Unnatural Instructions: Tuning Language Models with (Almost) No Human Labor.
CoRR(2022)
Abstract
Instruction tuning enables pretrained language models to perform new tasks from inferencetime natural language descriptions. These approaches rely on vast amounts of human supervision in the form of crowdsourced datasets or user interactions. In this work, we introduce Unnatural Instructions: a large dataset of creative and diverse instructions, collected with virtually no human labor. We collect 64,000 examples by prompting a language model with three seed examples of instructions and eliciting a fourth. This set is then expanded by prompting the model to rephrase each instruction, creating a total of approximately 240,000 examples of instructions, inputs, and outputs. Experiments show that despite containing a fair amount of noise, training on Unnatural Instructions rivals the effectiveness of training on open-source manually-curated datasets, surpassing the performance of models such as T0++ and Tk-Instruct across various benchmarks. These results demonstrate the potential of model-generated data as a cost-effective alternative to crowdsourcing for dataset expansion and diversification.
MoreTranslated text
AI Read Science
Must-Reading Tree
Example
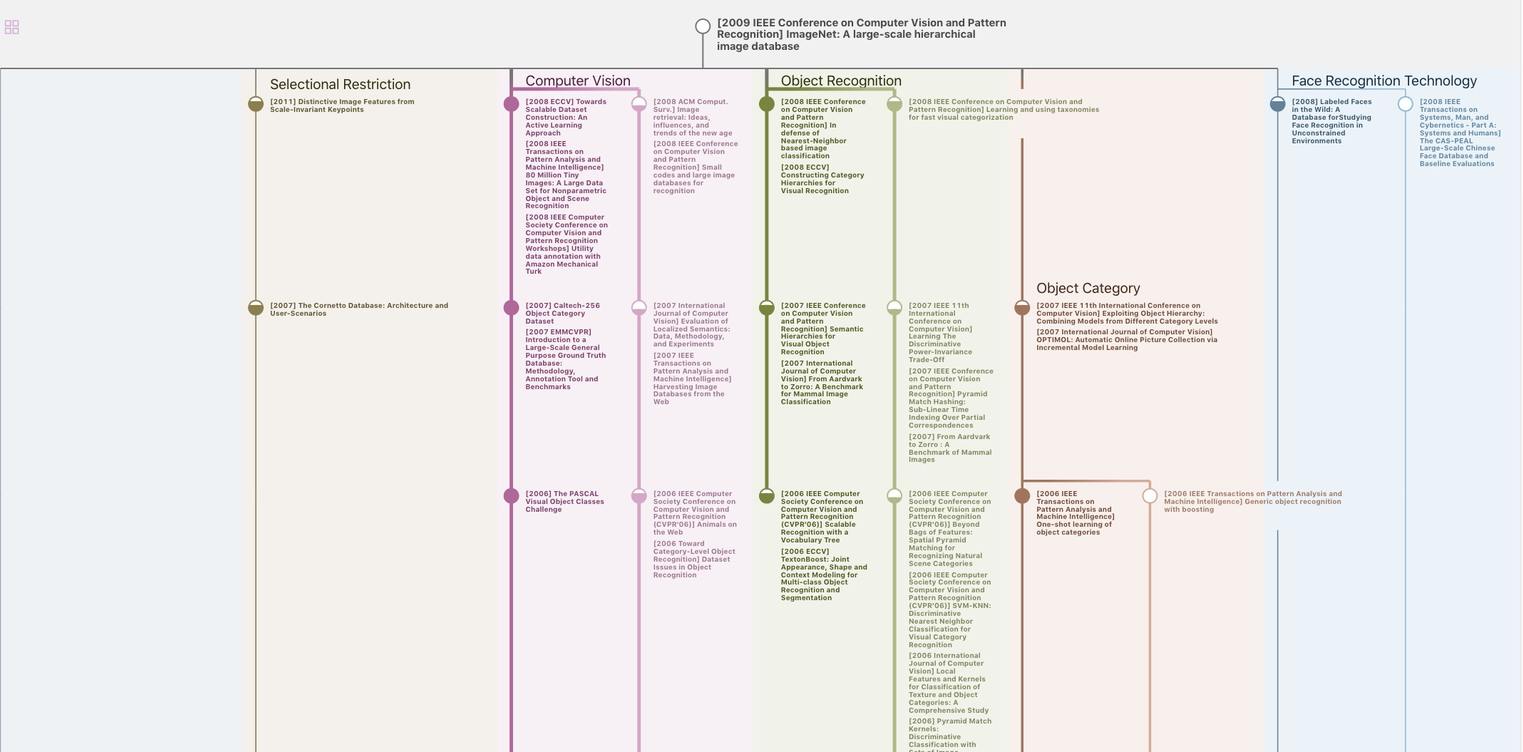
Generate MRT to find the research sequence of this paper
Chat Paper
Summary is being generated by the instructions you defined