Detection of Cache Pollution Attack Based on Federated Learning in Ultra-Dense Network
Computers & Security(2023)
摘要
Ultra-Dense Network (UDN) as one of the key technologies of 5G aims to increase the capacity per area of the current 4G network by 1,000-fold through the deployment of dense Small Base Stations (SBSs). To reduce the traffic on backhaul links, Content-Centric Networking (CCN) as a promising network architecture has been applied to UDN recently. However, its in-network caching mechanism is subject to Cache Pollution Attack (CPA), where attackers aim to occupy limited caching space with unpopular contents. Existing studies focusing on the static networks cannot be directly used in dynamic UDN scenarios. In this paper, we propose a detection and defense scheme of CPA based on federated learning, where CPA is determined by the cooperation of multiple SBSs. First, each SBS can build or join a cluster based on the metrics of distance similarity and load similarity. Then, each cluster head as the working node trains the local classifier to detect CPA based on the statistics within the cluster. The macro base station aggregates all the local models to generate an improved global classifier for all the SBSs. In our scheme, federated learning as a special case of distributed machine learning allows each cluster head to independently train the local data even when it is non-i.i.d. or unbalanced. Simulation results show that our scheme outperforms in terms of detection ratio, cache hit ratio, access delay, and false alarm ratio compared with other state-of-the-art schemes.
更多查看译文
关键词
Cache pollution attack,Federated learning,Content centric network,Ultra-Dense network,5G
AI 理解论文
溯源树
样例
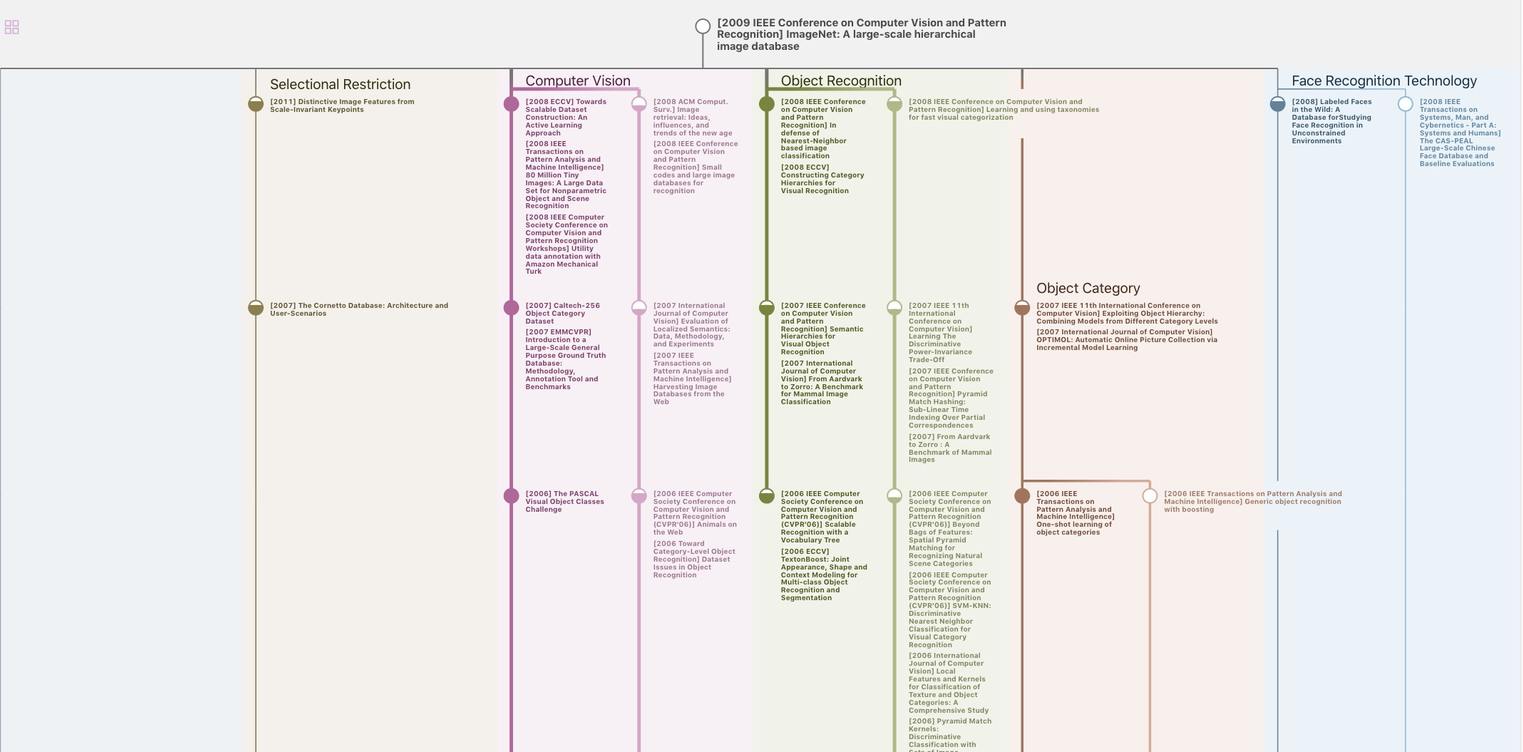
生成溯源树,研究论文发展脉络
Chat Paper
正在生成论文摘要