Task Accuracy Enhancement for a Surgical Macro-Micro Manipulator With Probabilistic Neural Networks and Uncertainty Minimization
IEEE TRANSACTIONS ON AUTOMATION SCIENCE AND ENGINEERING(2024)
摘要
Accurate robot kinematic modelling is a major component for autonomous robot control to guarantee safety and precision during task execution. In surgical robotics complex robotic structures and actuation mechanisms are generally employed, therefore machine learning techniques can be adopted to build the model of the robot. Probabilistic neural networks are a class of learning approaches that provide information about the uncertainty of the learnt models. In this work we compare two different probabilistic neural networks (Bayesian and Evidential Neural Networks) to model the kinematics of a surgical robotic instrument and propose a control strategy based on Hierarchical Quadratic Programming (HQP) capable of exploiting the model uncertainty to improve the accuracy and safety of the controller. Simulation and real world experiments on different autonomous path tracking tasks show that the model uncertainty highly affects the control performances and prove the effectiveness of the proposed controller in improving task execution.
更多查看译文
关键词
Probabilistic neural networks,redundant robots,optimal control,model uncertainty,minimally invasive robotic surgery
AI 理解论文
溯源树
样例
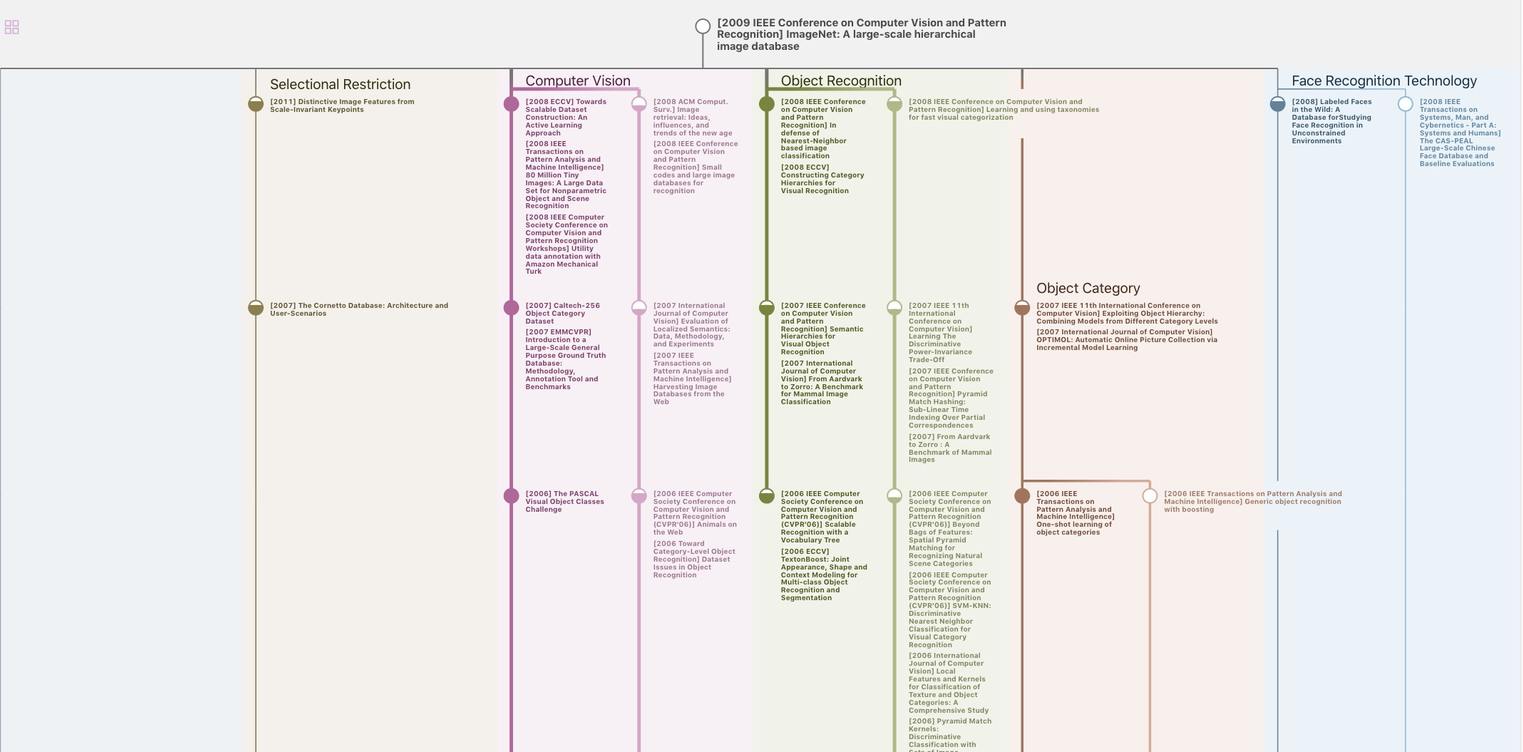
生成溯源树,研究论文发展脉络
Chat Paper
正在生成论文摘要