Evaluation of Synthetic Datasets for Conversational Recommender Systems
CoRR(2022)
Abstract
For researchers leveraging Large-Language Models (LLMs) in the generation of training datasets, especially for conversational recommender systems - the absence of robust evaluation frameworks has been a long-standing problem. The efficiency brought about by LLMs in the data generation phase is impeded during the process of evaluation of the generated data, since it generally requires human-raters to ensure that the data generated is of high quality and has sufficient diversity. Since the quality of training data is critical for downstream applications, it is important to develop metrics that evaluate the quality holistically and identify biases. In this paper, we present a framework that takes a multi-faceted approach towards evaluating datasets produced by generative models and discuss the advantages and limitations of various evaluation methods.
MoreTranslated text
Key words
synthetic datasets
AI Read Science
Must-Reading Tree
Example
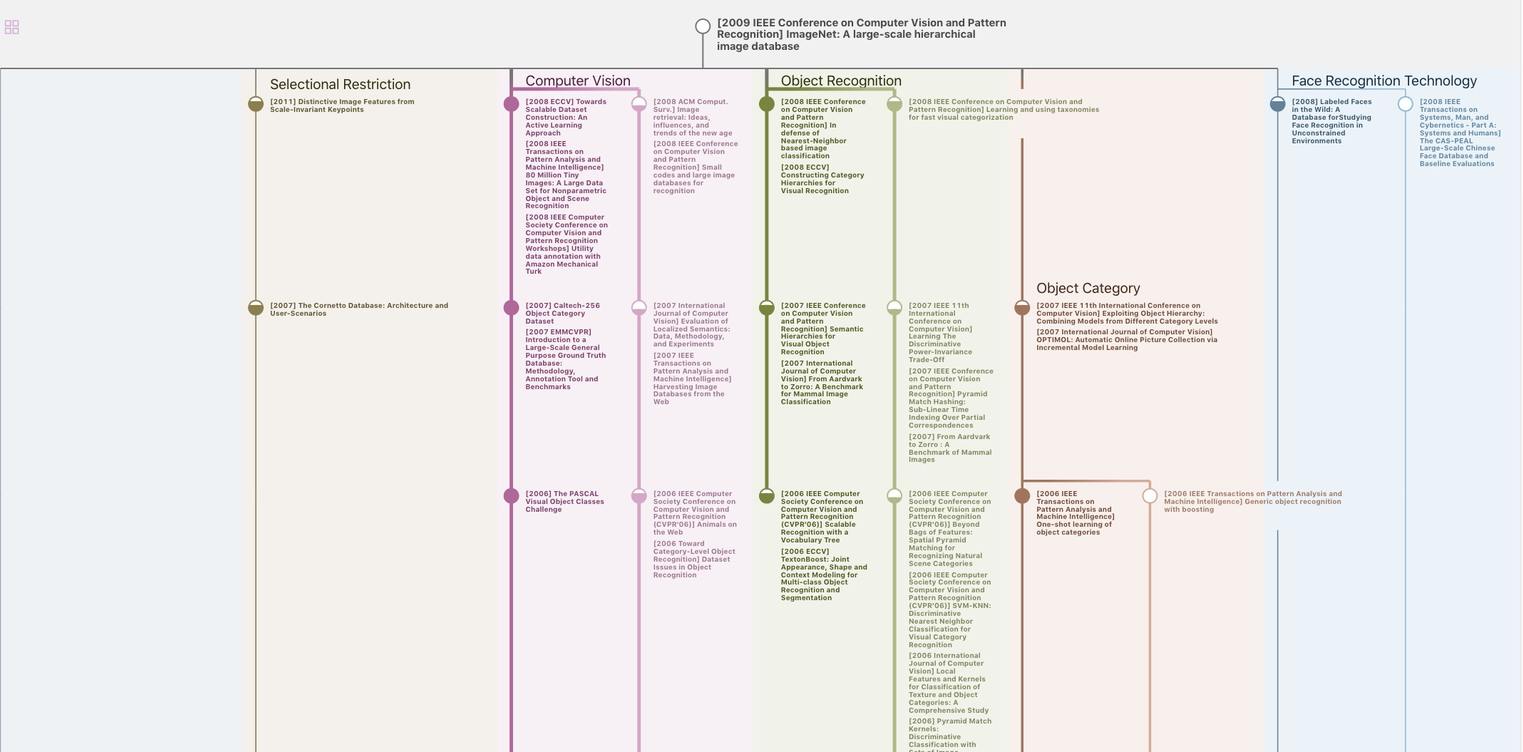
Generate MRT to find the research sequence of this paper
Chat Paper
Summary is being generated by the instructions you defined