Unconstrained generation of synthetic antibody–antigen structures to guide machine learning methodology for antibody specificity prediction
Nature Computational Science(2022)
摘要
Machine learning (ML) is a key technology for accurate prediction of antibody–antigen binding. Two orthogonal problems hinder the application of ML to antibody-specificity prediction and the benchmarking thereof: the lack of a unified ML formalization of immunological antibody-specificity prediction problems and the unavailability of large-scale synthetic datasets to benchmark real-world relevant ML methods and dataset design. Here we developed the Absolut! software suite that enables parameter-based unconstrained generation of synthetic lattice-based three-dimensional antibody–antigen-binding structures with ground-truth access to conformational paratope, epitope and affinity. We formalized common immunological antibody-specificity prediction problems as ML tasks and confirmed that for both sequence- and structure-based tasks, accuracy-based rankings of ML methods trained on experimental data hold for ML methods trained on Absolut!-generated data. The Absolut! framework has the potential to enable real-world relevant development and benchmarking of ML strategies for biotherapeutics design. The Absolut! framework can generate synthetic three-dimensional antibody–antigen structures to assist machine learning and dataset construction for antibody design. Most importantly, the relative machine learning performance learnt on Absolut! datasets is shown to transfer to experimental datasets.
更多查看译文
关键词
Antibodies,Machine learning,Software,Computer Science,general
AI 理解论文
溯源树
样例
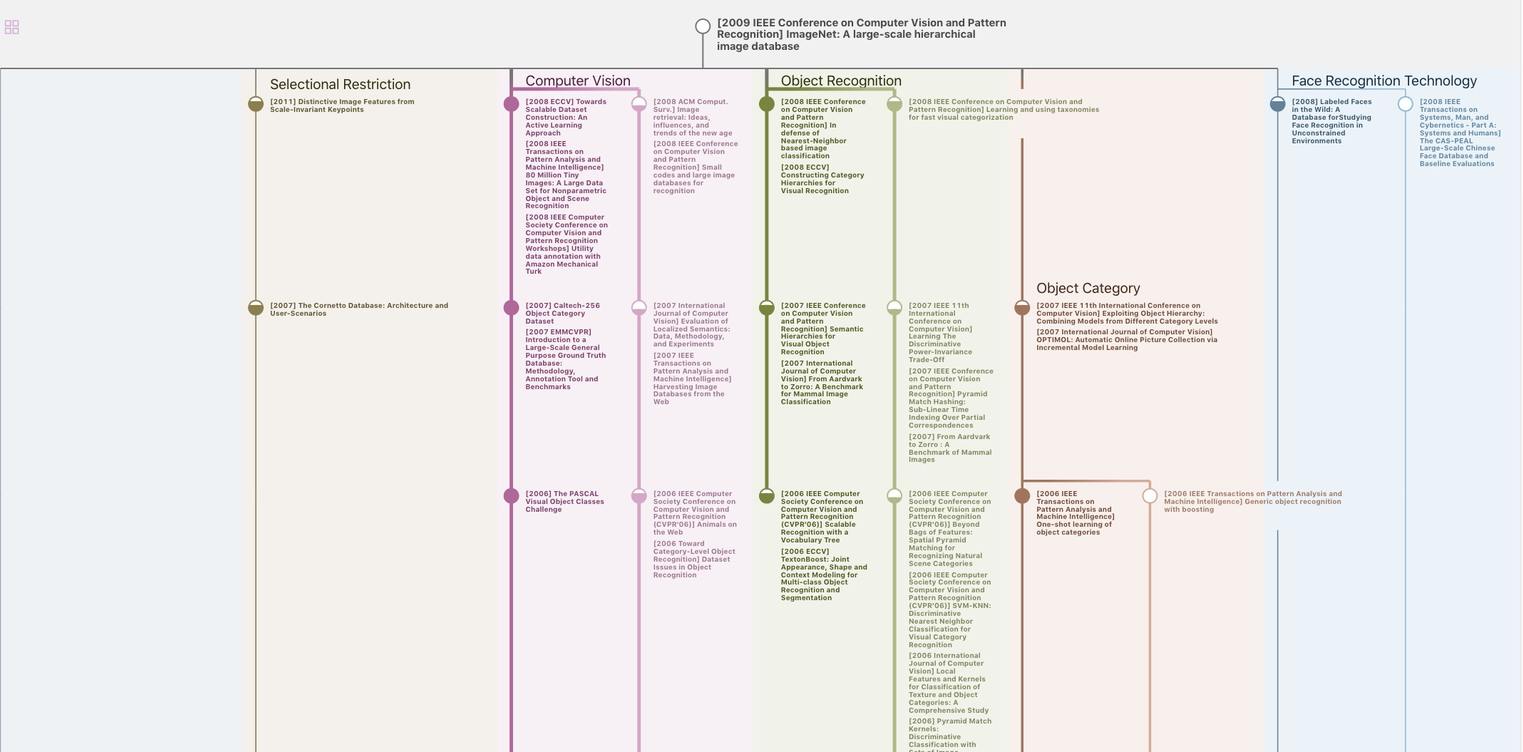
生成溯源树,研究论文发展脉络
Chat Paper
正在生成论文摘要