Parallel Learning of Dynamics in Complex Systems
Systems(2022)
Abstract
Dynamics always exist in complex systems. Graphs (complex networks) are a mathematical form for describing a complex system abstractly. Dynamics can be learned efficiently from the structure and dynamics state of a graph. Learning the dynamics in graphs plays an important role in predicting and controlling complex systems. Most of the methods for learning dynamics in graphs run slowly in large graphs. The complexity of the large graph’s structure and its nonlinear dynamics aggravate this problem. To overcome these difficulties, we propose a general framework with two novel methods in this paper, the Dynamics-METIS (D-METIS) and the Partitioned Graph Neural Dynamics Learner (PGNDL). The general framework combines D-METIS and PGNDL to perform tasks for large graphs. D-METIS is a new algorithm that can partition a large graph into multiple subgraphs. D-METIS innovatively considers the dynamic changes in the graph. PGNDL is a new parallel model that consists of ordinary differential equation systems and graph neural networks (GNNs). It can quickly learn the dynamics of subgraphs in parallel. In this framework, D-METIS provides PGNDL with partitioned subgraphs, and PGNDL can solve the tasks of interpolation and extrapolation prediction. We exhibit the universality and superiority of our framework on four kinds of graphs with three kinds of dynamics through an experiment.
MoreTranslated text
Key words
complex systems,graph partition,graph neural networks,ordinary differential equation,dynamics
AI Read Science
Must-Reading Tree
Example
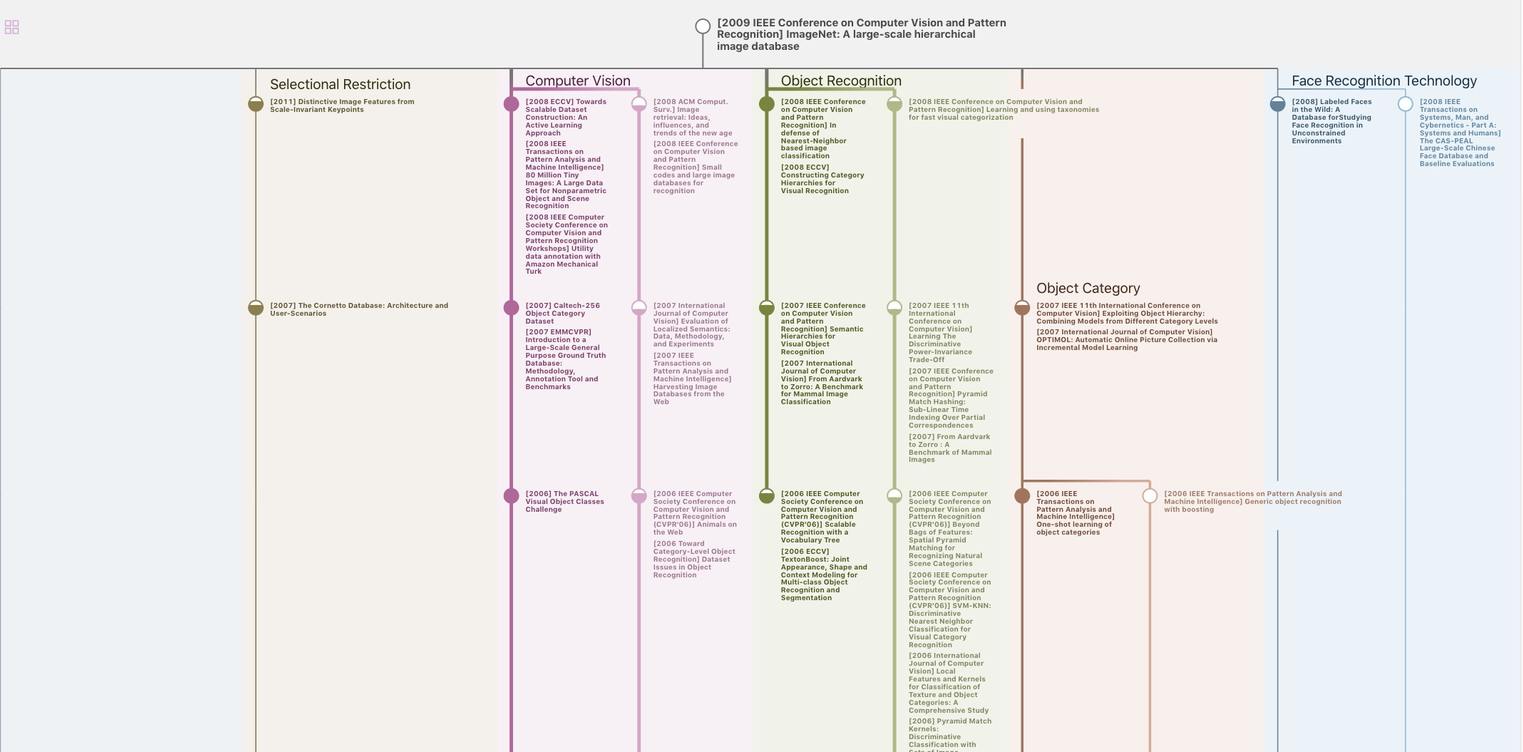
Generate MRT to find the research sequence of this paper
Chat Paper
Summary is being generated by the instructions you defined