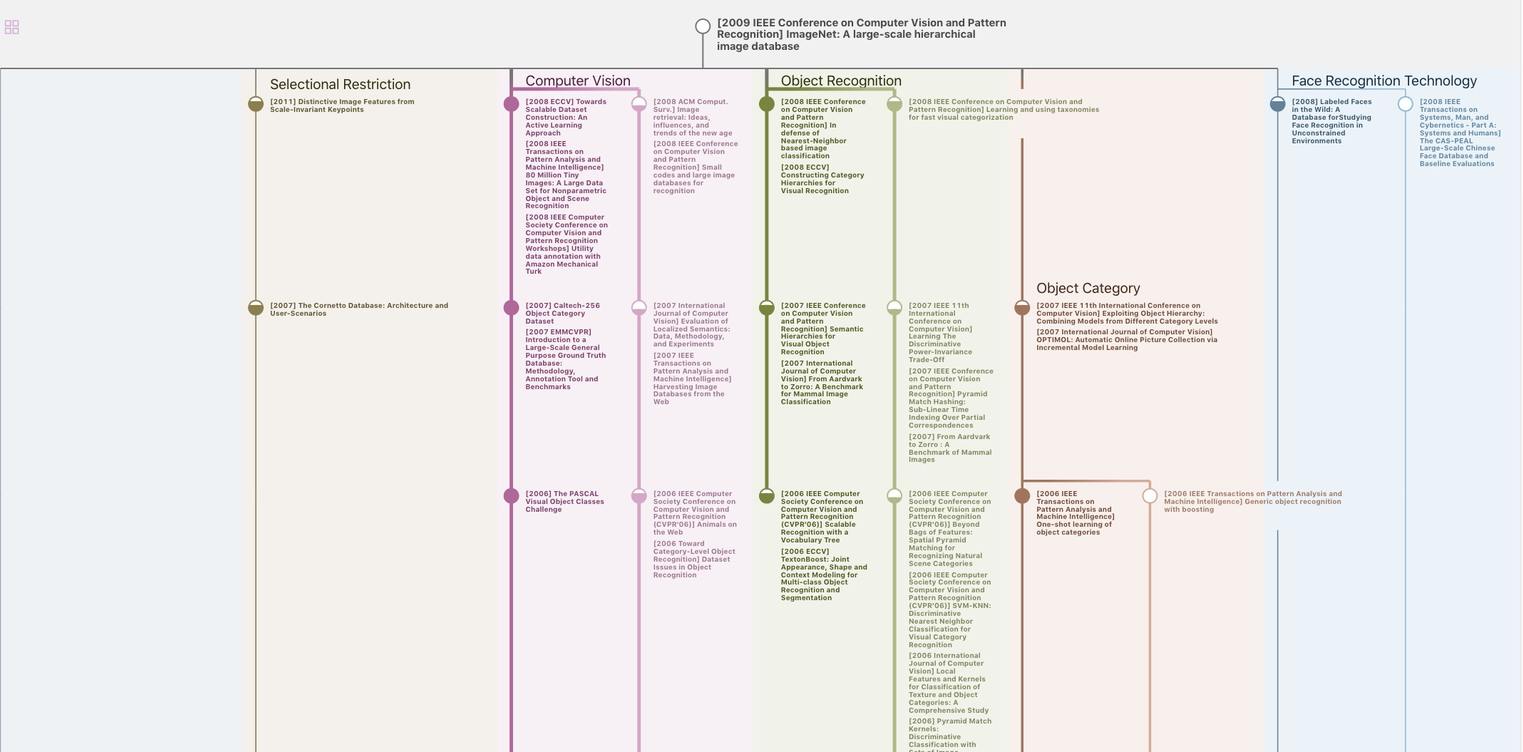
生成溯源树,研究论文发展脉络
Chat Paper
正在生成论文摘要