A network-constrained clustering method for bivariate origin-destination movement data
INTERNATIONAL JOURNAL OF GEOGRAPHICAL INFORMATION SCIENCE(2023)
Abstract
For bivariate origin-destination (OD) movement data composed of two types of individual OD movements, a bivariate cluster can be defined as a group of two types of OD movements, at least one of which has a high density. The identification of such bivariate clusters can provide new insights into the spatial interactions between different movement patterns. Because of spatial heterogeneity, the effective detection of inhomogeneous and irregularly shaped bivariate clusters from bivariate OD movement data remains a challenge. To fill this gap, we propose a network-constrained method for clustering two types of individual OD movements on road networks. To adaptively estimate the densities of inhomogeneous OD movements, we first define a new network-constrained density based on the concept of the shared nearest neighbor. A fast Monte Carlo simulation method is then developed to statistically estimate the density threshold for each type of OD movements. Finally, bivariate clusters are constructed using the density-connectivity mechanism. Experiments on simulated datasets demonstrate that the proposed method outperformed three state-of-the-art methods in identifying inhomogeneous and irregularly shaped bivariate clusters. The proposed method was applied to taxi and ride-hailing service datasets in Xiamen. The identified bivariate clusters successfully reveal competition patterns between taxi and ride-hailing services.
MoreTranslated text
Key words
Origin-destination movement data,bivariate clustering,road network,spatial heterogeneity
AI Read Science
Must-Reading Tree
Example
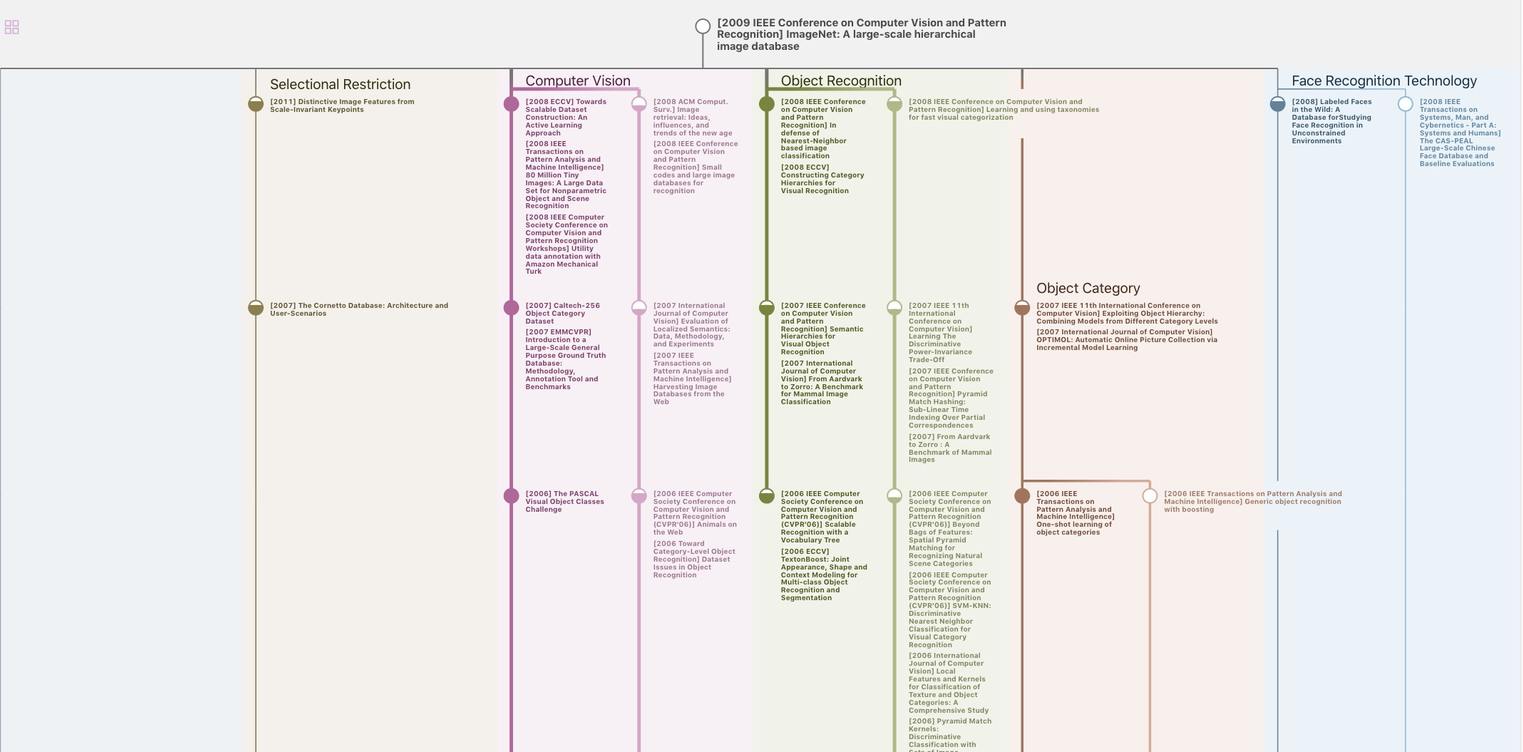
Generate MRT to find the research sequence of this paper
Chat Paper
Summary is being generated by the instructions you defined