Virtual prompt pre-training for prototype-based few-shot relation extraction
EXPERT SYSTEMS WITH APPLICATIONS(2023)
摘要
Prompt tuning with pre-trained language models (PLM) has exhibited outstanding performance by reducing the gap between pre-training tasks and various downstream applications, which requires additional labor efforts in label word mappings and prompt template engineering. However, in a label intensive research domain, e.g., few-shot relation extraction (RE), manually defining label word mappings is particularly challenging, because the number of utilized relation label classes with complex relation names can be extremely large. Besides, the manual prompt development in natural language is subjective to individuals. To tackle these issues, we propose a virtual prompt pre-training method, projecting the virtual prompt to latent space, then fusing with PLM parameters. The pre-training is entity-relation-aware for RE, including the tasks of mask entity prediction, entity typing, distant supervised RE, and contrastive prompt pre-training. The proposed pre-training method can provide robust initialization for prompt encoding, while maintaining the interaction with the PLM. Furthermore, the virtual prompt can effectively avoid the labor efforts and the subjectivity issue in label word mapping and prompt template engineering. Our proposed prompt-based prototype network delivers a novel learning paradigm to model entities and relations via the probability distribution and Euclidean distance of the predictions of query instances and prototypes. The results indicate that our model yields an averaged accuracy gain of 4.21% on two few-shot datasets over strong RE baselines. Based on our proposed framework, our pre-trained model outperforms the strongest RE-related PLM by 6.52%.
更多查看译文
关键词
Few-shot learning, Information extraction, Prompt tuning, Pre-trained Language Model
AI 理解论文
溯源树
样例
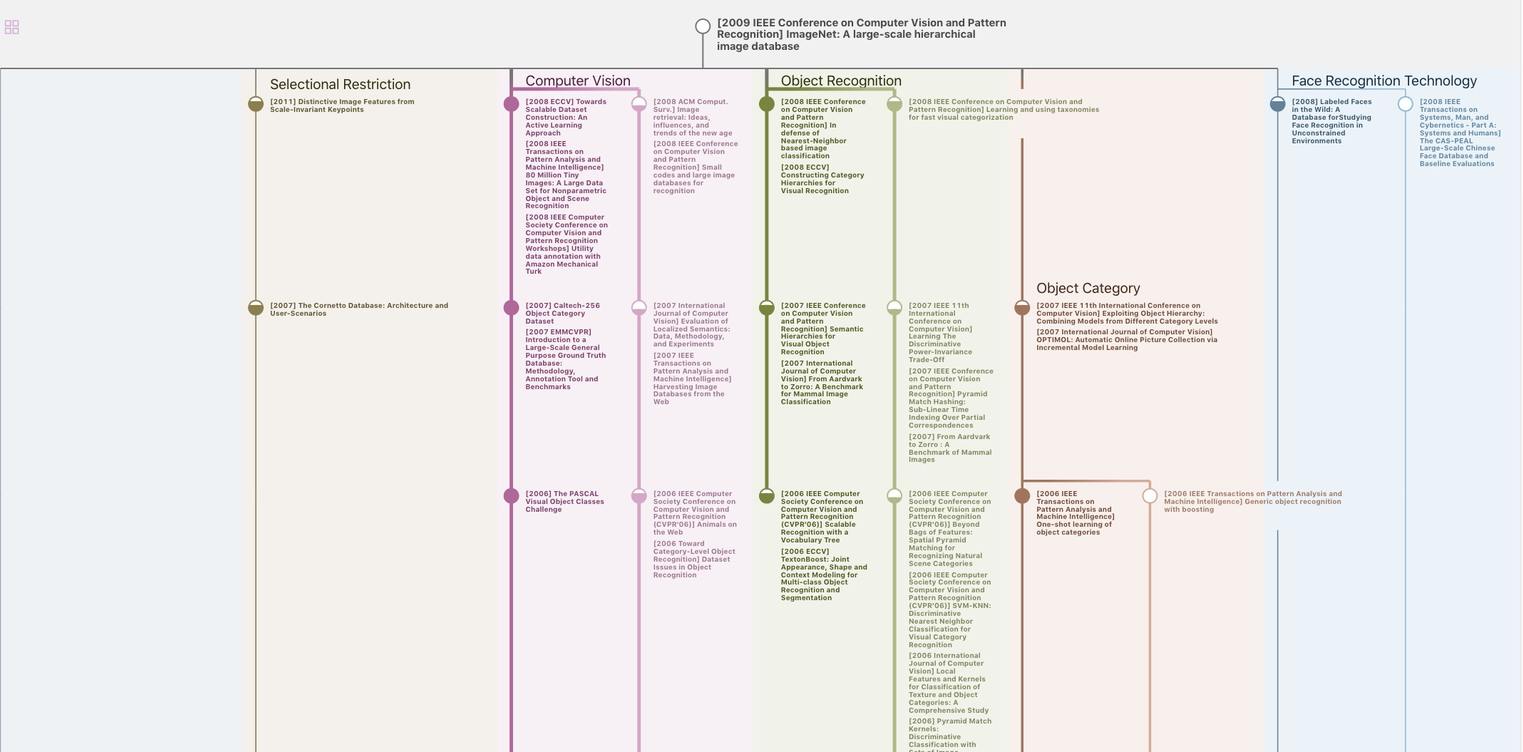
生成溯源树,研究论文发展脉络
Chat Paper
正在生成论文摘要