Total Deep Variation for Linear Inverse Problems
CVPR(2020)
摘要
Diverse inverse problems in imaging can be cast as variational problems composed of a task-specific data fidelity term and a regularization term. In this paper, we propose a novel learnable general-purpose regularizer exploiting recent architectural design patterns from deep learning. We cast the learning problem as a discrete sampled optimal control problem, for which we derive the adjoint state equations and an optimality condition. By exploiting the variational structure of our approach, we perform a sensitivity analysis with respect to the learned parameters obtained from different training datasets. Moreover, we carry out a nonlinear eigenmode analysis, which reveals interesting properties of the learned regularizer. We show state-of-the-art performance for classical image restoration and medical image reconstruction problems.
更多查看译文
关键词
learnable general-purpose regularizer,nonlinear eigenfunction analysis,training datasets,learned parameters,sensitivity analysis,variational structure,optimality condition,adjoint state equations,discrete sampled optimal control problem,deep learning,architectural design patterns,regularization term,task-specific data fidelity term,variational problems,diverse inverse problems,linear inverse problems,total deep variation,medical image reconstruction problems,classical image restoration,learned regularizer
AI 理解论文
溯源树
样例
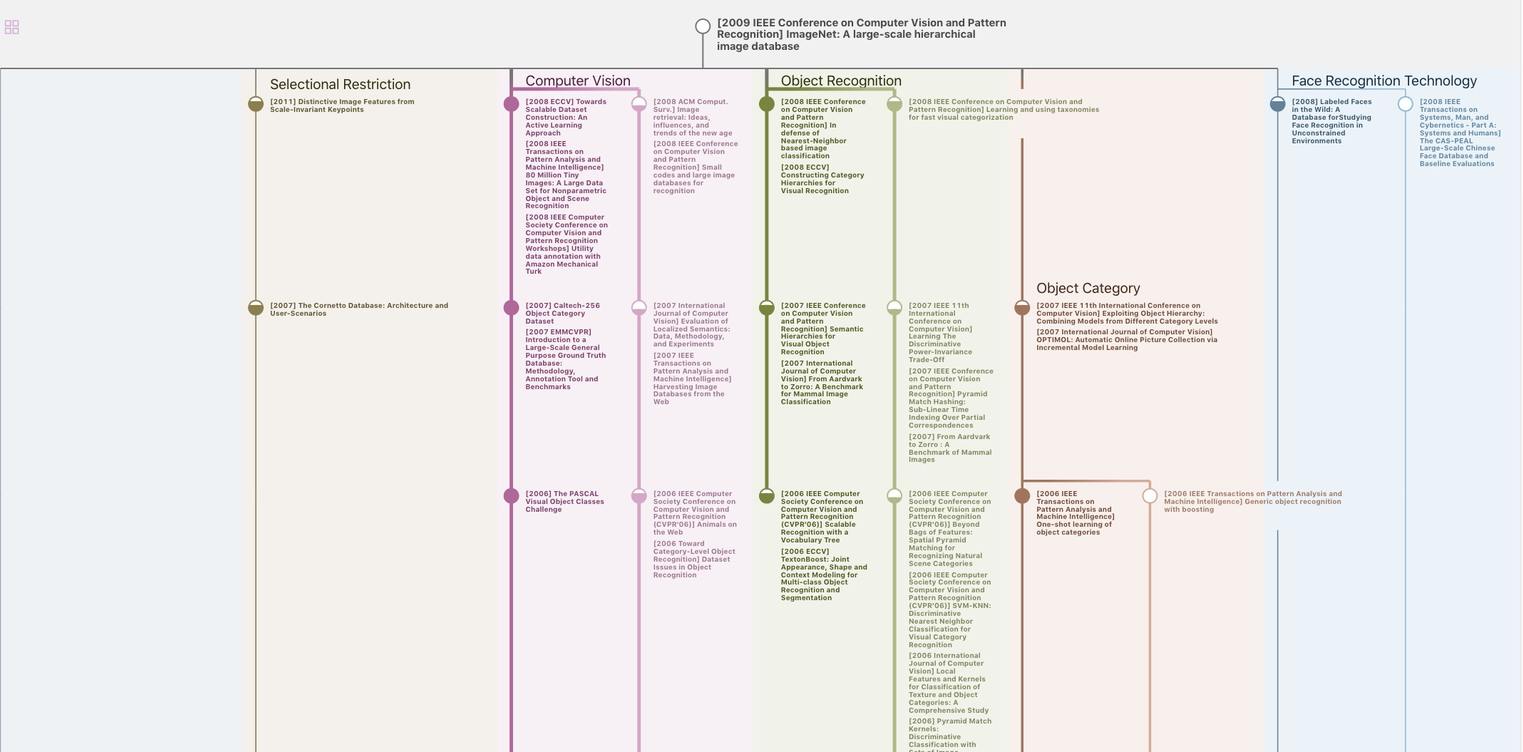
生成溯源树,研究论文发展脉络
Chat Paper
正在生成论文摘要