Spatial Foreground Bigraph Matching for Generalizable Person Re-identification
ARTIFICIAL NEURAL NETWORKS AND MACHINE LEARNING - ICANN 2022, PT III(2022)
Abstract
Most existing Domain Generalization person re-identification (DG ReID) methods often evaluate similarity using global features. However, the spatial information will be lost after global pooling, which leads to a lack of interpretability and decreased generalization ability. In this paper, to address these issues, we propose a novel interpretable and generalizable ReID framework, named Spatial Foreground Bigraph Match (SFBM), which is plug-and-play and no training is required. Our SFBM consists of two sub-parts, namely Contextual Foreground Region Extraction (CFE) and Feature Bigraph Module (FBM). The former extracts the foreground in the query's feature map and uses it to filter out the background noise in the matching relationship. The latter then establishes a matching relationship between each local feature in the feature map of query and gallery. Particularly, we present a judicious definition of the distance from query to gallery based on SFBM to enhance the robustness of existing models when the target domain has an occlusion. Extensive experimental results on three subtasks of DG ReID show that our method is model-agnostic and enables to improve the performance of various methods without re-training the models.
MoreTranslated text
Key words
Domain generalization,Person re-identification,Spatial feature
AI Read Science
Must-Reading Tree
Example
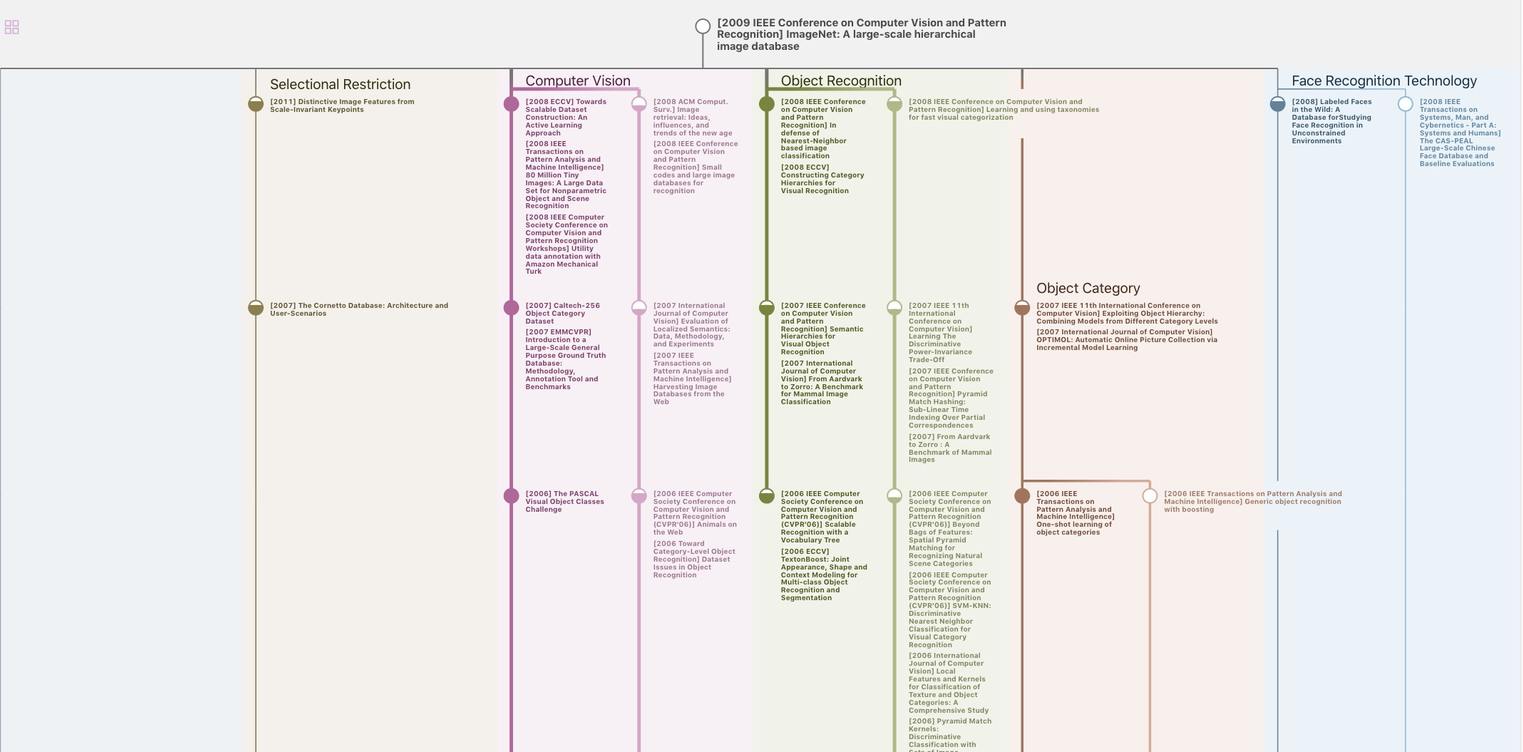
Generate MRT to find the research sequence of this paper
Chat Paper
Summary is being generated by the instructions you defined