Indirect measurement of cutting forces during robotic milling using multiple sensors and a machine learning-based system identifier
Journal of Manufacturing Processes(2023)
Abstract
Robotic machining has attracted great interest in the fields of aerospace and automotive manufacturing because of its advantages over conventional computer numerical controlled (CNC) machine tools; robotic machining is flexible and can fabricate components with complex (sculpted) geometries and of large dimensions. The principal challenge, however, is the poor structural rigidity of robots, leading to high-level vibration and chatter during machining. The cutting force must be monitored to detect and suppress chatter as well as optimize machining. A practical method is required to measure the cutting force during machining without impeding robot movement. This study presents an indirect method that accurately measures the milling force using an accelerometer located on the spindle and a flange-mounted capacitive force sensor. A Kalman filter was used to compensate for the dynamics between the robot tool center point (TCP) and the location of the accelerometer. In the context of pose-dependent modeling of the dynamics of the robot, a novel machine-learning (ML)-based identifier is presented that rapidly identifies the relevant dynamics (i.e., the modal parameters) without any initial human guesswork. Data from dynamic simulations and experiments were used to train and validate the ML-based identifier. The validation results showed that the identifier rapidly predicted the behaviour of physical systems with an average accuracy of 98.49 % compared to the modal analysis method. Next, the cutting forces were indirectly measured using the acceleration signals and a Kalman filter, developed based on the modal parameters. The forces measured by the proposed method were in good agreement with the reference forces, measured using a table dynamometer; the average peak-to-peak accuracy was 90.17 % for the AC component of the cutting force, and the average time-domain accuracy was 95.27 % when the DC component was also considered.
MoreTranslated text
AI Read Science
Must-Reading Tree
Example
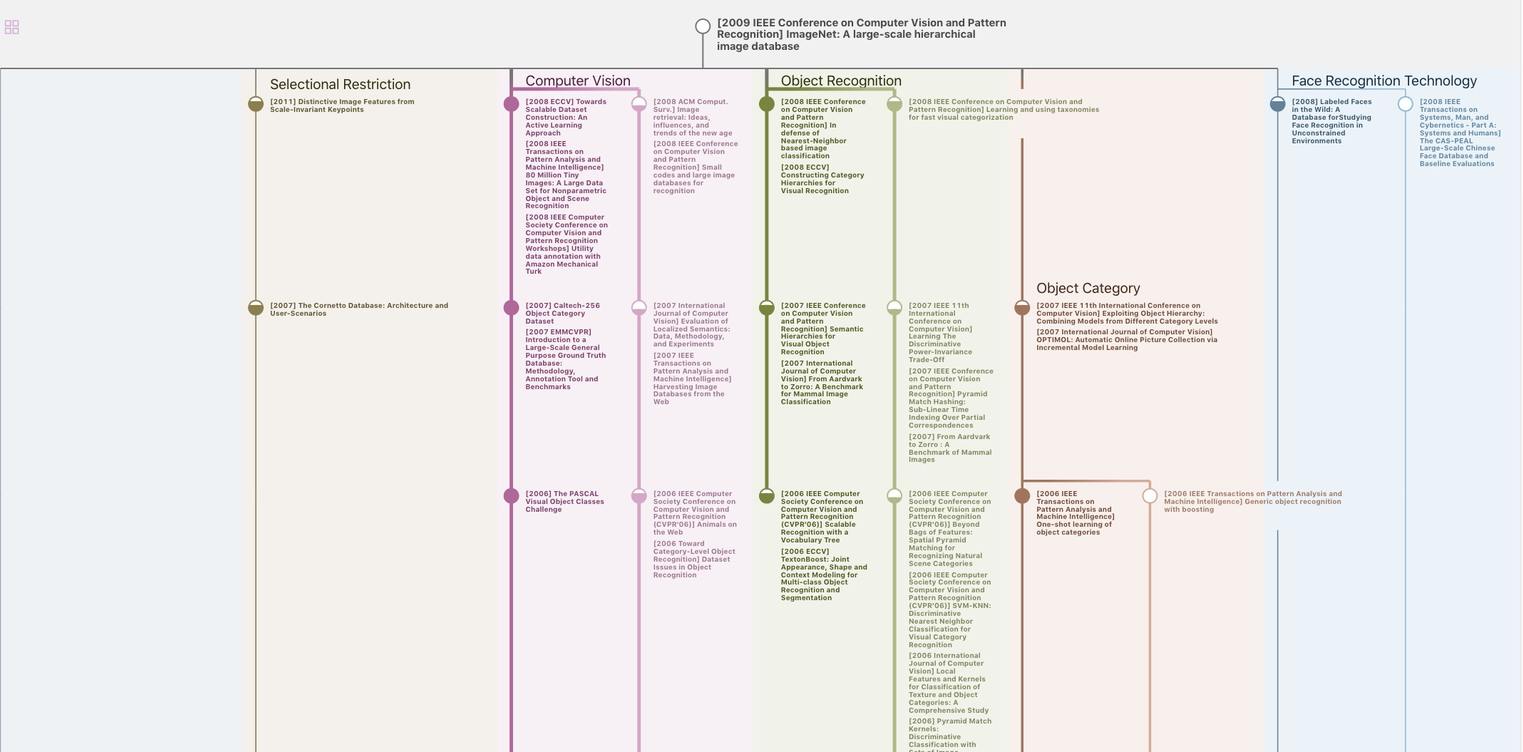
Generate MRT to find the research sequence of this paper
Chat Paper
Summary is being generated by the instructions you defined