Full-scale spatio-temporal traffic flow estimation for city-wide networks: a transfer learning based approach
TRANSPORTMETRICA B-TRANSPORT DYNAMICS(2023)
摘要
The full-scale spatio-temporal traffic flow estimation/prediction has always been a hot spot in transportation engineering. The low coverage rate of detectors in transport networks brings difficulties to the city-wide traffic flow estimation/prediction. Moreover, it is difficult for traditional analytical traffic flow models to deal with the traffic flow estimation/prediction problem over urban transport networks in a complex environment. Current data-driven methods mainly focus on road segments with detectors. An instance-based transfer learning method is proposed to estimate network-wide traffic flows including road segments without detectors. Case studies based on simulation data and empirical data collected from the open-source PeMS database are conducted to verify its effectiveness. For the traffic flow estimation of segments without detectors, the mean absolute percentage error (MAPE) is approximately 11% for both datasets, which is superior to the existing methods in the literature and reduces MAPE by two percentage points.
更多查看译文
关键词
Transport network flow estimation,Gaussian process,clustering ensemble algorithm,transfer learning method,link relevance
AI 理解论文
溯源树
样例
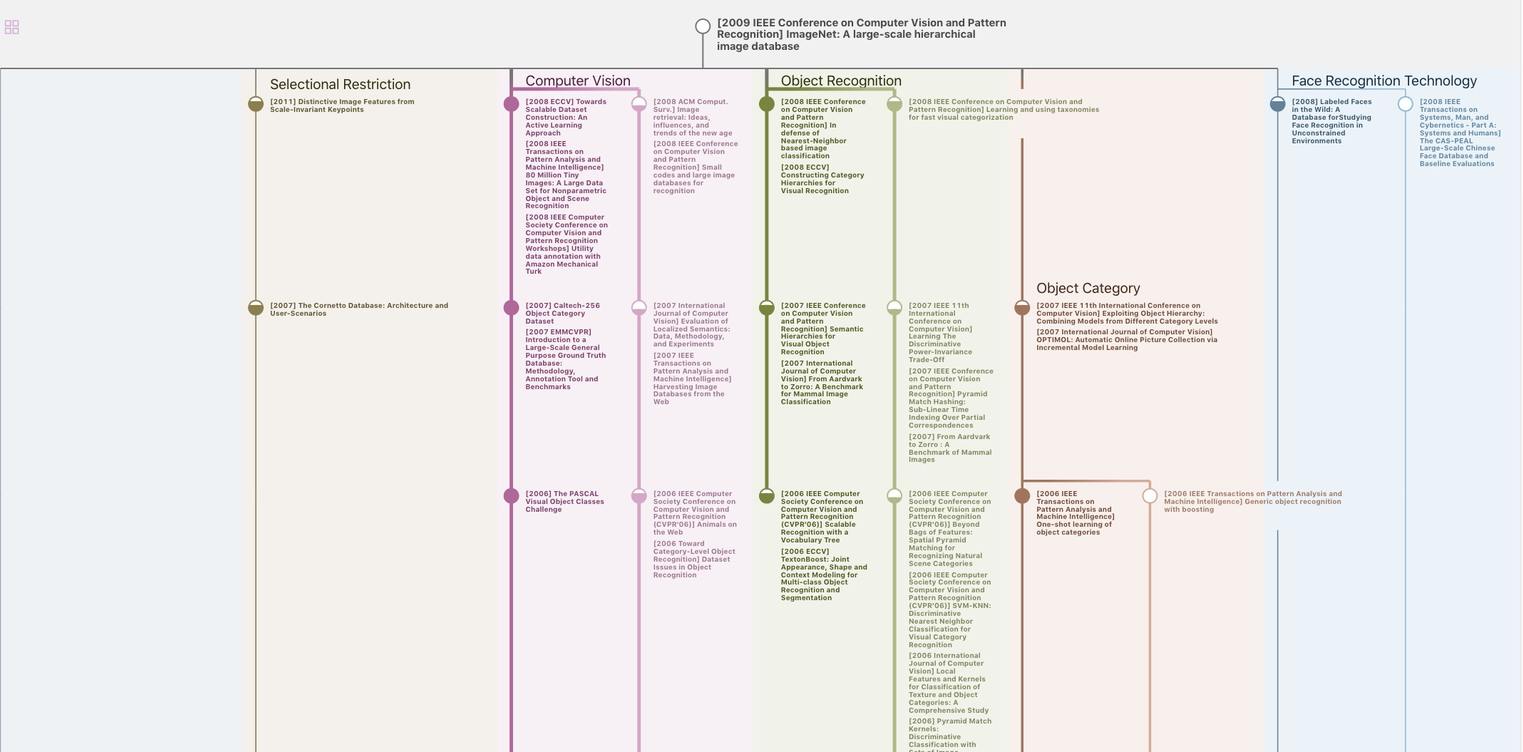
生成溯源树,研究论文发展脉络
Chat Paper
正在生成论文摘要