Comparison of inverse uncertainty quantification methods for critical flow test
ENERGY(2023)
Abstract
A problem of epistemic uncertainties introduced by code input parameters that cannot be estimated other than by user expertise has existed in nuclear reactor systems analyses since first uncertainty analyses were conducted. Inverse Uncertainty Quantification (IUQ) methods aim at providing an estimation of the distributions of such parameters. This paper compares and assesses two approaches to quantify the uncertainty of critical flow model parameters available in the TRACE code. First novel approach is based on a machine-learning algorithm, using a Random Forest classifier to assign the results of calculations to one of the defined classes of prediction accuracy with respect to experimental data. The second approach is based on Markov Chain Monte Carlo sampling and Bayesian inference. Both methods allows to assign probability distribution functions to TRACE internal variables, which is a goal for IUQ methods. Both approaches were used to estimate uncertainties for four critical flow parameters available in the TRACE code based on the Marviken critical flow experiment. In case of two parameters both methods produced similar results of the probability density functions. Based on the results of machine learning two additional parameters were deemed noninfluential, while for the second method such definitive assessment could not be made.
MoreTranslated text
Key words
Critical flow,TRACE,Machine learning,Inverse uncertainty quantification,Markov chain Monte Carlo
AI Read Science
Must-Reading Tree
Example
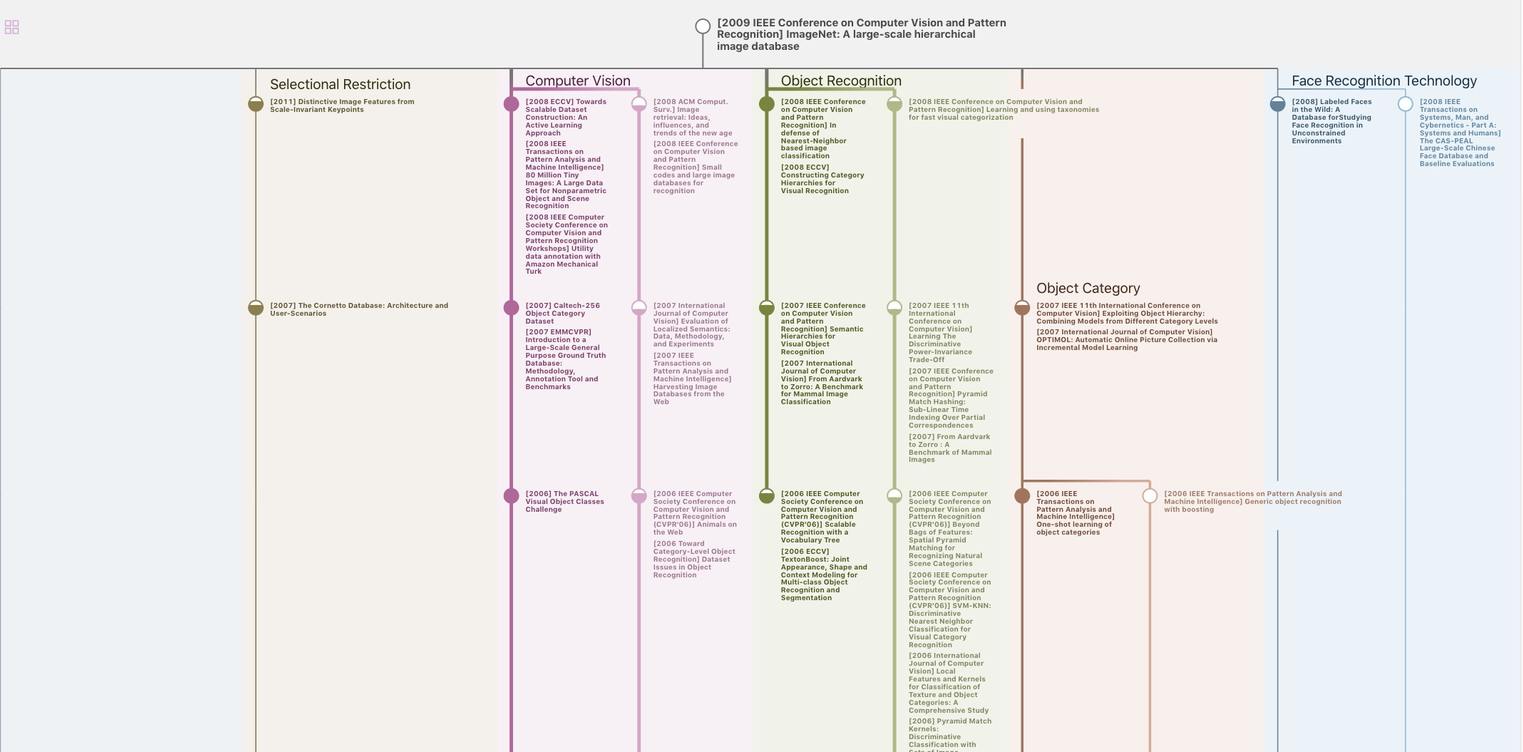
Generate MRT to find the research sequence of this paper
Chat Paper
Summary is being generated by the instructions you defined