Machine Learning Based Intrusion Detection on Complex Nested Transactional SQL Queries
2022 IEEE NIGERIA 4TH INTERNATIONAL CONFERENCE ON DISRUPTIVE TECHNOLOGIES FOR SUSTAINABLE DEVELOPMENT (IEEE NIGERCON)(2022)
摘要
for many private and government sectors, such as education, banks, and businesses, a database has been a vital source of information storage. Many academics have focused on security issues such as privacy and integrity because of the extensive use of this database on the cloud. With an Intrusion Detection System (IDS), researchers have proposed security measures to detect unusual database user behavior. However, in a recent study, role-based, nested queries within transactional SQL queries have not been put into consideration. This work proposes the Multiplet data structure for identifying anomalies in nested transactional SQL queries. These queries are translated into the presented data structure, which models the use of the user's profile, therefore, detects intruders. This Multiplet allows us to compare transaction-log with SQL statements given by the user in an attempt to improve detection accuracy. The algorithm detects and reports the suspected invader if there is no link between the usual user queries and the suspicious issued queries. The results from the Naive Bayes Classifier (NBC) showed that 97% accuracy of intrusion was effectively detected. Moreover, a complex transactional database that tends to deviate from the usual user SQL queries were detected, which signifies the usefulness contribution in this paper.
更多查看译文
关键词
Security,Intrusion,Detection,Integrity,Privacy
AI 理解论文
溯源树
样例
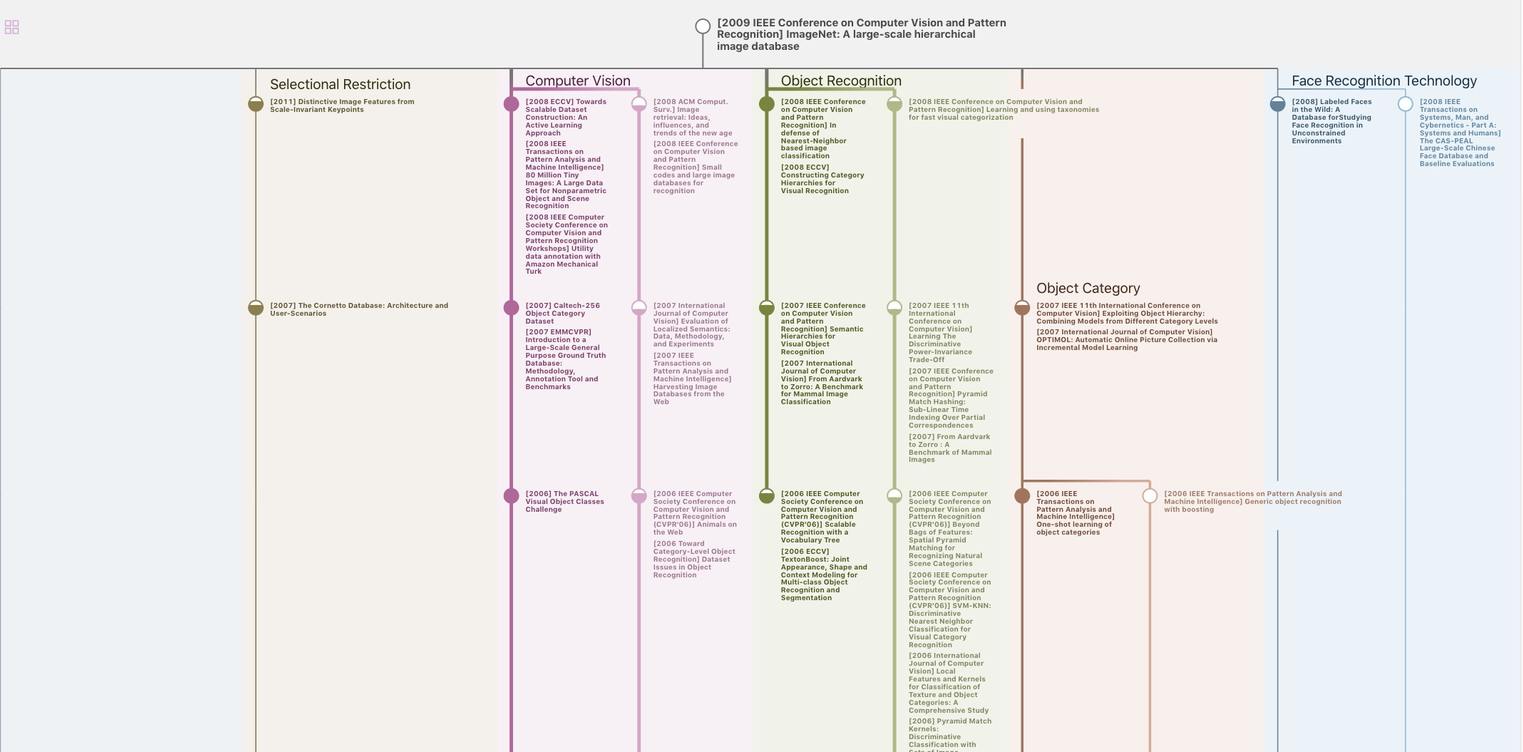
生成溯源树,研究论文发展脉络
Chat Paper
正在生成论文摘要