Deep learning model for diagnosing early gastric cancer using preoperative computed tomography images
FRONTIERS IN ONCOLOGY(2022)
摘要
BackgroundEarly gastric cancer (EGC) is defined as a lesion restricted to the mucosa or submucosa, independent of size or evidence of regional lymph node metastases. Although computed tomography (CT) is the main technique for determining the stage of gastric cancer (GC), the accuracy of CT for determining tumor invasion of EGC was still unsatisfactory by radiologists. In this research, we attempted to construct an AI model to discriminate EGC in portal venous phase CT images. MethodsWe retrospectively collected 658 GC patients from the first affiliated hospital of Nanchang university, and divided them into training and internal validation cohorts with a ratio of 8:2. As the external validation cohort, 93 GC patients were recruited from the second affiliated hospital of Soochow university. We developed several prediction models based on various convolutional neural networks, and compared their predictive performance. ResultsThe deep learning model based on the ResNet101 neural network represented sufficient discrimination of EGC. In two validation cohorts, the areas under the curves (AUCs) for the receiver operating characteristic (ROC) curves were 0.993 (95% CI: 0.984-1.000) and 0.968 (95% CI: 0.935-1.000), respectively, and the accuracy was 0.946 and 0.914. Additionally, the deep learning model can also differentiate between mucosa and submucosa tumors of EGC. ConclusionsThese results suggested that deep learning classifiers have the potential to be used as a screening tool for EGC, which is crucial in the individualized treatment of EGC patients.
更多查看译文
关键词
early gastric cancer,deep learning,computed tomography,convolutional neural,diagnosis
AI 理解论文
溯源树
样例
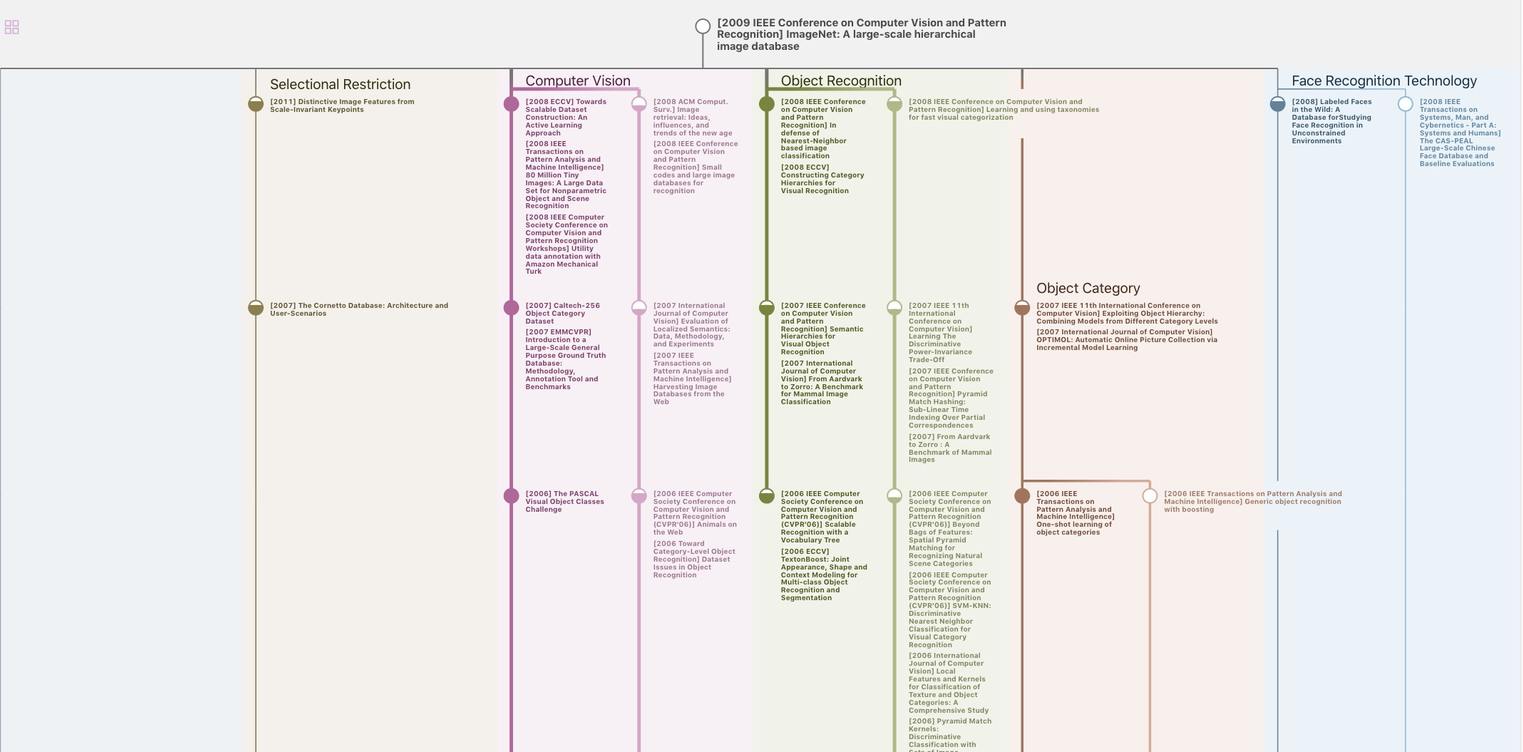
生成溯源树,研究论文发展脉络
Chat Paper
正在生成论文摘要