High-Performance Filters For GPUs
arxiv(2022)
摘要
Filters approximately store a set of items while trading off accuracy for space-efficiency and can address the limited memory on accelerators, such as GPUs. However, there is a lack of high-performance and feature-rich GPU filters as most advancements in filter research has focused on CPUs. In this paper, we explore the design space of filters with a goal to develop massively parallel, high performance, and feature rich filters for GPUs. We evaluate various filter designs in terms of performance, usability, and supported features and identify two filter designs that offer the right trade off in terms of performance, features, and usability. We present two new GPU-based filters, the TCF and GQF, that can be employed in various high performance data analytics applications. The TCF is a set membership filter and supports faster inserts and queries, whereas the GQF supports counting which comes at an additional performance cost. Both the GQF and TCF provide point and bulk insertion API and are designed to exploit the massive parallelism in the GPU without sacrificing usability and necessary features. The TCF and GQF are up to $4.4\times$ and $1.4\times$ faster than the previous GPU filters in our benchmarks and at the same time overcome the fundamental constraints in performance and usability in current GPU filters.
更多查看译文
关键词
gpus,high-performance
AI 理解论文
溯源树
样例
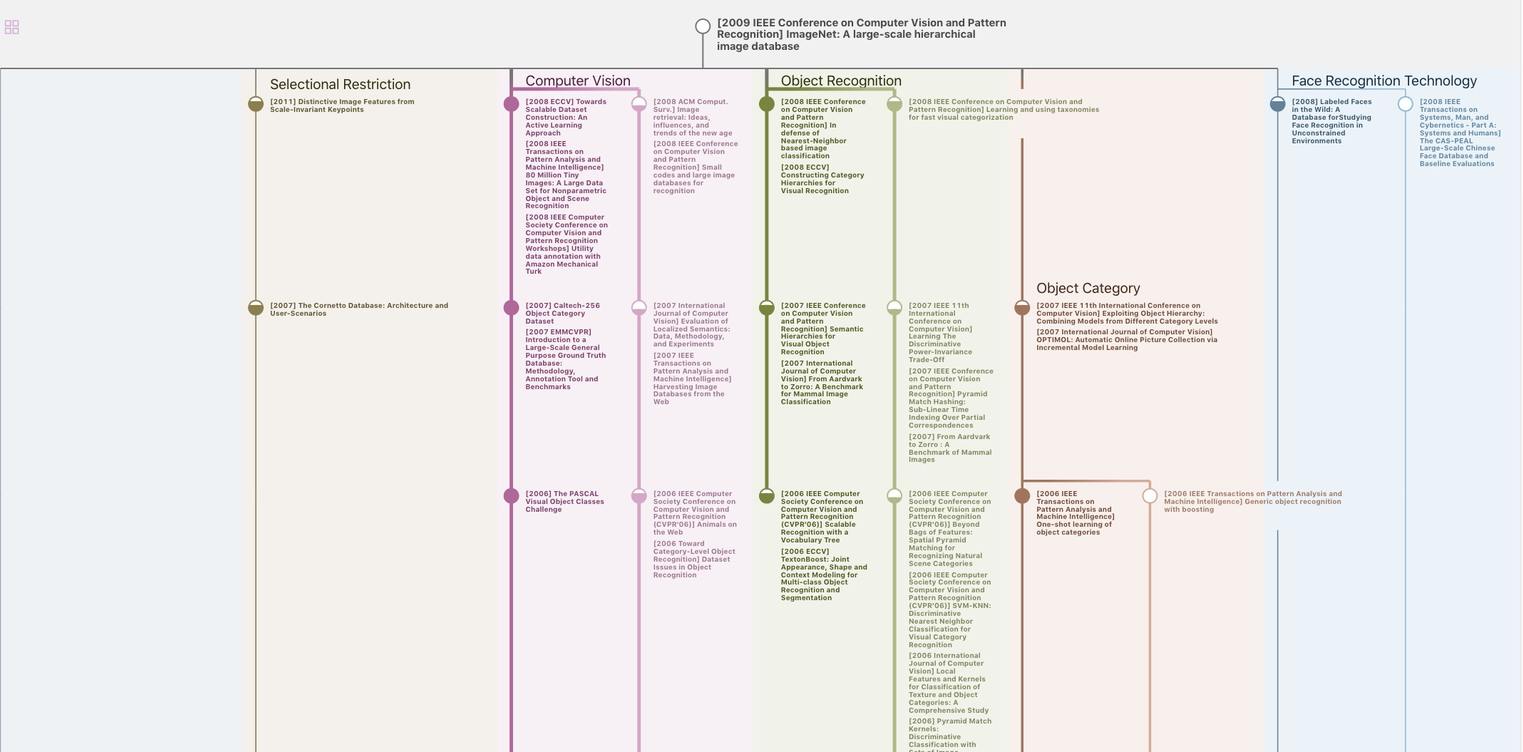
生成溯源树,研究论文发展脉络
Chat Paper
正在生成论文摘要