Hierarchical Deep Multi-task Learning for Classification of Patient Diagnoses
ARTIFICIAL INTELLIGENCE IN MEDICINE, AIME 2022(2022)
摘要
Recent years have witnessed an increased interest in the biomedical research community in developing machine learning models and methods that can automatically assign diagnostic codes (ICD) to patient stays based on the information in their Electronic Health Records (EHR). However, despite the recent advances, accurate automatic classification of diagnostic codes continues to face challenges, especially for low-prior diagnostic codes. To alleviate the problem, we propose to leverage information in the diagnostic hierarchy and better utilize the dependencies among diseases in this hierarchy. We develop a new hierarchical deep multi-task learning method that learns classification models for multiple diagnostic codes at the different levels of abstraction in the disease hierarchy while allowing the transfer of information from high-level nodes, more general diagnoses codes to the low-level ones, more specific diagnostic codes. After that, we refine the initial hierarchical model by utilizing the relations and information that can discriminate better between competing diseases. Our empirical results show that our new method and its refinement outperform baseline machine learning architectures that do not leverage the hierarchical structure of target diagnoses tasks or disease-disease relationships.
更多查看译文
关键词
Hierarchical multi-task learning, Patient diagnoses classification, International classification diseases
AI 理解论文
溯源树
样例
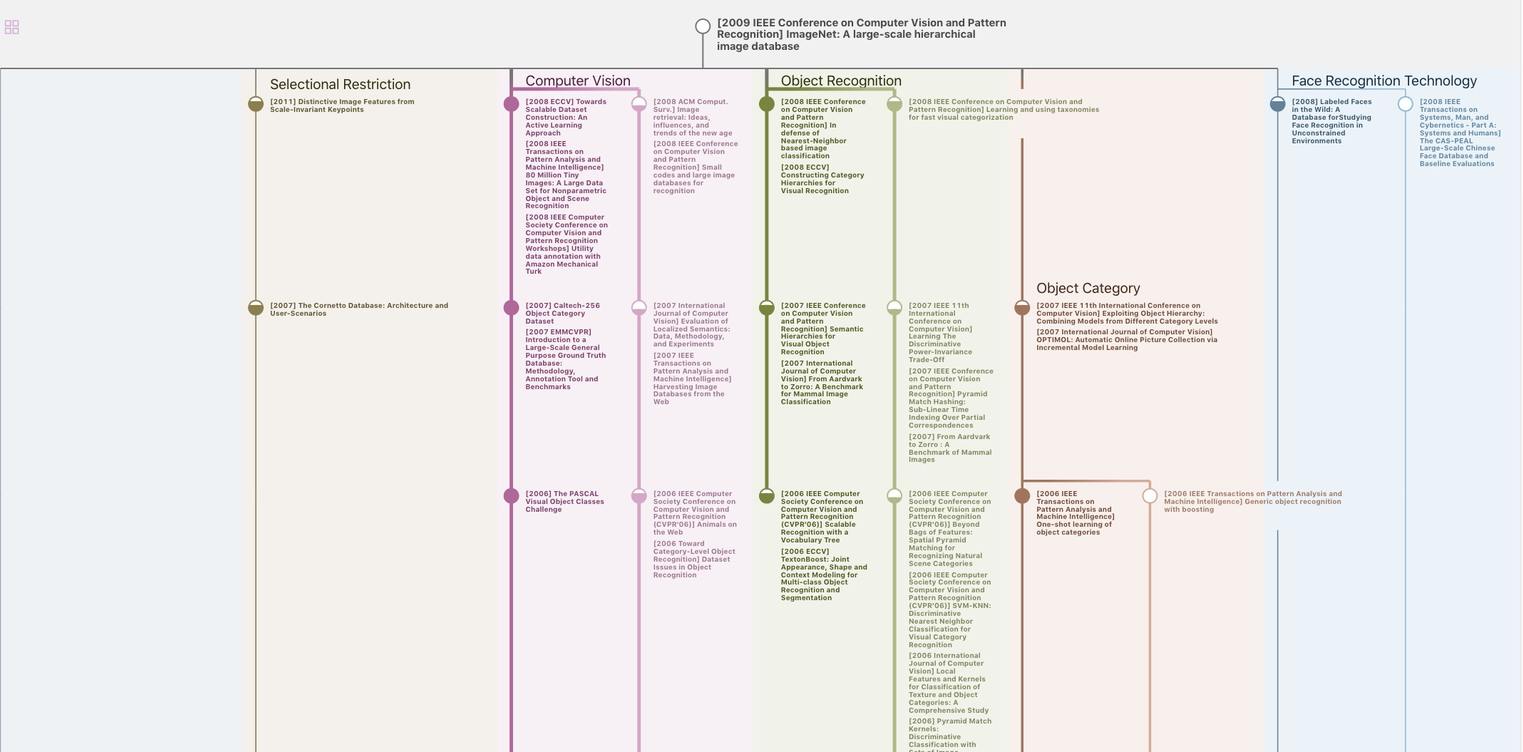
生成溯源树,研究论文发展脉络
Chat Paper
正在生成论文摘要