Multiscale spectral modelling for nonstationary time series within an ordered multiple-trial experiment
ANNALS OF APPLIED STATISTICS(2022)
摘要
Within the neurosciences it is natural to observe variability across time in the dynamics of an underlying brain process. Wavelets are essential in analysing brain signals because, even within a single trial, brain signals exhibit nonstationary behaviour. However, neurological signals generated within an experiment may also potentially exhibit evolution across trials (replicates), even for identical stimuli. As neurologists consider localised spectra of brain signals to be most informative, we propose the MULtiple-Trials Locally Stationary Wavelet process (MULT-LSW) that fills the gap in the literature by directly giving a stochastic wavelet representation of the time series of ordered replicates itself. MULT-LSW yields a natural desired time-and trial-localisation of the process dynamics, capturing nonstation-ary behaviour both within and across trials. While current techniques are restricted by the assumption of uncorrelated replicates, here we account for between-trial correlation. We rigorously develop the associated wavelet spec-tral estimation framework along with its asymptotic properties. By means of thorough simulation studies, we demonstrate the theoretical estimator proper-ties hold in practice. A real data investigation into the evolutionary dynamics of the hippocampus and nucleus accumbens, during an associative learning experiment, demonstrates the applicability of our proposed methodology as well as the new insights it provides. Our model is general and facilitates wider experimental data analysis than the current literature allows.
更多查看译文
关键词
multiscale spectral modelling,nonstationary time series,multiple-trial
AI 理解论文
溯源树
样例
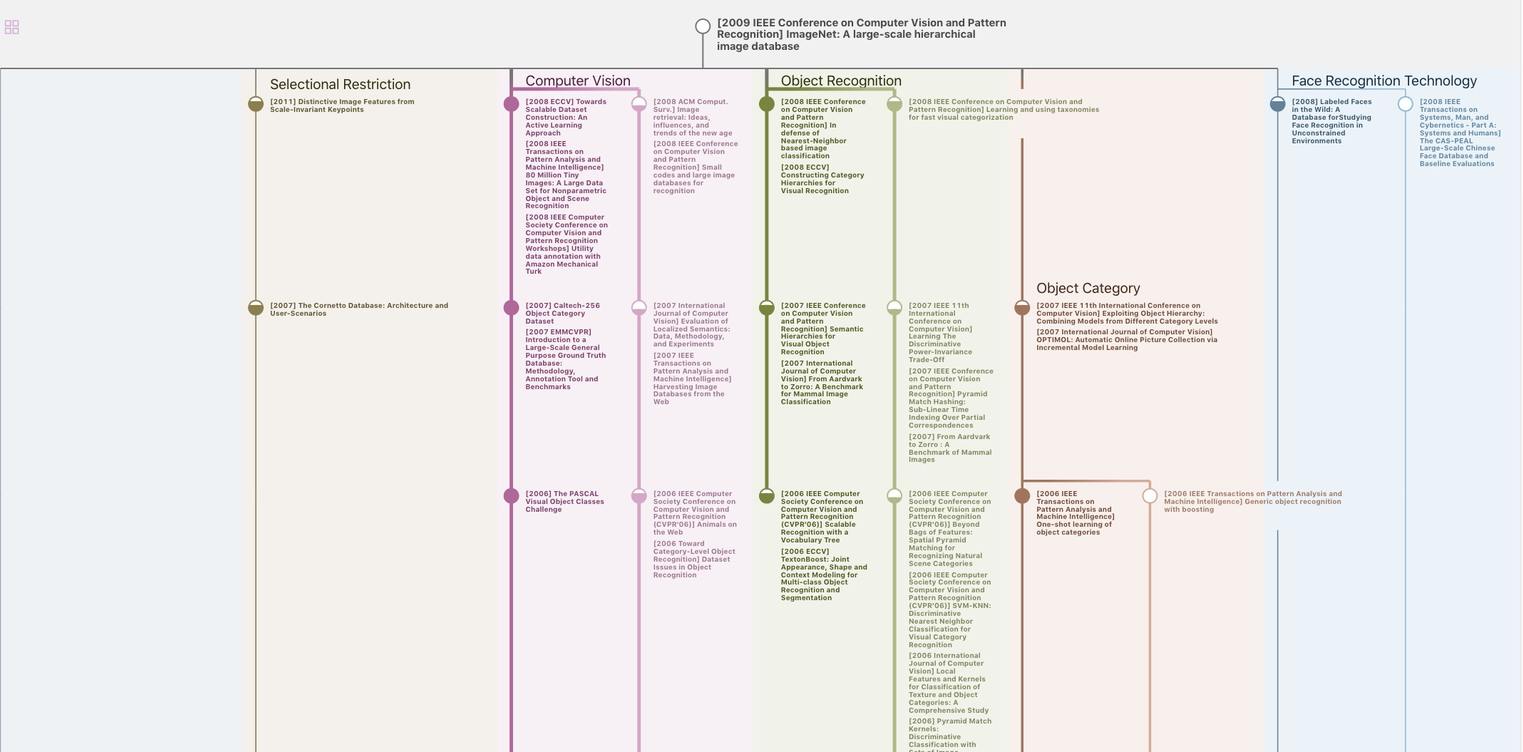
生成溯源树,研究论文发展脉络
Chat Paper
正在生成论文摘要