Efficient Meta label correction based on Meta Learning and bi-level optimization
ENGINEERING APPLICATIONS OF ARTIFICIAL INTELLIGENCE(2023)
Abstract
The design of highly accurate deep learning architectures is related to different parameters through different optimizations at different levels of the design. While the architectural design and the training procedure of the neural networks play significant role, the quantity and the quality of the available data remain also very important issues. This research aims to address the quality of the data issue: the data contain imprecise or noisy labels. Numerous approaches have been proposed in the literature to leverage noisy data in case of different noisy labels as well as the strategies and frameworks developed to use the data in practice. Recently, meta-learning based methods have shown good performance to optimize models that take into account the presence of noisy labels within the training dataset. In this work, we propose a new method called Meta label correction via Meta Learning and bi-level optimization (CO-META). It is based on extracting and providing only relevant features to enhance the performance of the label corrector network to tackle the raised problem. The obtained results demonstrate that the proposed method outperforms the state-of-the-art accuracy on the well-known image classification datasets.
MoreTranslated text
Key words
Deep learning, Bi-level optimization, Meta Learning, Image classification
AI Read Science
Must-Reading Tree
Example
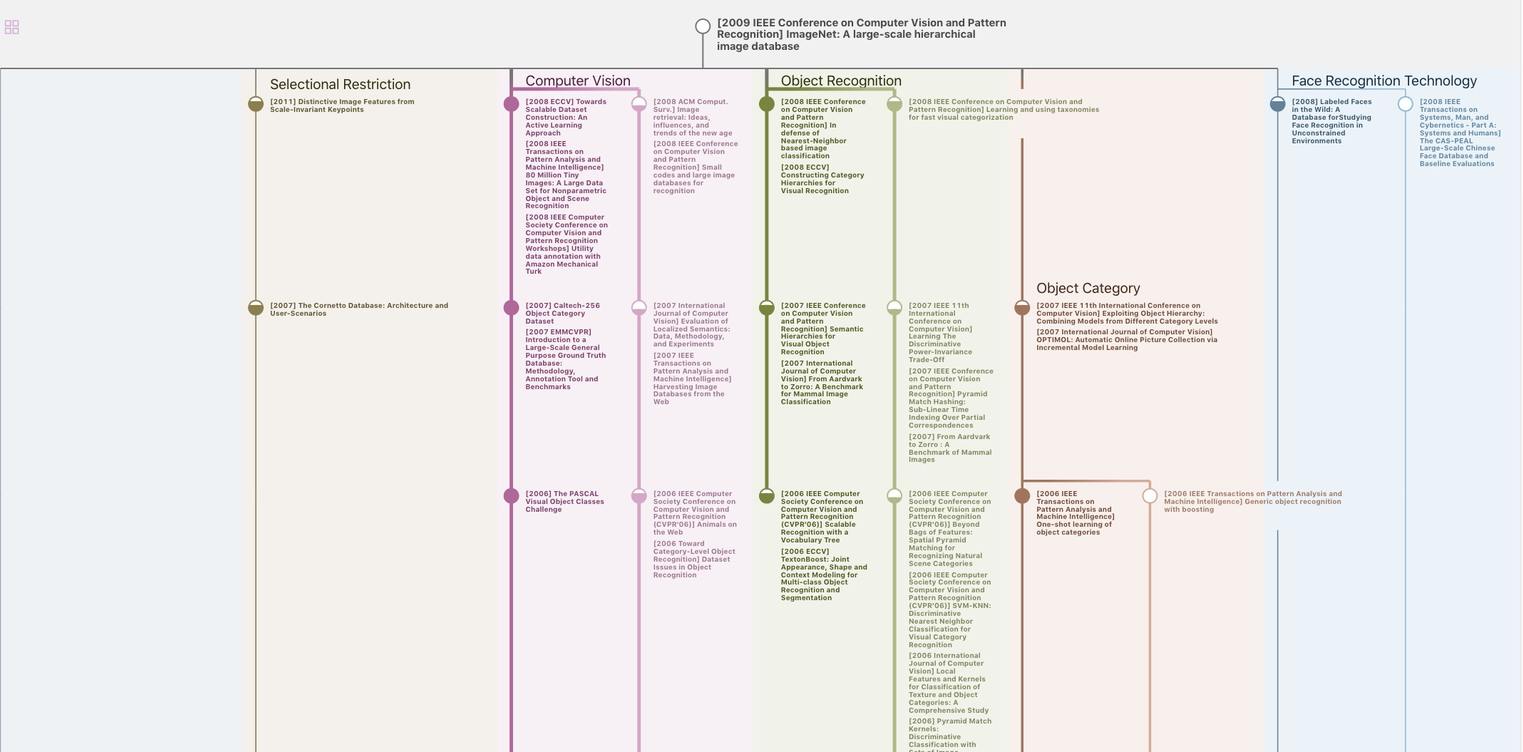
Generate MRT to find the research sequence of this paper
Chat Paper
Summary is being generated by the instructions you defined