Joint grading of diabetic retinopathy and diabetic macular edema using an adaptive attention block and semisupervised learning
APPLIED INTELLIGENCE(2022)
摘要
The early screening and treatment of diabetic retinopathy (DR) and diabetic macular edema (DME) can prevent the risk of blindness for most diabetic patients. Joint grading of DR and DME can reduce the screening cost and improve screening efficiency. Most grading methods only focus on grading one disease and lack generality. In this paper, we propose an adaptive attention block (AAB) to better extract features and improve model performance, which can be adaptively adjusted according to different grading tasks. We propose the AABNet to handle multiple grading tasks, consisting of a lightweight architecture MobileNetv2 as a backbone for feature extraction and two independent branches, the DR branch and the DME branch, for grading prediction. To alleviate the lack of labeled data, we propose an adaptive teacher-student model as a semisupervised learning method to train the AABNet with additional unlabeled data, dependent on fewer model parameters, whose foundation is consistency regularization, which is added to the loss to provide additional supervised signals for training to obtain a more accurate model without destroying the image details and strictly requiring the quality of unlabeled images. Extensive experiments are conducted, including performance comparisons with state-of-the-art methods and ablation studies of attention mechanisms and semisupervised learning mechanisms. Experiments verify that AABNet can bring a satisfactory improvement in the multiple DR grading, DME grading and joint DR&DME grading tasks. AABNet outperforms other state-of-the-art methods and achieves better results in multiple grading tasks on the Messidor and DDR datasets.
更多查看译文
关键词
Diabetic retinopathy grading,Diabetic macular edema grading,Attention mechanism,Semisupervised learning
AI 理解论文
溯源树
样例
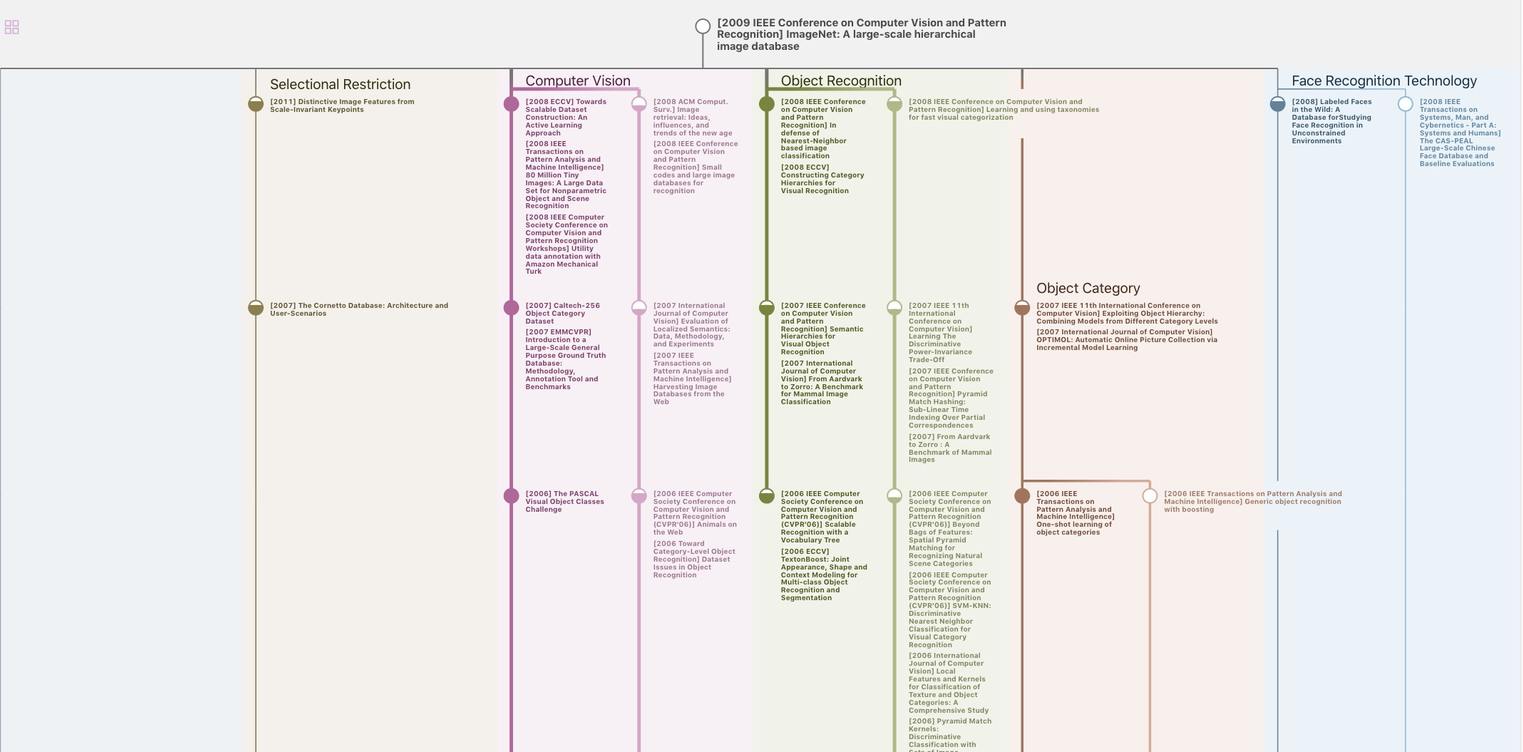
生成溯源树,研究论文发展脉络
Chat Paper
正在生成论文摘要