Penalized regression with multiple sources of prior effects
BIOINFORMATICS(2023)
摘要
Motivation In many high-dimensional prediction or classification tasks, complementary data on the features are available, e.g. prior biological knowledge on (epi)genetic markers. Here we consider tasks with numerical prior information that provide an insight into the importance (weight) and the direction (sign) of the feature effects, e.g. regression coefficients from previous studies.Results We propose an approach for integrating multiple sources of such prior information into penalized regression. If suitable co-data are available, this improves the predictive performance, as shown by simulation and application.Availability and implementation The proposed method is implemented in the R package transreg (https://github.com/lcsb-bds/transreg, https://cran.r-project.org/package=transreg).
更多查看译文
AI 理解论文
溯源树
样例
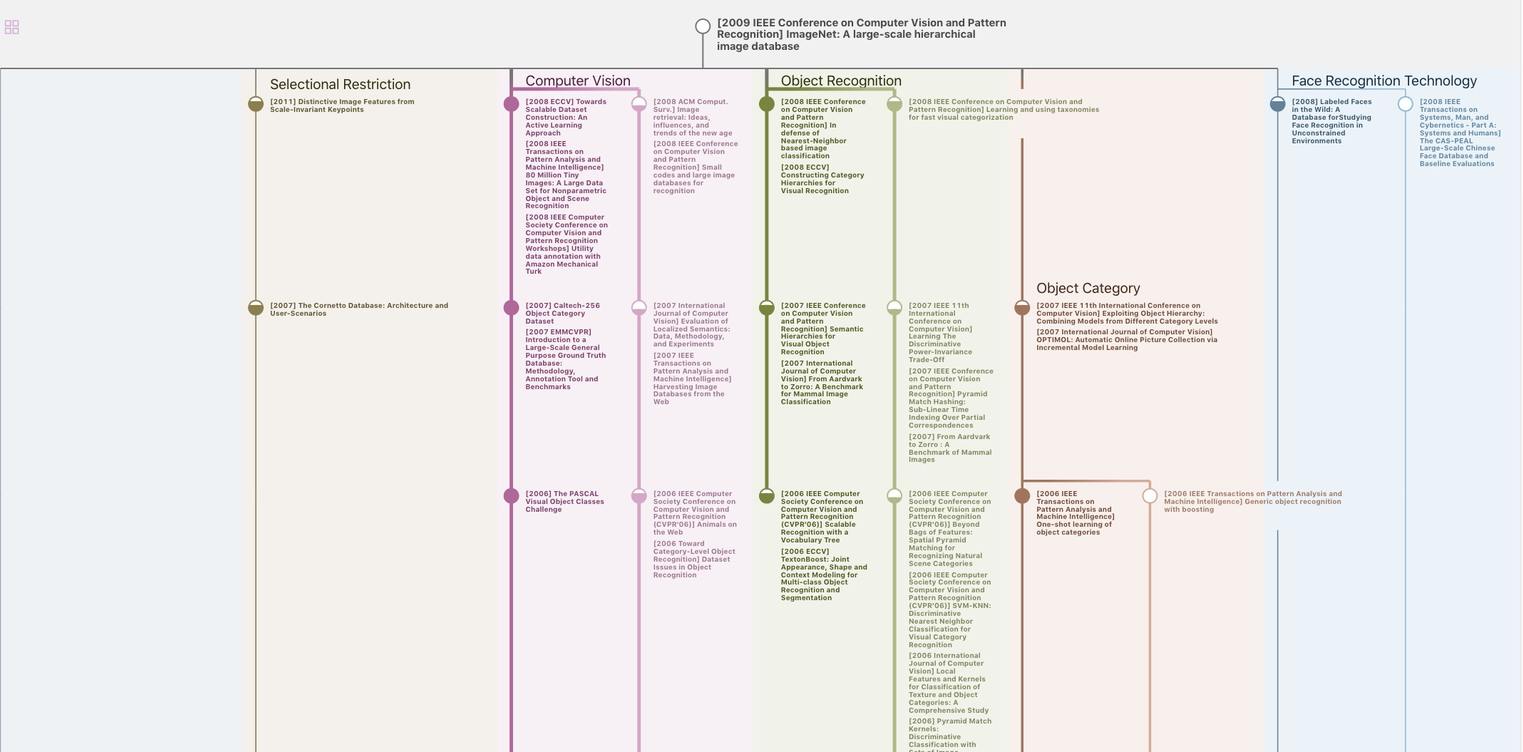
生成溯源树,研究论文发展脉络
Chat Paper
正在生成论文摘要