Reinforcement learning-driven deep question generation with rich semantics
INFORMATION PROCESSING & MANAGEMENT(2023)
摘要
Deep question generation (DQG) refers to generating a complex question from different sen-tences in context. Existing methods mainly focus on enhancing information extraction based on the encoder-decoder neural networks though they cannot perform well in DQG tasks. To address this issue, we consider combining reinforcement learning with semantic-rich information to generate deep questions in this paper. In particular, we propose a Semantic-Rich Reinforcement Learning Deep Question Generation (SRL-DQG) model, which better utilizes the semantic graphs of document representations based on the Gated Graph Neural Network (GGNN). In order to generate high-quality questions, we also optimize specific objectives via reinforcement learning with consideration of four evaluation factors including naturality, relevance, answerability, and difficulty. Empirical evaluations demonstrate that our SRL-DQG effectively improves the quality of generated questions and achieves superior performance than existing methods in terms of multiple performance metrics. Specifically, we show that several BLEU -n scores were improved by 3.5% to 10% after running SRL-DQG on 6072 samples of HotPotQA.
更多查看译文
关键词
Deep question generation,Reinforcement learning,Semantic graphs,Gated Graph Neural Network
AI 理解论文
溯源树
样例
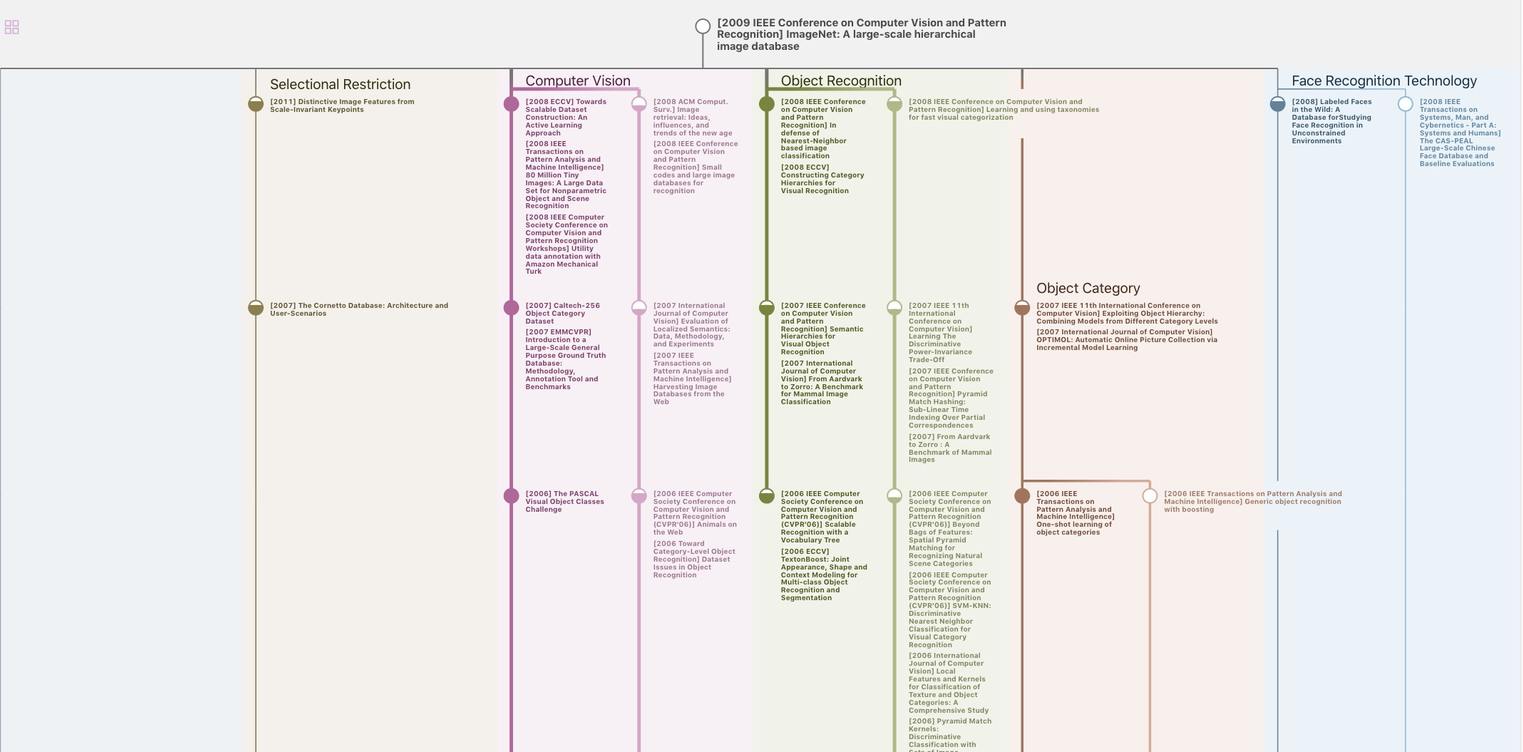
生成溯源树,研究论文发展脉络
Chat Paper
正在生成论文摘要