Learning-based State-dependent Coefficient Form Task Space Tracking Control of Soft Robot
2022 AMERICAN CONTROL CONFERENCE (ACC)(2022)
摘要
In this paper, a data-driven modeling and control framework is developed for task space control of a soft robot gripper which consists of four individual soft lingers. Each of the four fingers is modeled as a manipulator with high degrees of freedom. The corresponding task space dynamics of the manipulator are derived using a rigid-link approximation of the continuum manipulator. A neural network approach is used to learn the derived dynamics in State Dependent Coefficient (SDC) form. Using the learned SDC matrices, an asymptotically stable optimal closed-loop tracking controller which is based on solving the State Dependent Riccati Equation (SDRE) is derived. The model learning and trajectory tracking controller is implemented on an open source Soft Motion (SoMo) platform simulating the soft gripper motion and corresponding tracking results are presented.
更多查看译文
关键词
robot,learning-based,state-dependent
AI 理解论文
溯源树
样例
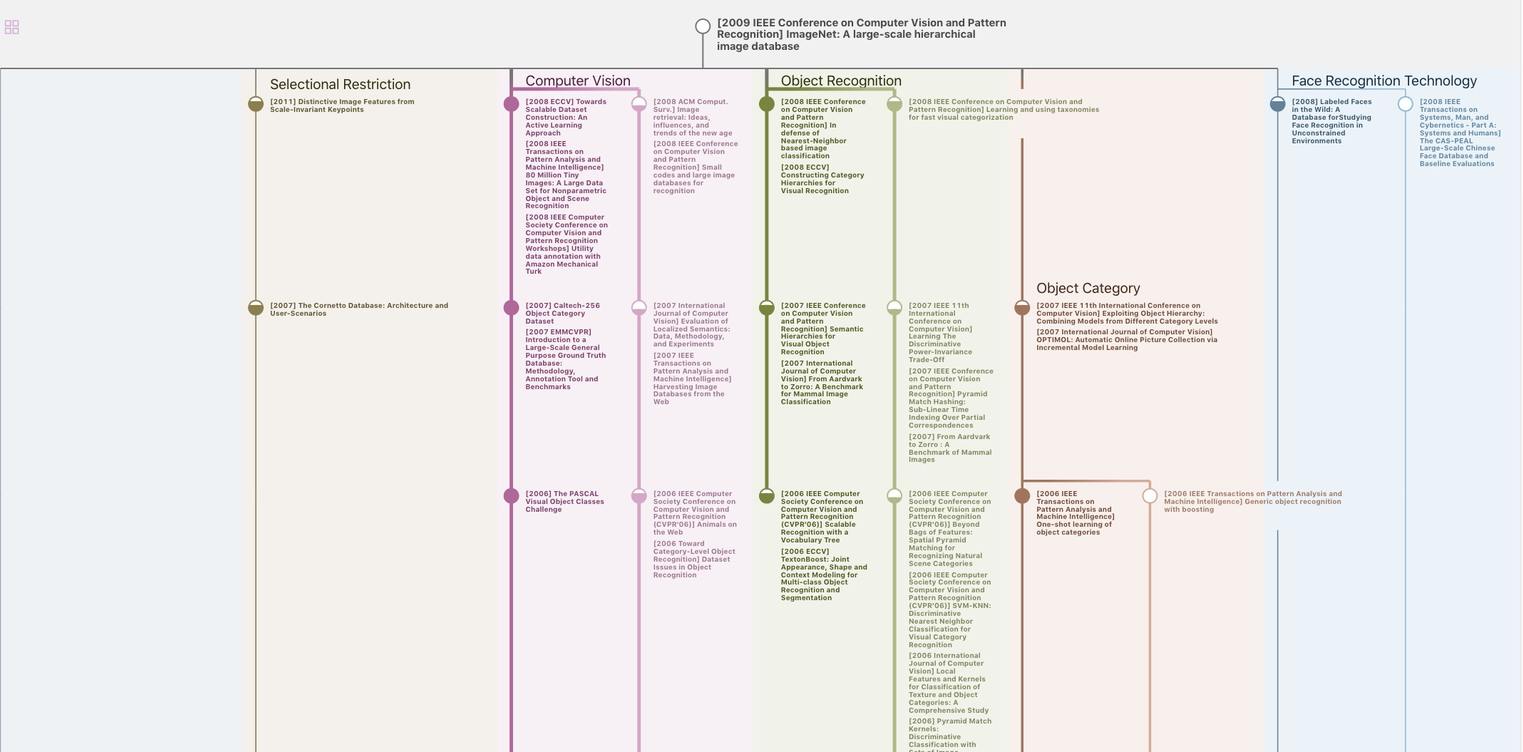
生成溯源树,研究论文发展脉络
Chat Paper
正在生成论文摘要