Category-Guided Localization Network for Visual Sound Source Separation.
ARTIFICIAL NEURAL NETWORKS AND MACHINE LEARNING - ICANN 2022, PT III(2022)
摘要
Learning audiovisual correspondence plays an important role in understanding outside world. The object of this work is to clearly separate the audio corresponding to the given visual object from mixed audio, i.e., visually guided sound source separation. However, accurately obtaining and matching reference features in visual and audio modalities, which is crucial in separation tasks, still remains a tough challenge. In this paper, we propose a novel three-modality audio-visual separation framework, Category-Guided Localization Network (CGLN), to address this problem. The proposed category-guided approach can cover the sound object more precisely than others to obtain better separation. Object and motion features are used as guiding signals to realize sound source separation in an end-to-end manner Extensive experiments are performed on two publicly-available datasets, MUSIC and AudioSet. Our method has achieved the goal of modality matching and alignment, and the results could outperform other state-of-the-art approaches.
更多查看译文
关键词
Audio-visual,Sound separation,Sound localization
AI 理解论文
溯源树
样例
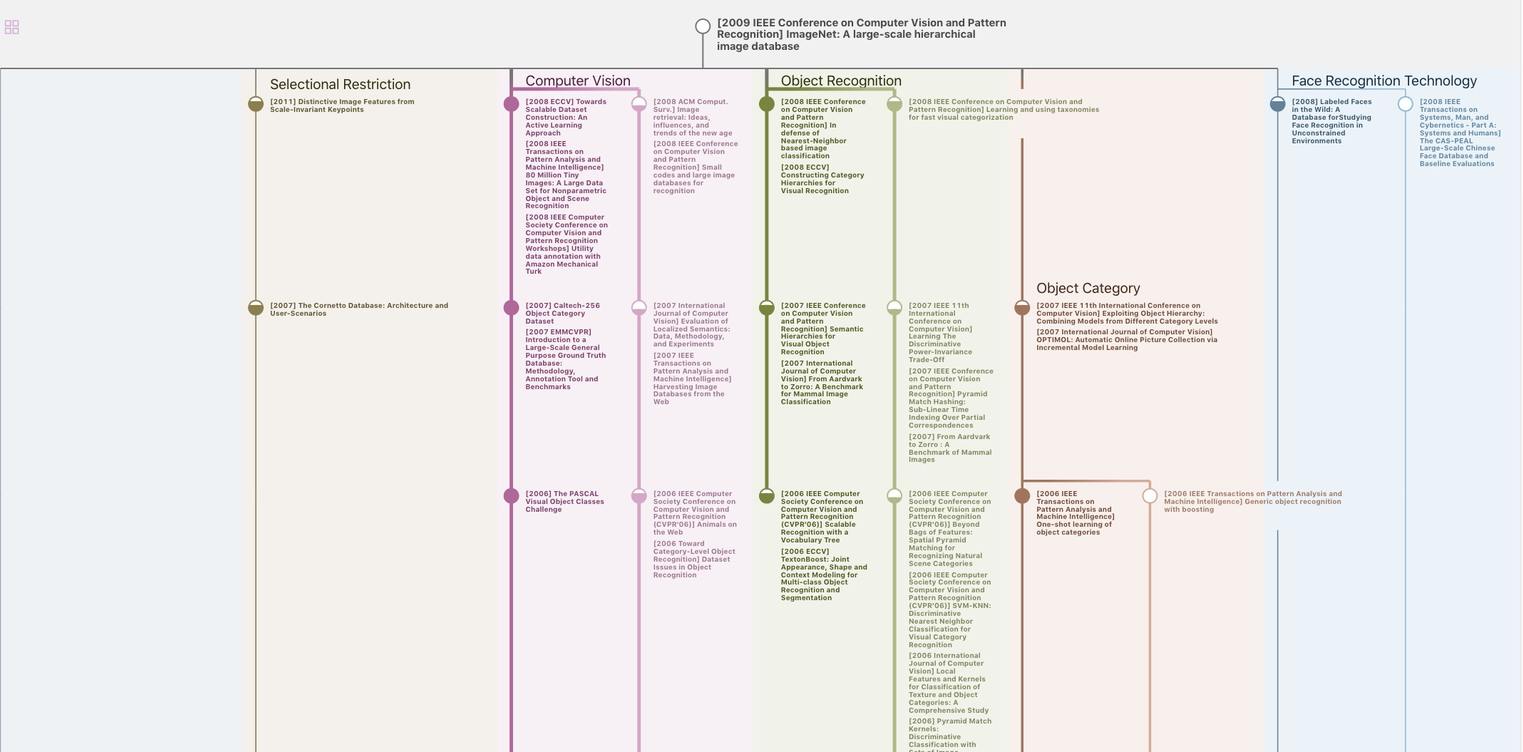
生成溯源树,研究论文发展脉络
Chat Paper
正在生成论文摘要