Reinforcement Learning-Based Underwater Acoustic Channel Tracking with Forgetting Factors
OCEANS 2022, Hampton Roads(2022)
摘要
Since the underwater acoustic (UWA) channels are usually correlated, subspace methods are studied for channel tracking. In these methods, the UWA channels can be reconstructed with the reduced-rank uncorrelated subspace and the corresponding channel principal components. A priori knowledge of channel characteristics is incorporated in the form of an autoregressive (AR) model for the channel principal components. However, the model mismatch is inevitable due to the challenging time-varying UWA channels. Reinforcement learning (RL) is an area of machine learning concerned with how intelligent agents ought to take actions in an environment in order to maximize the notion of cumulative reward. In this work, we explore RL-based channel tracking in UWA environments. We introduced a variable forgetting factor into forward-backward Kalman filter, and an RL agent to decide the parameter for the forgetting factor. Experimental results show that proposed channel tracking approaches have around 10 dB lower prediction error in calm sea and 5 dB lower prediction error in rough sea than conventional subspace channel tracking algorithms with the same at-sea data.
更多查看译文
关键词
Underwater acoustic channels, correlated channels, time-varying channels, reinforcement learning, forward-backward Kalman filter
AI 理解论文
溯源树
样例
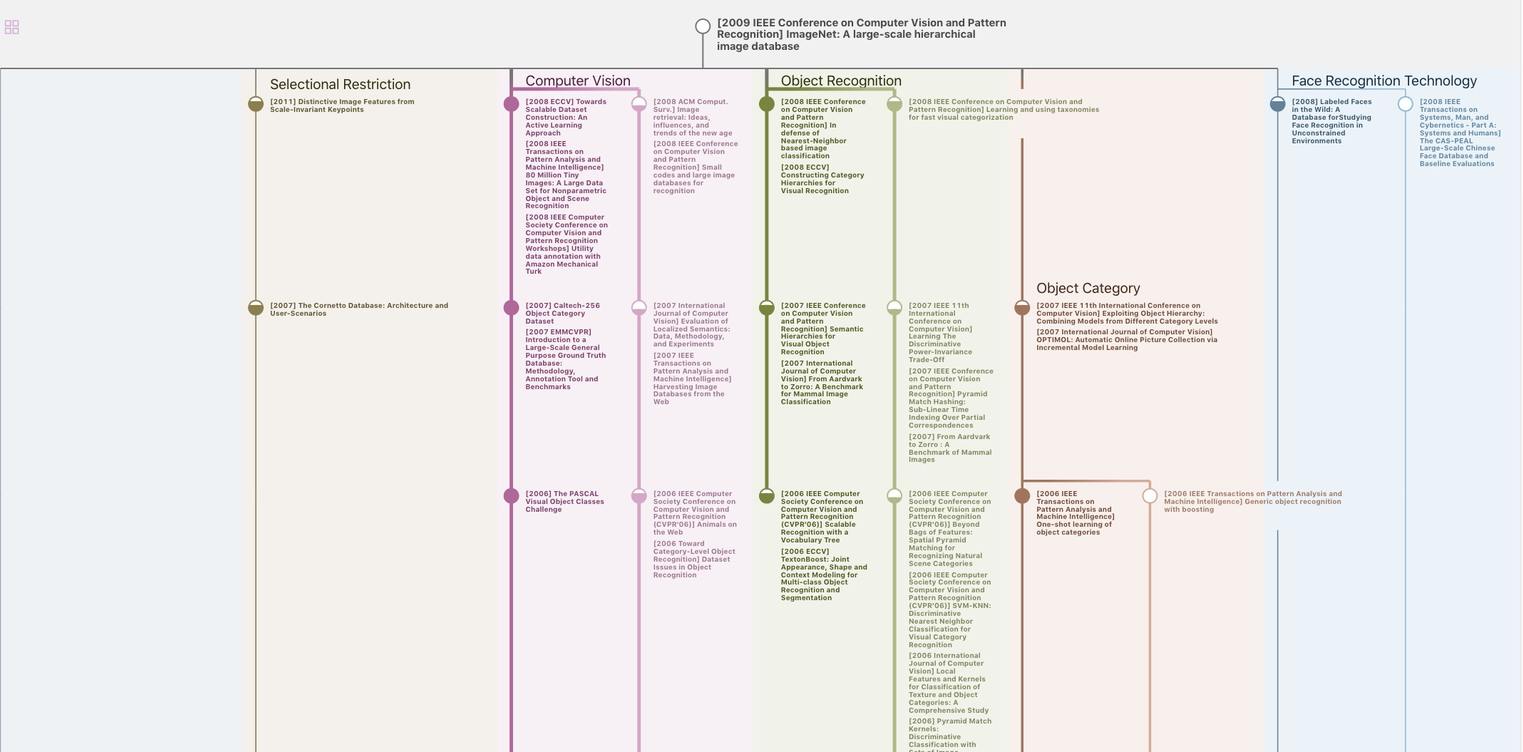
生成溯源树,研究论文发展脉络
Chat Paper
正在生成论文摘要