Performance Analysis of BFGS Quasi-Newton Neuro Algorithm for the Design of 30 GHz Patch Antenna for 5G Applications
2022 IEEE NIGERIA 4TH INTERNATIONAL CONFERENCE ON DISRUPTIVE TECHNOLOGIES FOR SUSTAINABLE DEVELOPMENT (IEEE NIGERCON)(2022)
摘要
Wireless communication systems have been driven towards Fifth Generation (5G) networks due to increasing demands for compact, high speed, and large bandwidth systems. These types of wireless communication systems require new and more efficient antenna designs. This article therefore researches into the radiation pattern and properties of one of such technologies - a 30 GHz microstrip antenna, using Broyden-Fletcher-Goldfarb-Shanno-trained Artificial Neural Network to analyze its radiation properties in line with the wireless communication design objectives for 5G applications. The overall performances thereof analyzed using standard performance metrics show that the Broyden-Fletcher-Goldfarb-Shanno (BFGS) neural network algorithm performed satisfactorily in rapidly analyzing the antenna's radiation properties. This result finds relevance in network planning of 5G networks and smart city designs.
更多查看译文
关键词
Microstrip antenna, 5G Networks, BFGS, Neural Network, Directive Gain
AI 理解论文
溯源树
样例
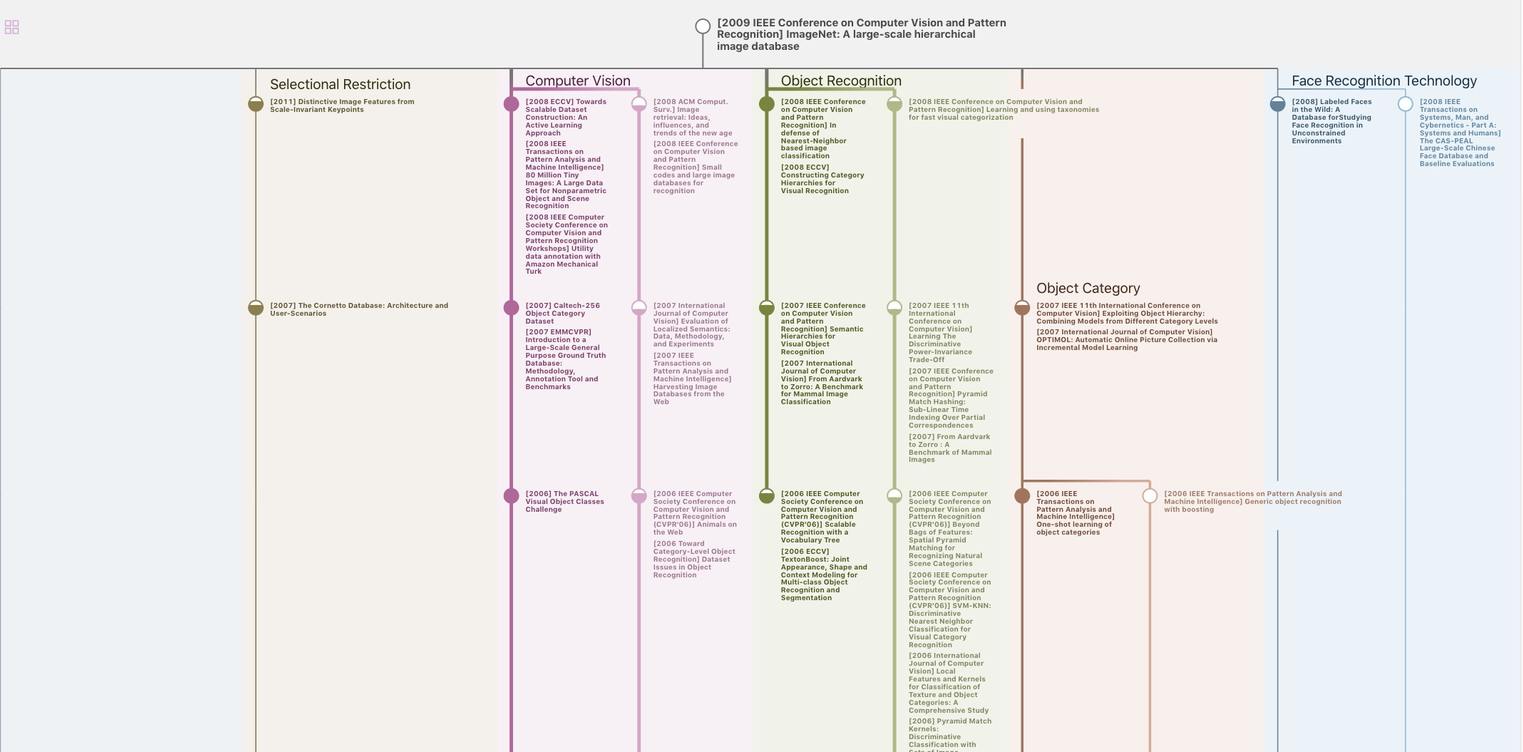
生成溯源树,研究论文发展脉络
Chat Paper
正在生成论文摘要